SAR Target Open-Set Recognition Based on Joint Training of Class-Specific Sub-Dictionary Learning
IEEE GEOSCIENCE AND REMOTE SENSING LETTERS(2024)
摘要
Synthetic aperture radar (SAR) automatic target recognition (ATR) has attracted extensive attention and achieved satisfactory results. However, most SAR ATR methods follow the closed-set assumption, which assumes that all target classes in the test set have been contained by the training set. In actual scenarios, it may encounter the target classes that are not included in the training set, and it presents a challenge for SAR ATR. To tackle this issue, this letter proposes an open-set recognition method based on joint training of class-specific sub-dictionary learning. First, joint training is used to optimize the sub-dictionary learning process, and it could significantly enhance the discriminative ability of these sub-dictionaries. Second, the reconstruction errors of the targets on each sub-dictionary are calculated. These errors can be split into matched and no-matched errors. Third, extreme value theory (EVT) is employed to model the matched and no-matched errors of each class, which could determine the class boundaries. Finally, for the target to be recognized, its reconstruction errors on each sub-dictionary are calculated individually. The class of this target can be determined by comparing the errors with these class boundaries. Our method achieved an accuracy of 87.22-94.02 and an F1 score of 88.03-90.25 in multiple experiments on moving and stationary target automatic recognition (MSTAR) dataset. Compared with several state-of-the-art methods, it has better accuracy and robustness.
更多查看译文
关键词
Extreme value theory (EVT),open-set recognition,sub-dictionary learning,synthetic aperture radar (SAR)
AI 理解论文
溯源树
样例
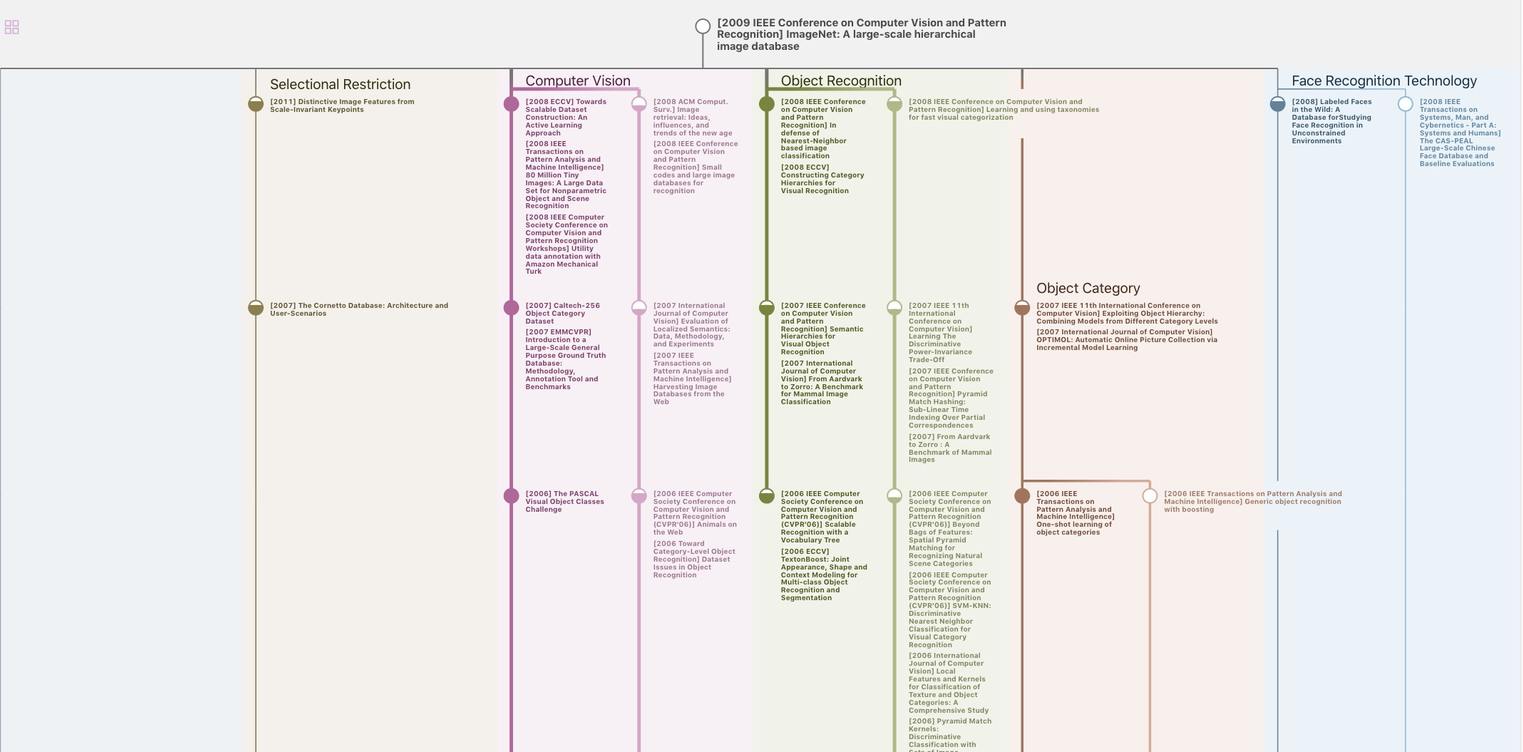
生成溯源树,研究论文发展脉络
Chat Paper
正在生成论文摘要