Investigating the Effectiveness of Whitening Post-processing Methods on Modifying LLMs Representations
2023 IEEE 35TH INTERNATIONAL CONFERENCE ON TOOLS WITH ARTIFICIAL INTELLIGENCE, ICTAI(2023)
摘要
In contemporary natural language processing(NLP) tasks, it is common to utilize the representation of large language models(LLMs) directly in downstream applications. However, this approach is restricted to anisotropy due to the convex cone representation space of LLMs, which hinders performance evaluations across various NLP tasks. In light of this limitation, we investigate several whitening post-processing methods to modify the LLMs representation space, including PCA, ZCA, PCA-cor, ZCA-cor and Cholesky whitening methods, aiming to transform textual representation space into a decorrelated orthogonal basis space. Comprehensive experiments are conducted over 20 datasets encompassing diverse NLP tasks to evaluate the effectiveness of these methods based on models such as Bert, GPT2, and others. Our results indicate that ZCA-cor whitening is appropriate for supervised text classification, while PCA-cor whitening, after reducing dimensionality, is suitable for unsupervised semantic textual similarity. Furthermore, we perform analysis of the relationship between task evaluations and isotropy scores, indicating that whitening methods is capable of mitigating anisotropy.
更多查看译文
关键词
Whitening Transformation,Decorrelation,Text Representation,PCA-cor,ZCA-cor
AI 理解论文
溯源树
样例
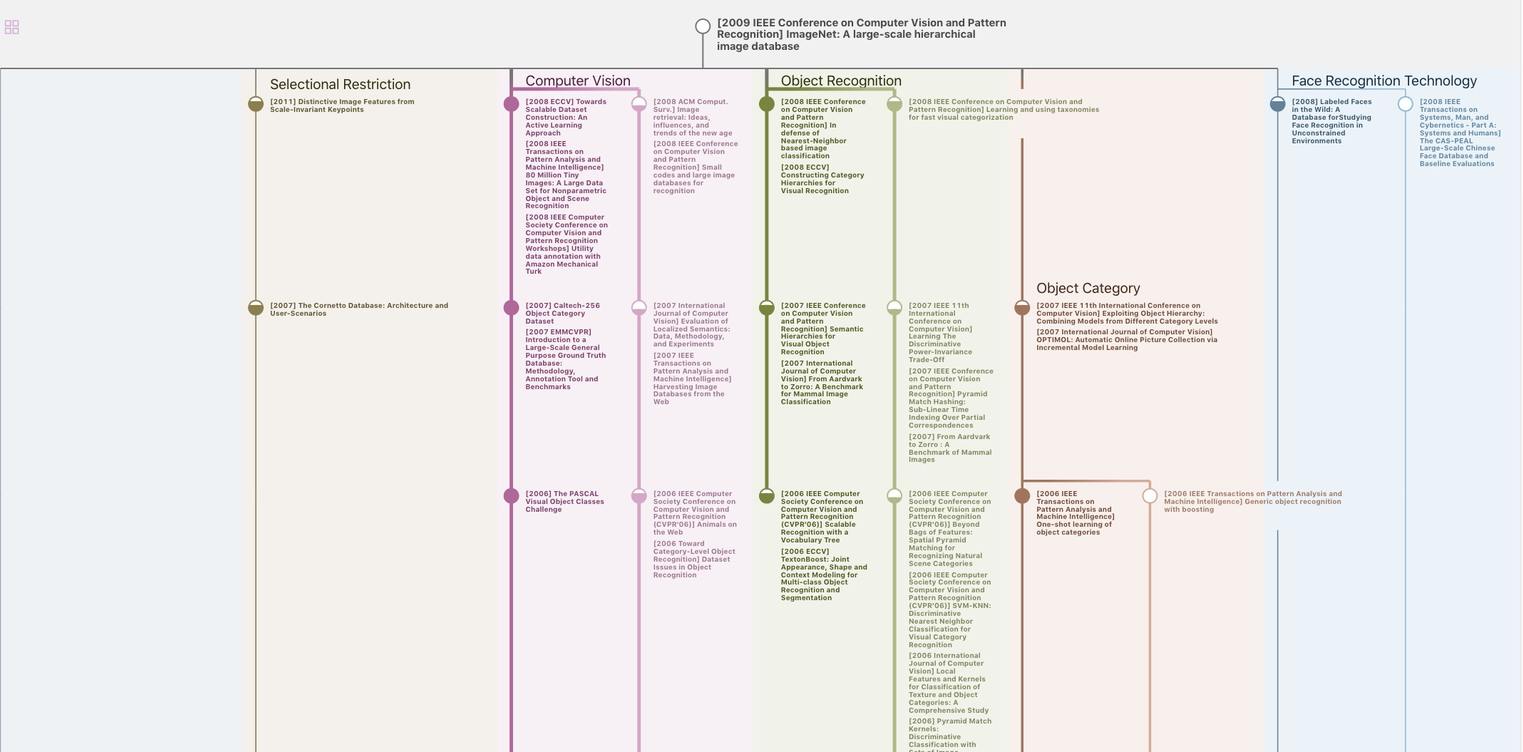
生成溯源树,研究论文发展脉络
Chat Paper
正在生成论文摘要