Human Activity Recognition in Free Living Using a Wearable Sensor: A Two-Stage Approach.
2023 12th International Conference on Awareness Science and Technology (iCAST)(2023)
摘要
A novel two-stage approach for human activity recognition (HAR) using a wearable sensor is proposed in this study. The goal is to achieve both coarse-level and fine-level recognition of activities. The study focuses on five common activities: desk-working, driving, walking, downstairs, and upstairs. Three-axis acceleration signals were collected using a wrist-worn accelerometer sensor, and the activities were annotated during the participant’s daily routines. The acceleration data were preprocessed through segmentation, feature extraction, and normalization. In the first stage, a traditional machine learning model (decision tree) was used for coarse-level classification, distinguishing between activities with exercise and without exercise. In the second stage, deep learning models were employed for fine-level classification to recognize specific activities within the coarse classes. The performance of the models was evaluated using accuracy, sensitivity, and specificity. The proposed approach showed promising results with accuracies of 93.53%, 93.52%, 85.70%, 98.11%, and 85.91% respectively for desk-working, driving, walking, downstairs, and upstairs, demonstrating the effectiveness of the two-stage strategy for HAR. The proposed two-stage approach outperforms the one-stage method (decision tree classifier and deep learning model), particularly in imbalanced data, achieving a graceful equilibrium between sensitivity and specificity. The study highlights the importance of considering the complexity and variability of daily activities for accurate classification and resource optimization.
更多查看译文
关键词
coarse-fine classification,deep learning,human activity recognition,machine learning,wearable sensor
AI 理解论文
溯源树
样例
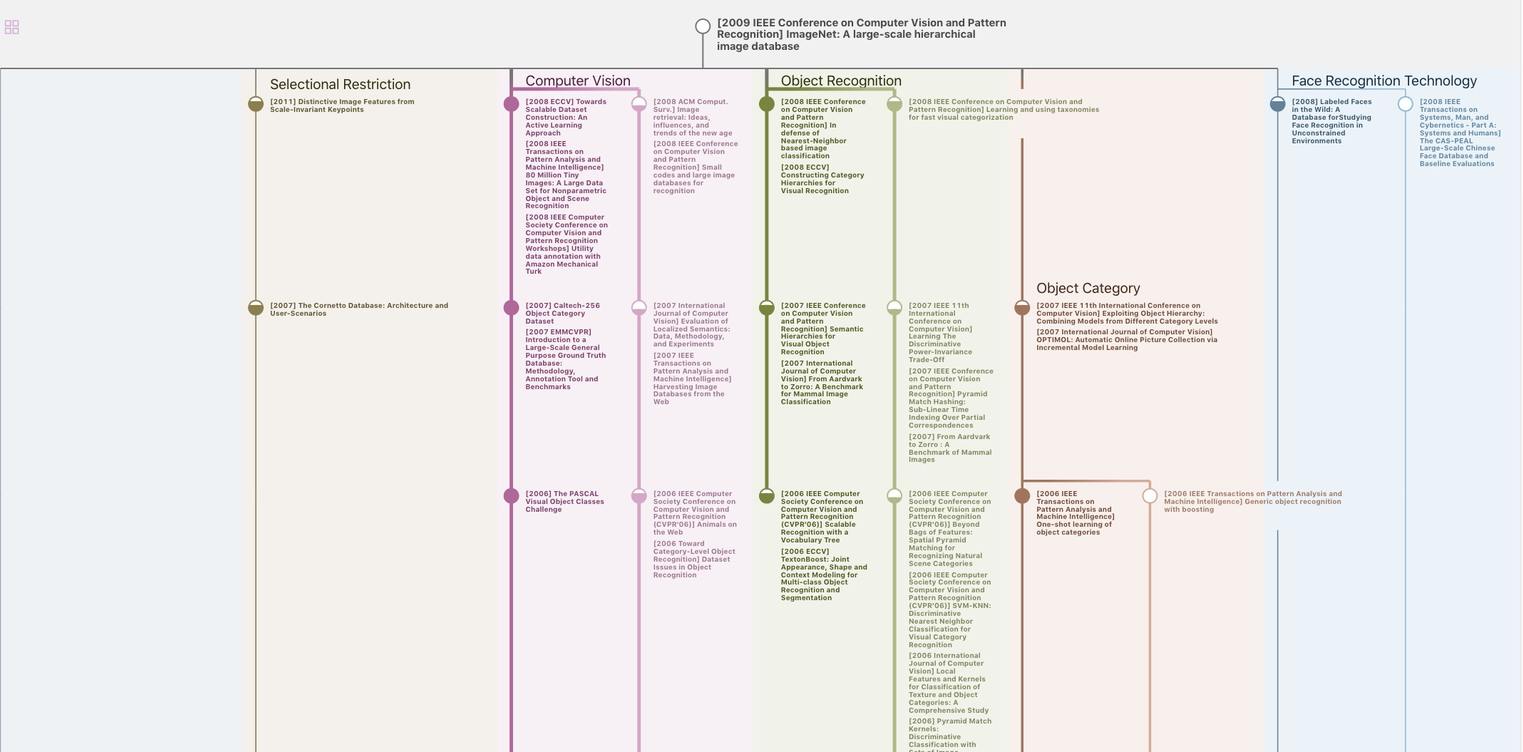
生成溯源树,研究论文发展脉络
Chat Paper
正在生成论文摘要