Multi-Agent Reinforcement Learning for a Multichannel Uplink Random Access: Congestion Game Perspective.
2023 12th International Conference on Awareness Science and Technology (iCAST)(2023)
摘要
In this paper, we propose a time-slotted multichannel uplink random access (RA) game model where players do not cooperate. We first analyze its sum throughput from the congestion game (CG) perspective and obtain the pure strategy Nash equilibria (PNEs) that fully utilize each slot. Then, we propose an Upper Confidence Bound (UCB)-based multi-agent reinforcement learning (MARL) algorithm to realize the PNEs, where UCB is one of the multi-armed bandit algorithms that work by assigning a confidence level for each action. Finally, via simulation, we show that our proposed algorithm can obtain near-optimal average sum throughput in the long run.
更多查看译文
关键词
Multi-armed bandit,random access,reinforcement learning,congestion game,nash equilibrium
AI 理解论文
溯源树
样例
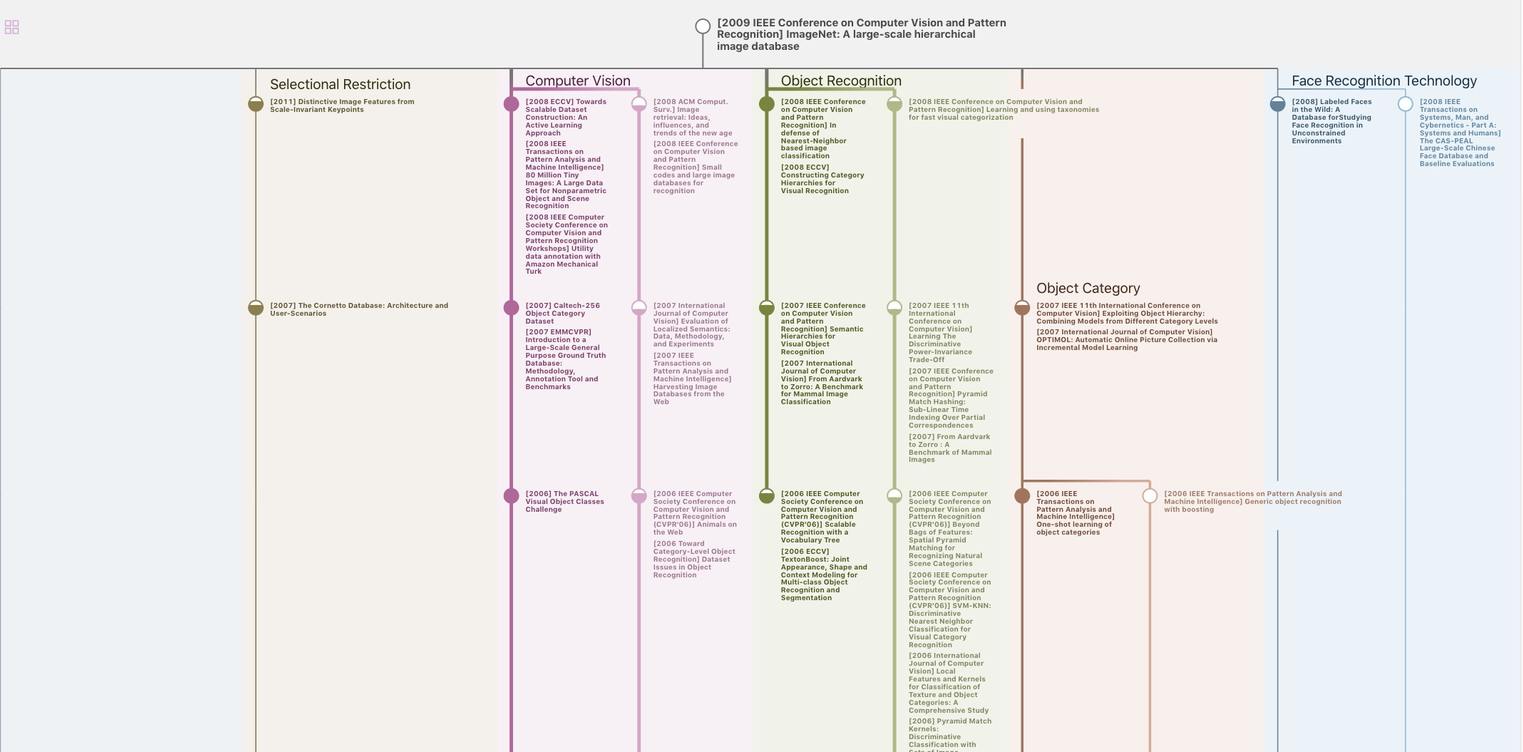
生成溯源树,研究论文发展脉络
Chat Paper
正在生成论文摘要