Real-Time Lightweight Convolutional Neural Network for Polyp Detection in Endoscope Images
Journal of Shanghai Jiaotong University (Science)(2023)
摘要
Colorectal cancer is the most common cancer with a second mortality rate. Polyp lesion is a precursor symptom of colorectal cancer. Detection and removal of polyps can effectively reduce the mortality of patients in the early period. However, mass images will be generated during an endoscopy, which will greatly increase the workload of doctors, and long-term mechanical screening of endoscopy images will also lead to a high misdiagnosis rate. Aiming at the problem that computer-aided diagnosis models deeply depend on the computational power in the polyp detection task, we propose a lightweight model, coordinate attention-YOLOv5-Lite-Prune, based on the YOLOv5 algorithm, which is different from state-of-the-art methods proposed by the existing research that applied object detection models or their variants directly to prediction task without any lightweight processing, such as faster region-based convolutional neural networks, YOLOv3, YOLOv4, and single shot multibox detector. The innovations of our model are as follows: First, the lightweight EfficientNetLite network is introduced as the new feature extraction network. Second, the depthwise separable convolution and its improved modules with different attention mechanisms are used to replace the standard convolution in the detection head structure. Then, the α -intersection over union loss function is applied to improve the precision and convergence speed of the model. Finally, the model size is compressed with a pruning algorithm. Our model effectively reduces parameter amount and computational complexity without significant accuracy loss. Therefore, the model can be successfully deployed on the embedded deep learning platform, and detect polyps with a speed above 30 frames per second, which means the model gets rid of the limitation that deep learning models must rely on high-performance servers.
更多查看译文
关键词
YOLOv5, polyp lesions, object detection, lightweight, weight pruning, YOLOv5, 息肉病变, 目标检测, 轻量化, 权值剪枝, TP 391, R 735, A
AI 理解论文
溯源树
样例
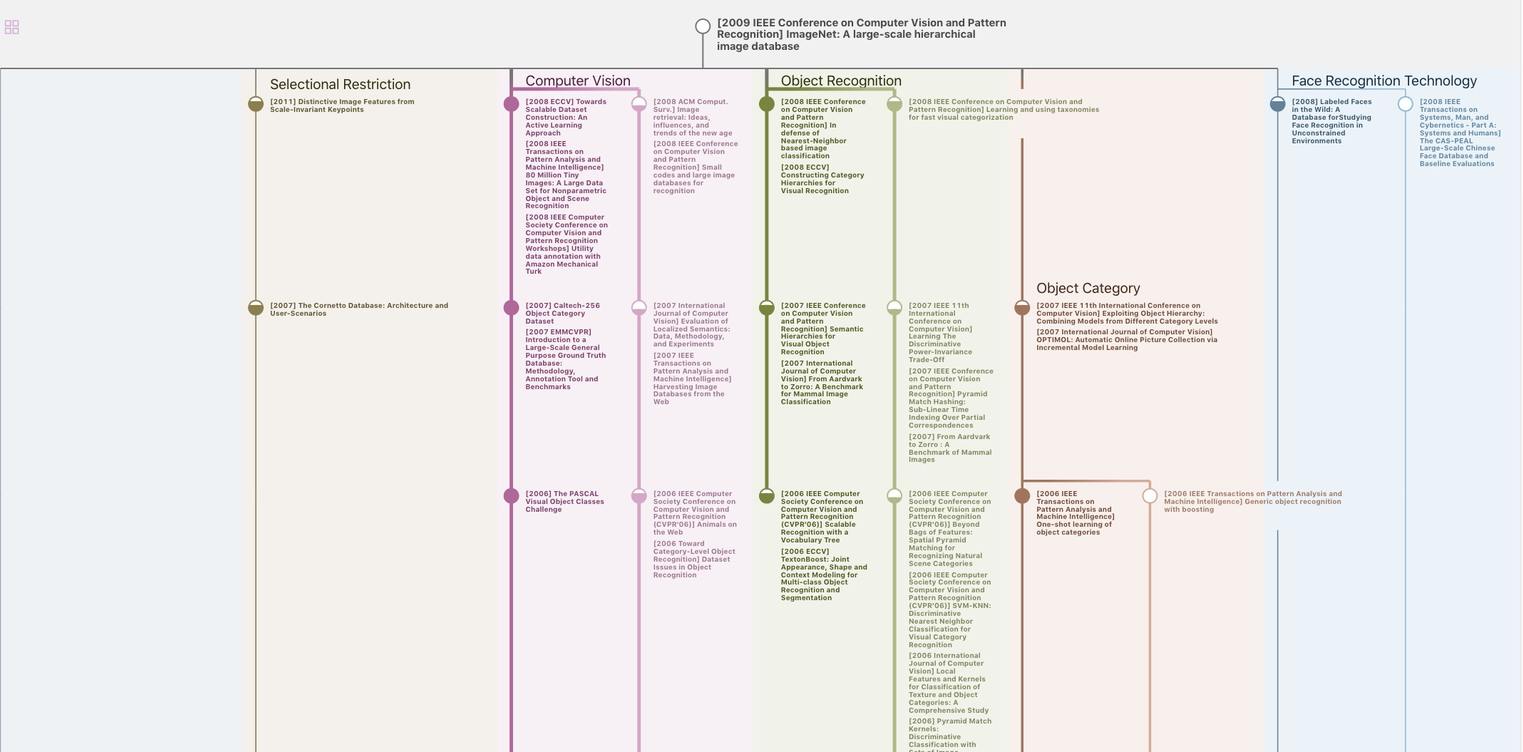
生成溯源树,研究论文发展脉络
Chat Paper
正在生成论文摘要