Improving the performance of learned descriptors in the matching of high spatial resolution aerial images by proposing a large-scale dataset of vertical images
Arabian Journal of Geosciences(2023)
摘要
Image matching is a branch of computer vision that forms the basis of most photogrammetry and remote sensing data processes. Outstanding results from recent activities in this field have shown the effectiveness of trainable descriptors. Currently, the existing datasets used to train these types of descriptors are collected based on front-view photos, and because of extractable features in these images, learned descriptors face challenges in the vertical image matching process. Also, a training dataset derived from top-view images has not been developed for the learned descriptors. To overcome these limitations, a training dataset based on remote sensing images is presented. Training patches in this collection are extracted from 61,223 images with 130 different scenes and different kind of platforms including fixed and multi-rotor unmanned aerial vehicles (UAV). To evaluate the performance of the dataset, mean average precision (mAP) criterion, ratio of descriptor distances, and point cloud density were considered. The effectiveness of the developed dataset was proved with 15% improvements in matching mAP of horizontally rotated UAV images compared to HardNet. It is also worth mentioning that the output point cloud derived from the proposed method has better quality with an average of 5426 points and 2575 accurate points on evaluation sets. By training the mentioned descriptors on the proposed dataset, it is possible to improve the stability of vertical image matching. Variety of landscapes, different imaging systems along with various environmental conditions, and finally, the appropriate architecture increase the robustness of the very high-resolution top-view image matching and make the output point cloud denser. Available at: https://github.com/farhadinima75/UAVPatches .
更多查看译文
关键词
Image matching, UAV local features, Learned descriptors, Deep learning
AI 理解论文
溯源树
样例
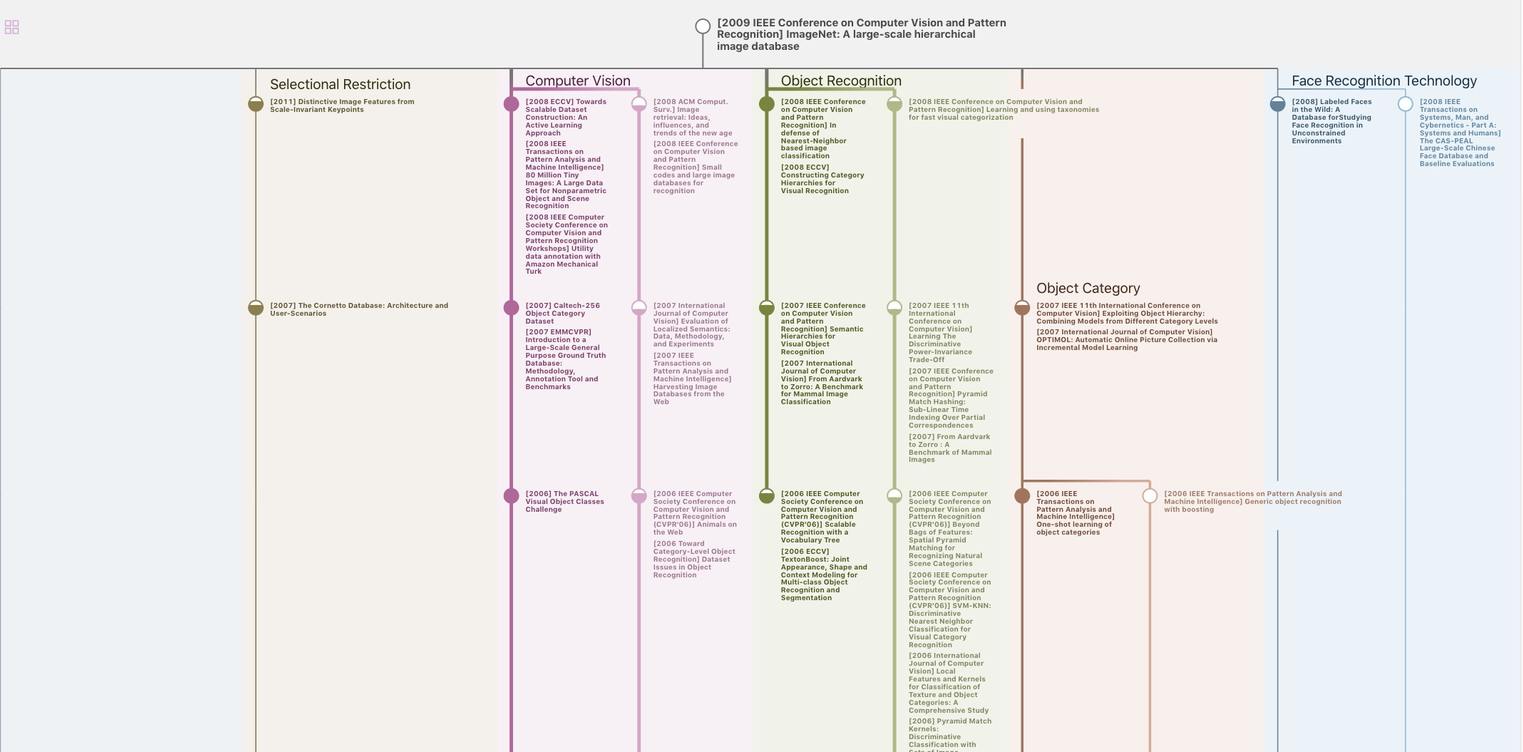
生成溯源树,研究论文发展脉络
Chat Paper
正在生成论文摘要