HDUD-Net: heterogeneous decoupling unsupervised dehaze network
Neural Computing and Applications(2023)
摘要
Haze reduces the imaging effectiveness of outdoor vision systems, significantly degrading the quality of images; hence, reducing haze has been a focus of many studies. In recent years, decoupled representation learning has been applied in image processing; however, existing decoupled networks lack a specific design for information with different characteristics to achieve satisfactory results in dehazing tasks. This study proposes a heterogeneous decoupling unsupervised dehazing network (HDUD-Net). Heterogeneous modules are used to learn the content and haze information of images individually to separate them effectively. To address the problem of information loss when extracting the content from hazy images with complex noise, this study proposes a bi-branch multi-hierarchical feature fusion module. Additionally, it proposes a style feature contrast learning method to generate positive and negative sample queues and construct contrast loss for enhancing decoupling performance. Numerous experiments confirm that the proposed algorithm achieves higher performance according to objective metrics and a more realistic visual effect when compared with state-of-the-art single-image dehazing algorithms.
更多查看译文
关键词
Image enhancement,Image restoration,Single image dehazing,Unsupervised learning
AI 理解论文
溯源树
样例
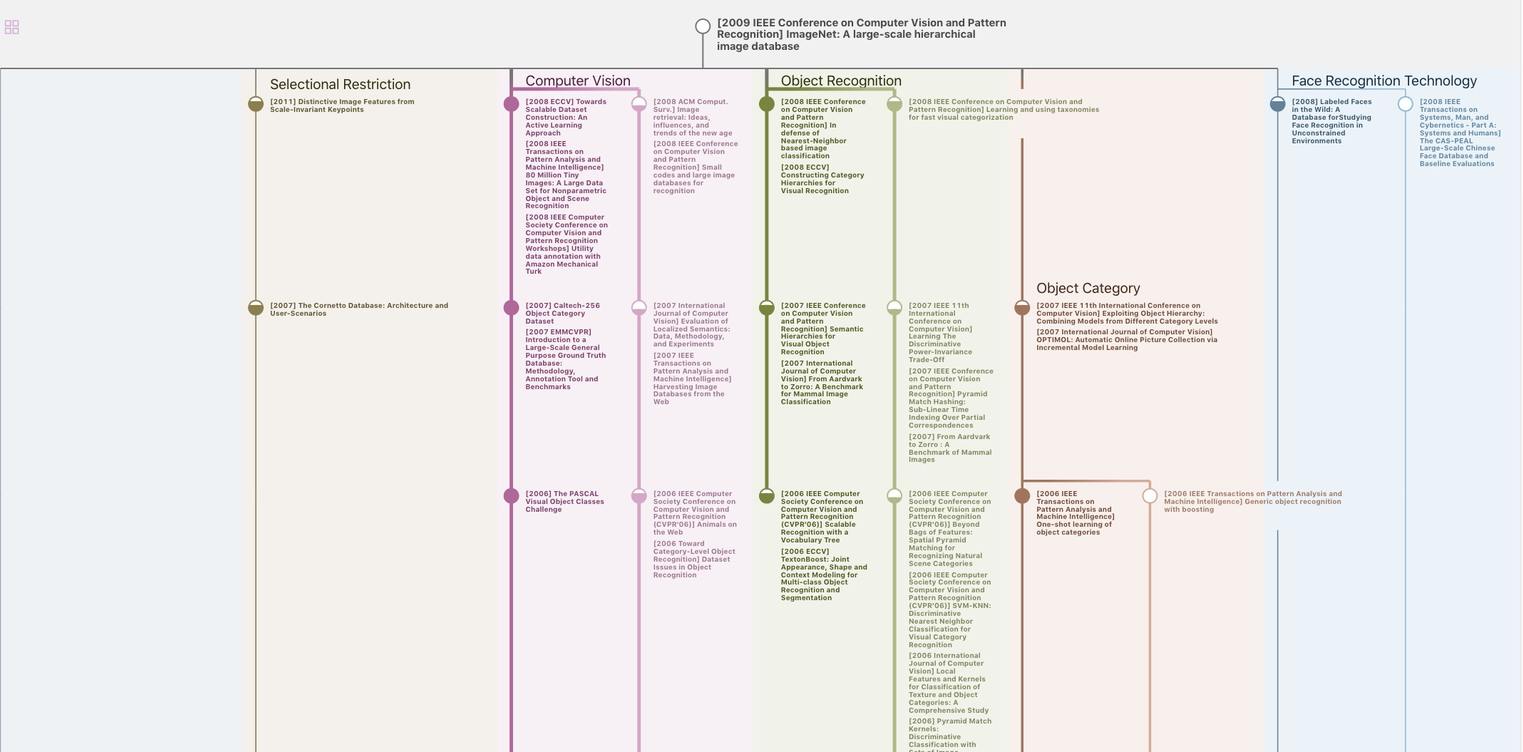
生成溯源树,研究论文发展脉络
Chat Paper
正在生成论文摘要