A Deep Learning Labeling Method for Material Microstructure Image Segmentation
PROCESSES(2023)
摘要
In the existing deep learning modeling process for material microstructure image segmentation, the manual pixel labeling process is time-consuming and laborious. In order to achieve fast and high-accuracy modeling, this work proposes a convenient deep learning labeling method and a workflow for generating a synthetic image data set. Firstly, a series of label templates was prepared by referring to the distribution of the material microstructure. Then, the typical textures of different microstructures were box-selected in the images to be segmented to form texture templates. The manual pixel labeling was simplified to the box-selection of the typical microstructure texture. Finally, a synthetic data set can be generated using the label and texture templates for further deep learning model training. Two image cases containing multiple types of microstructures were used to verify the labeling method and workflow. The results show that the pixel segmentation accuracy of the deep learning model for the test images reaches 95.92% and 95.40%, respectively. The modeling workflow can be completed within 20 min, and the labeling time that requires manual participation is within 10 min, significantly reducing the modeling time compared to traditional methods where the labeling process may take several hours.
更多查看译文
关键词
data augmentation,synthetic microstructure image,texture learning,label template
AI 理解论文
溯源树
样例
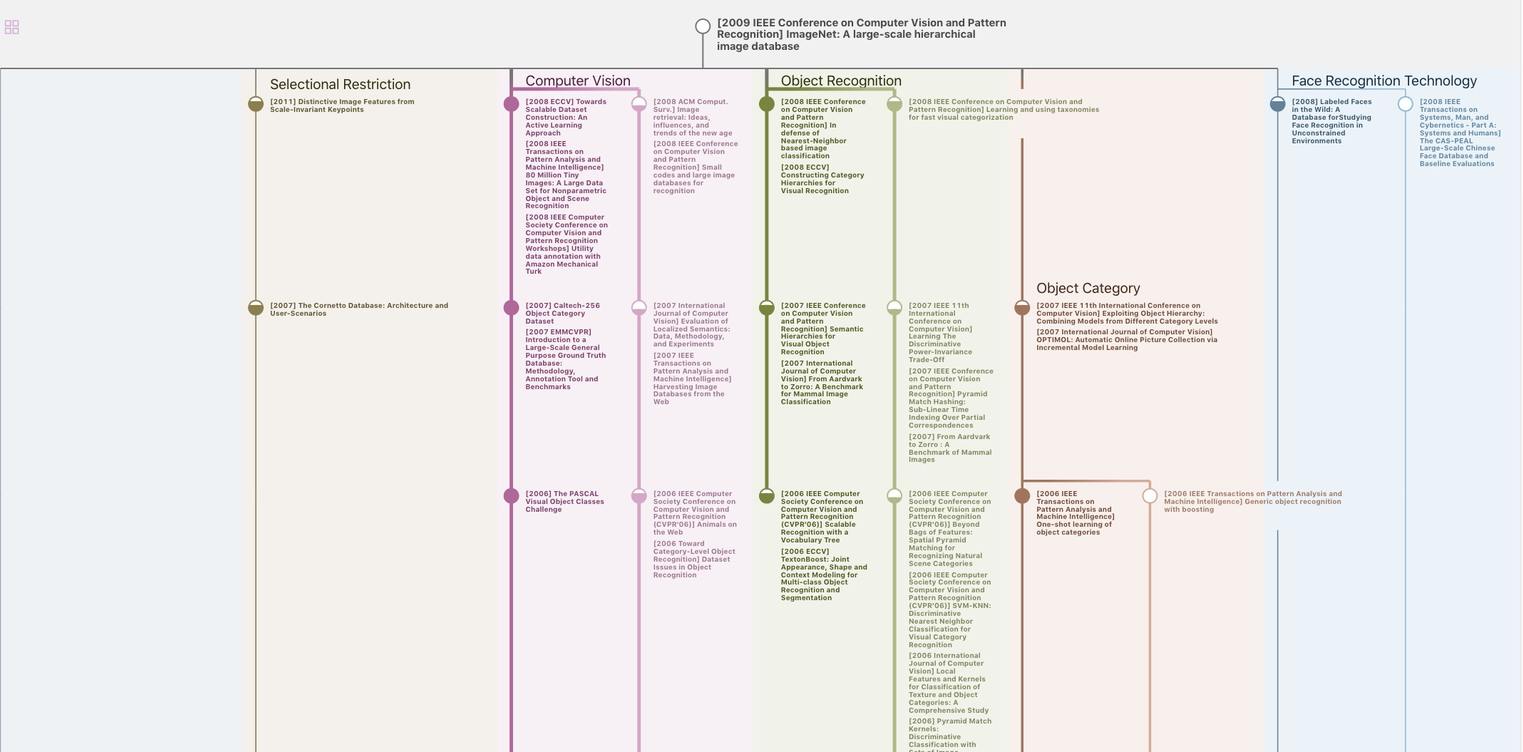
生成溯源树,研究论文发展脉络
Chat Paper
正在生成论文摘要