Sparsity and M-Estimators in RFI Mitigation for Typical Radio Astrophysical Signals
UNIVERSE(2023)
摘要
In this paper, radio frequency interference (RFI) mitigation by robust maximum likelihood estimators (M-estimators) for typical radio astrophysical signals of, e.g., pulsars and fast radio bursts (FRBs), will be investigated. The current status reveals several defects in signal modeling, manual factors, and a limited range of RFI morphologies. The goal is to avoid these defects while realizing RFI mitigation with an attempt at feature detection for FRB signals. The motivation behind this paper is to combine the essential signal sparsity with the M-estimators, which are pertinent to the RFI outliers. Thus, the sparsity of the signals is fully explored. Consequently, typical isotropic and anisotropic features of multichannel radio signals are accurately approximated by sparse transforms. The RFI mitigation problem is thus modeled as a sparsity-promoting robust nonlinear estimator. This general model can reduce manual factors and is expected to be effective in mitigating most types of RFI, thus alleviating the defects. Comparative studies are carried out among three classes of M-estimators combined with several sparse transforms. Numerical experiments focus on real radio signals of several pulsars and FRB 121102. There are two discoveries in the high-frequency components of FRB 121102-11A. First, highly varying narrow-band isotropic flux regions of superradiance are discovered. Second, emission centers revealed by the isotropic features can be completely separated in the time axis. The results have demonstrated that the M-estimator-based sparse optimization frameworks show competitive results and have potential application prospects.
更多查看译文
关键词
fast radio bursts,pulsar signals,radio frequency interference,RFI mitigation/excision,robust nonlinear filters,maximum likelihood estimators,sparse representation,wavelets,shearlets,curvelets
AI 理解论文
溯源树
样例
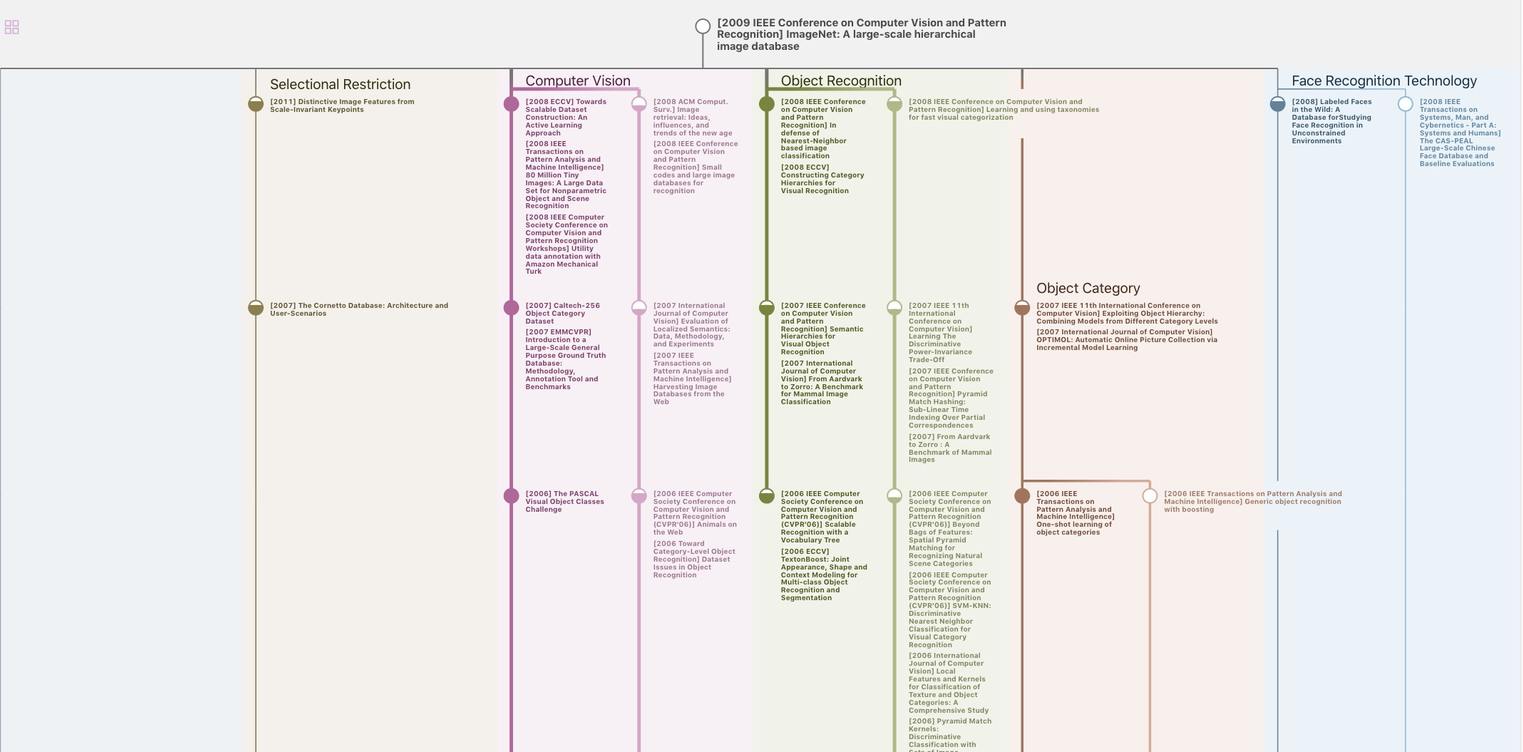
生成溯源树,研究论文发展脉络
Chat Paper
正在生成论文摘要