LW-BPNN: A Novel Feature Extraction Method for Rolling Bearing Fault Diagnosis
Processes(2023)
摘要
Efficiently diagnosing bearing faults is of paramount importance to enhance safety and reduce maintenance costs for rotating machinery. This paper introduces a novel bearing fault diagnosis method (LW-BPNN), which combines the rich properties of Legendre multiwavelet bases with the robust learning capabilities of a BP neural network (BPNN). The proposed method not only addresses the limitations of traditional deep networks, which rely on manual feature extraction and expert experience but also eliminates the complexity associated with designing and training deep network architectures. To be specific, only two statistical parameters, root mean square (RMS) and standard deviation (SD), are calculated on different Legendre multiwavelet decomposition levels to thoroughly represent more salient and comprehensive fault characteristics by using several scale and wavelet bases with various regularities. Then, the mapping relation between the extracted features and the health conditions of the bearing is automatically learned by the simpler BPNN classifier rather than the complex deep network structure. Finally, a few experiments on a popular bearing dataset are implemented to verify the effectiveness and robustness of the presented method. The experimental findings illustrate that the proposed method exhibits a high degree of precision in diagnosing various fault patterns. It outperforms other methods in terms of diagnostic accuracy, making it a viable and promising solution for real-world industrial applications in the field of rotating machinery.
更多查看译文
关键词
fault diagnosis,rolling bearings,legendre multiwavelet transform,feature extraction method
AI 理解论文
溯源树
样例
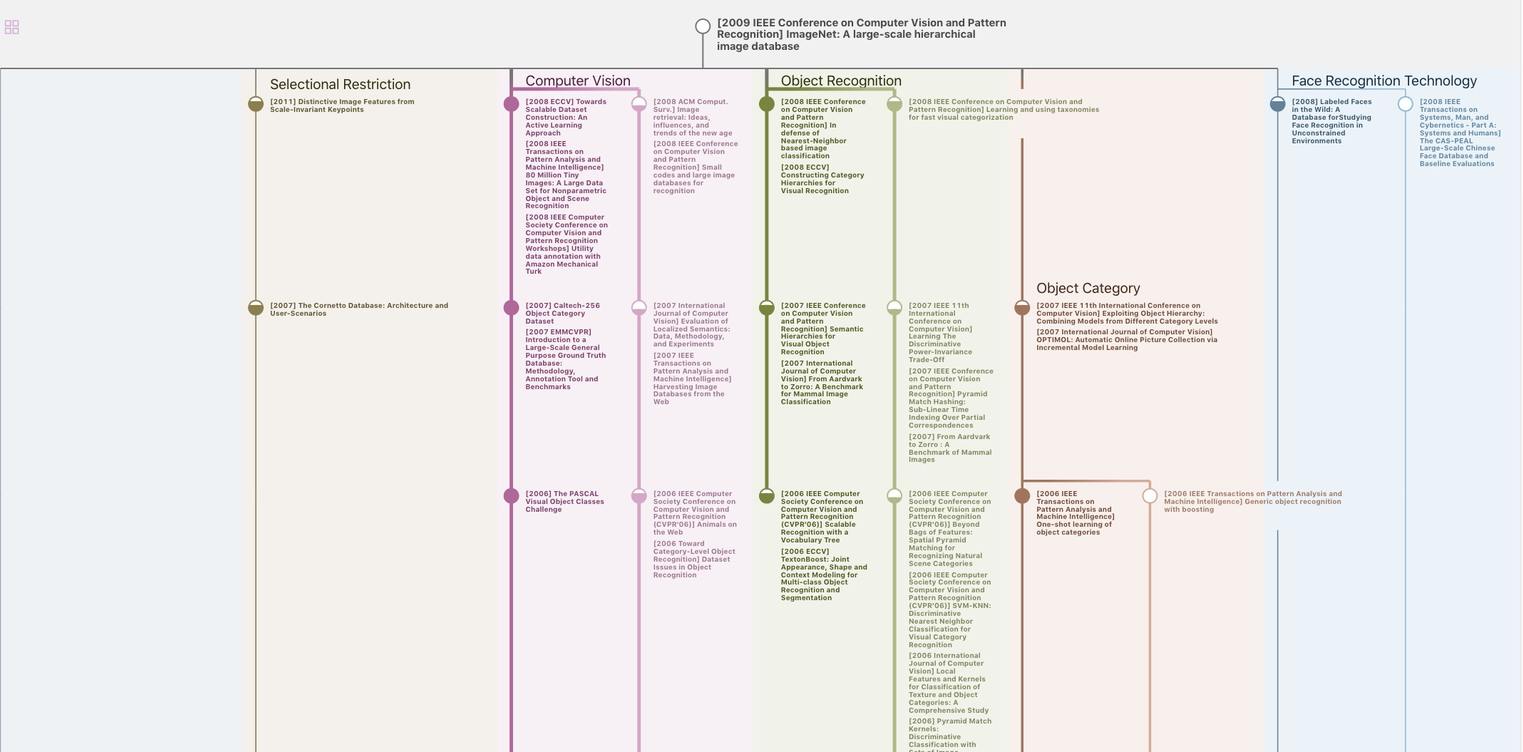
生成溯源树,研究论文发展脉络
Chat Paper
正在生成论文摘要