A Spatio-Temporal Robust Tracker with Spatial-Channel Transformer and Jitter Suppression
International Journal of Computer Vision(2023)
摘要
The robustness of visual object tracking is reflected not only in the accuracy of the target localisation in every single frame, but also in the smoothness of the predicted motion of the tracked object across consecutive frames. From the perspective of appearance modelling, the success of the state-of-the-art Transformer-based trackers derives from their ability to adaptively associate the representations of related spatial regions. However, the absence of attention in the channel dimension hinders the realisation of their potential tracking capacity. To cope with the commonly occurring misalignment of the spatial scale between the template and a search patch, we propose a novel cross channel correlation mechanism. Accordingly, the relevance of multi-channel features in the channel Transformer is modelled using two different sources of information. The result is a novel spatial-channel Transformer, which integrates information conveyed by features along both, the spatial and channel directions. For temporal modelling, to quantify the temporal smoothness, we propose a jitter metric that measures the cross-frame variation of the predicted bounding boxes as a function of the parameters such as centre displacement, area, and aspect ratio. As the changes of an object between consecutive frames are limited, the proposed jitter loss can be used to monitor the temporal behaviour of the tracking results and penalise erroneus predictions during the training stage, thus enhancing the temporal stability of an appearance-based tracker. Extensive experiments on several well-known benchmarking datasets demonstrate the robustness of the proposed tracker.
更多查看译文
关键词
Visual object tracking,Visual transformer,Temporal modelling
AI 理解论文
溯源树
样例
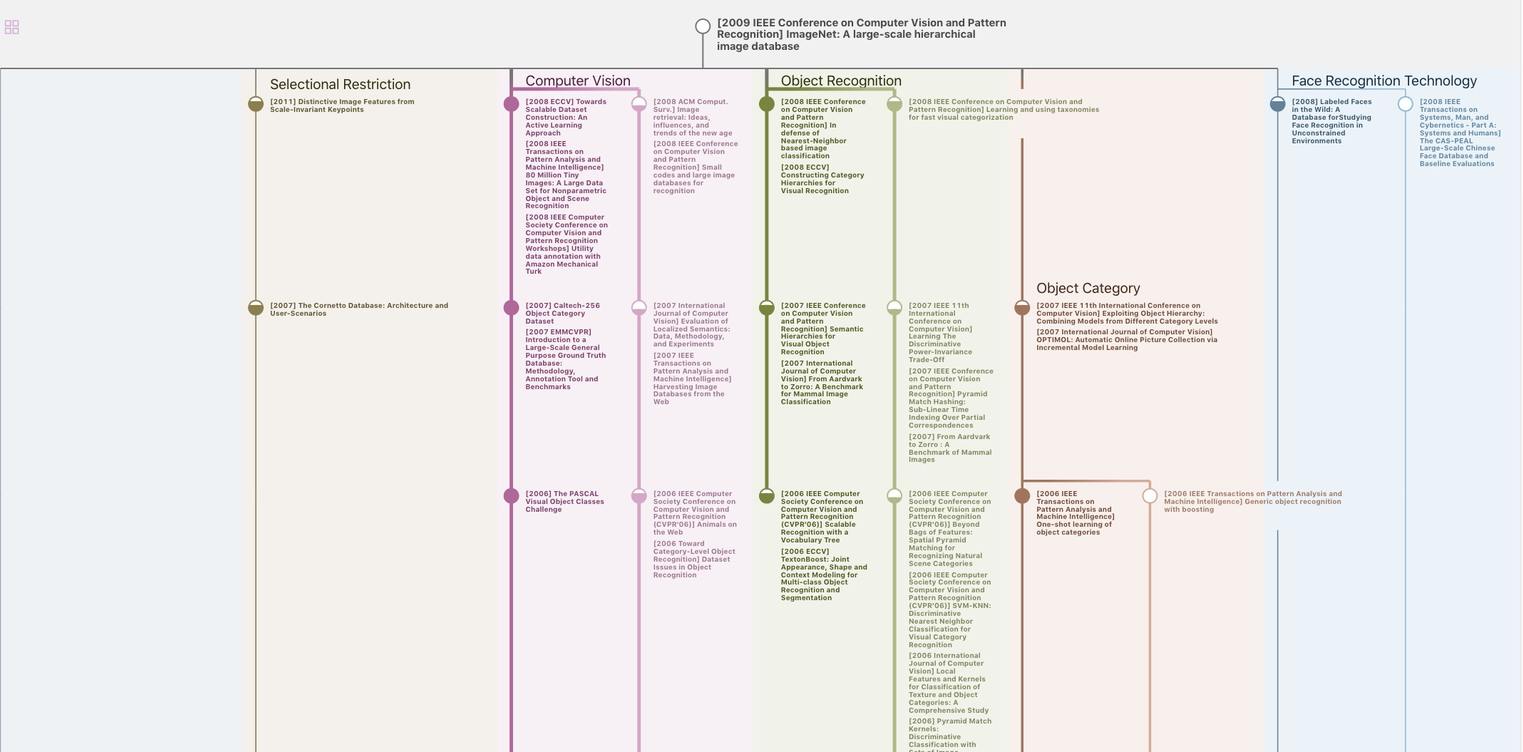
生成溯源树,研究论文发展脉络
Chat Paper
正在生成论文摘要