Hyperparameter optimization: a comparative machine learning model analysis for enhanced heart disease prediction accuracy
Multimedia Tools and Applications(2023)
摘要
An optimizer is the process of hyperparameter tuning that updates the machine learning model after each step of weight loss adjustment of input features. The permutation and combination of high and low learning rates with various step sizes ultimately leads to an optimal tuning model. The step size and learning rate sometimes take much smaller steps, allowing the derivatives of tangent to gradually reach global minima. The primary goal of this study is to compare the prediction accuracy of enhanced heart disease using various optimization algorithms. Heart disease treatment requires ensemble hyperparameter tuning for accurate prediction and classification due to multiple feature dependencies. The study analyzed model tuning techniques using the AUC and confusion matrix, revealing improvements in precision, recall, and f1 score from default to optimized models. The Hyper-opt in Bayesian optimizer and T-pot classifiers were used in genetic populations and offspring with 5 and 10 generations, while using Optuna optimization frozen trails was combined with a random forest algorithm. The default random forest (86.6
更多查看译文
关键词
Bayesian optimization,Genetic optimization,GAsearchCV optimization,Optuna optimization,Gaussian,Random forest,Support vector machine,Principal component analysis
AI 理解论文
溯源树
样例
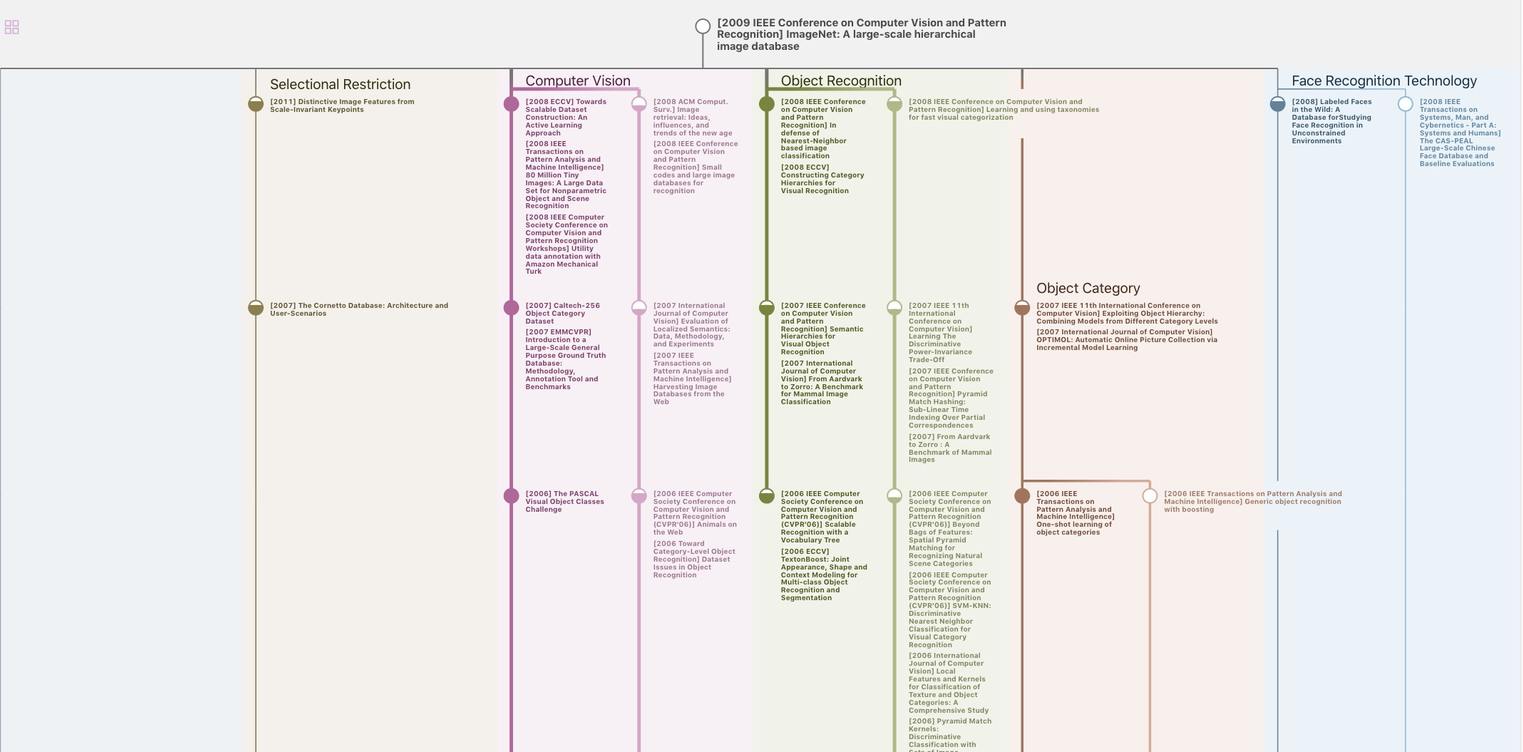
生成溯源树,研究论文发展脉络
Chat Paper
正在生成论文摘要