ML-IDS: an efficient ML-enabled intrusion detection system for securing IoT networks and applications
Soft Computing(2023)
摘要
The Internet of Things (IoT) and machine learning (ML) have various applications in different sectors of life, such as healthcare, agriculture, industries, transportation, smart cities, smart homes, etc., and their number is increasing with each passing day. The rapid development of IoT and its increasing demand in different fields of life create a serious problem of security for the IoT environment, which needs serious consideration to protect the IoT-enabled systems from external networks and cyber-attacks. Because of the open deployment environment and constrained resources, the IoT is prone to malicious assaults. Furthermore, the IoT’s diverse and dispersed properties make it difficult for conventional intrusion detection systems (IDS) to keep up with current technological developments. An ML-enabled IoT-based IDS is one of the most important security methods that can assist in defending computer networks and the IoT environment from numerous attacks and malicious activities. Keeping in mind the significant contribution of ML to securing the IoT environment, we proposed an ML-enabled IDS for securing the IoT networks and applications in this study. In the proposed system, we proposed a modified Random Forest (RF) algorithm and compared its performance with nine well-known ML algorithms for the detection of network attacks. Further, two of the most recent and well-known network datasets, i.e., TON-IoT and UNSW-NB15, are used to check the effectiveness of the ML-enabled IDS. The performance of the utilized ML algorithms was measured with the help of different performance measures such as accuracy, sensitivity, etc. The experimental outcomes illustrate the importance of the proposed ML-enabled IDS for securing the IoT environment and applications. The proposed system applies to almost all of the resource-constrained devices that use the IoT network.
更多查看译文
关键词
Internet of Things,Intrusion detection system,Machine learning,Security and privacy,Security-enabled IoT,TON-IoT,UNSW-NB15
AI 理解论文
溯源树
样例
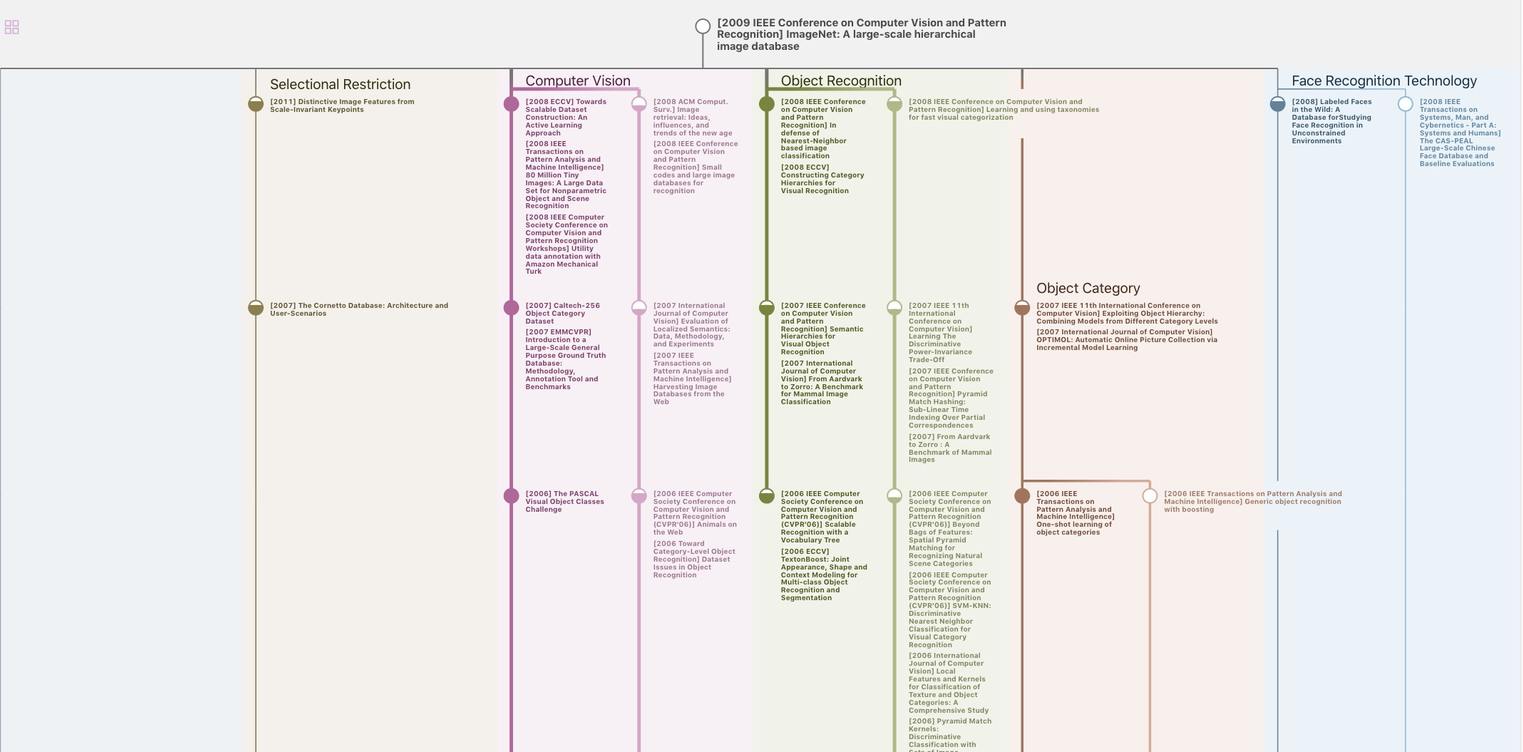
生成溯源树,研究论文发展脉络
Chat Paper
正在生成论文摘要