MSC-DeepFM: OSM Road Type Prediction via Integrating Spatial Context Using DeepFM
SUSTAINABILITY(2023)
摘要
The quality of OpenStreetMap (OSM) has been widely concerned as a valuable source for monitoring some sustainable development goals (SDG) indicators. Improving its semantic quality is still challenging. As a kind of solution, road type prediction plays an important role. However, most existing algorithms show low accuracy, owing to data sparseness and inaccurate description. To address these problems, we propose a novel OSM road type prediction approach via integrating multiple spatial contexts with DeepFM, named MSC-DeepFM. A deep learning model DeepFM is used for dealing with data sparseness. Moreover, multiple spatial contexts (MSC), including the features of intersecting roads, surrounding buildings, and points of interest (POIs), are distilled to describe multiple types of road more accurately. The MSC combined with geometric features and restricted features are put into DeepFM, in which the low-order and high-order features fully interact. And a multivariate classifier OneVsRest is adopted to predict road types. Experiments on OSM show that the proposed model MSC-DeepFM achieves excellent performance and outperforms some state-of-the-art methods.
更多查看译文
关键词
road type prediction,multiple spatial contexts (MSC),DeepFM,OpenStreetMap,deep learning
AI 理解论文
溯源树
样例
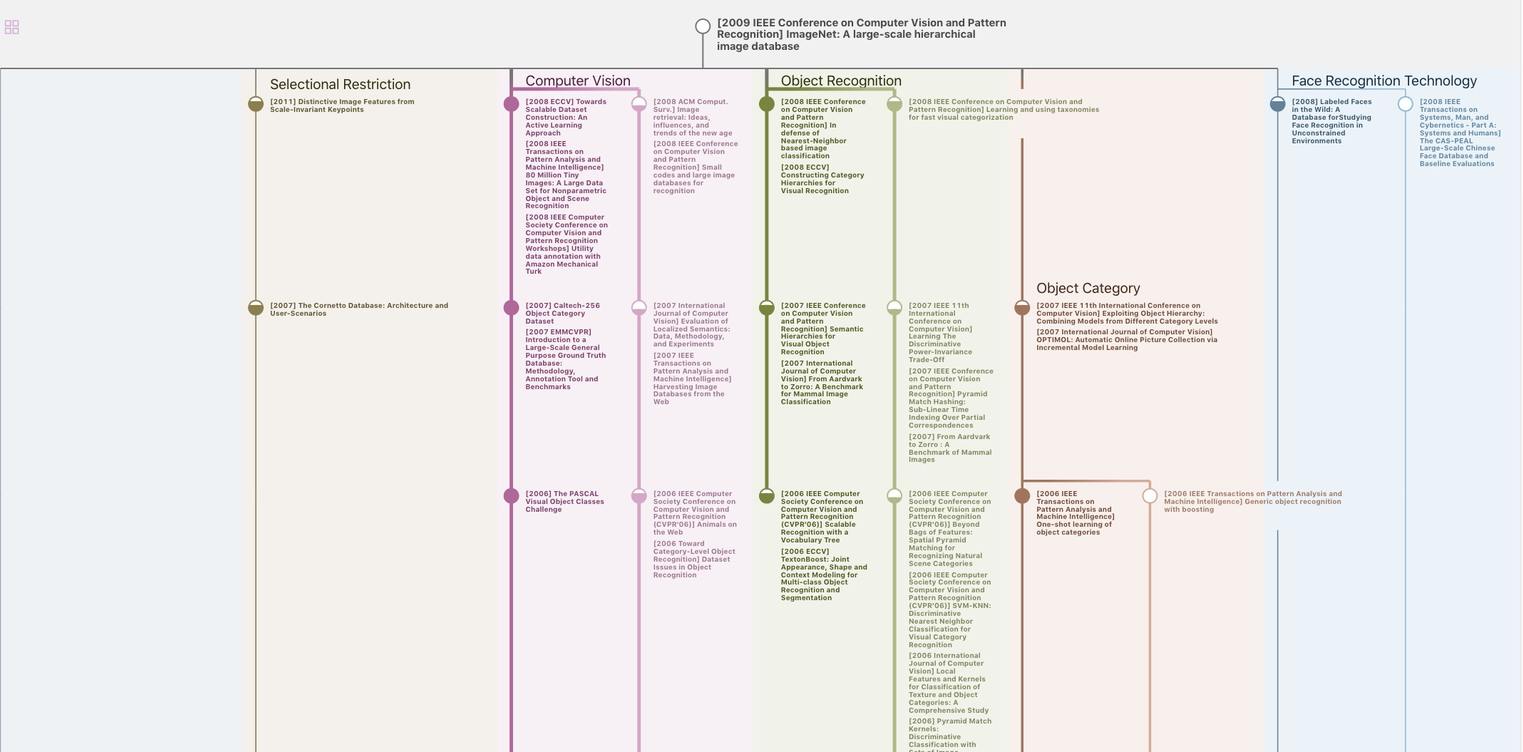
生成溯源树,研究论文发展脉络
Chat Paper
正在生成论文摘要