A holistic view on positive and negative implicit feedback for micro-video recommendation
KNOWLEDGE-BASED SYSTEMS(2024)
摘要
Micro-video online platforms have become prevalent in recent years, necessitating effective recommender systems to help identify users' preferences. Previous works have made significant efforts to leverage the multi -modal information and leverage the multi-interests of users to enhance the recommendation performance. Despite their effectiveness, they ignored valuable information implied in users' negative feedback. Moreover, they did not consider the fact that the positive and negative implicit behaviors form a holistic sequence (exposure sequence) in the micro-video recommendation. Additionally, they failed to capture sequential and non-sequential dependencies among multi-type behaviors in users' long interactive sequence. To address these issues, we devise a HOlistic framework to understand the natural distinction and inherent connection between positive and negative feedback for micro-video recommendation with Multiplex Networks, named HMNet. Specifically, the HMNet framework first builds a hierarchical architecture to distinguish two types of behaviors: the exposure layer represents observations (including skips and clicks), while the conversion layer is on behalf of clicks. We further propose two multiplex networks to capture dependencies within and across the two layers. In particular, a multiplex recurrent neural network is proposed to encode sequential cross-type dependencies, while a relation-aware multiplex graph convolutional network is to model the non-sequential semantic dependencies between multi-type behaviors. Extensive experiments on two public real-world datasets demonstrate the effectiveness of our model compared with the state-of-the-art methods.
更多查看译文
关键词
Micro-video,Multi-behavior recommendation,Multiplex network,Recurrent neural network,Exposure sequence
AI 理解论文
溯源树
样例
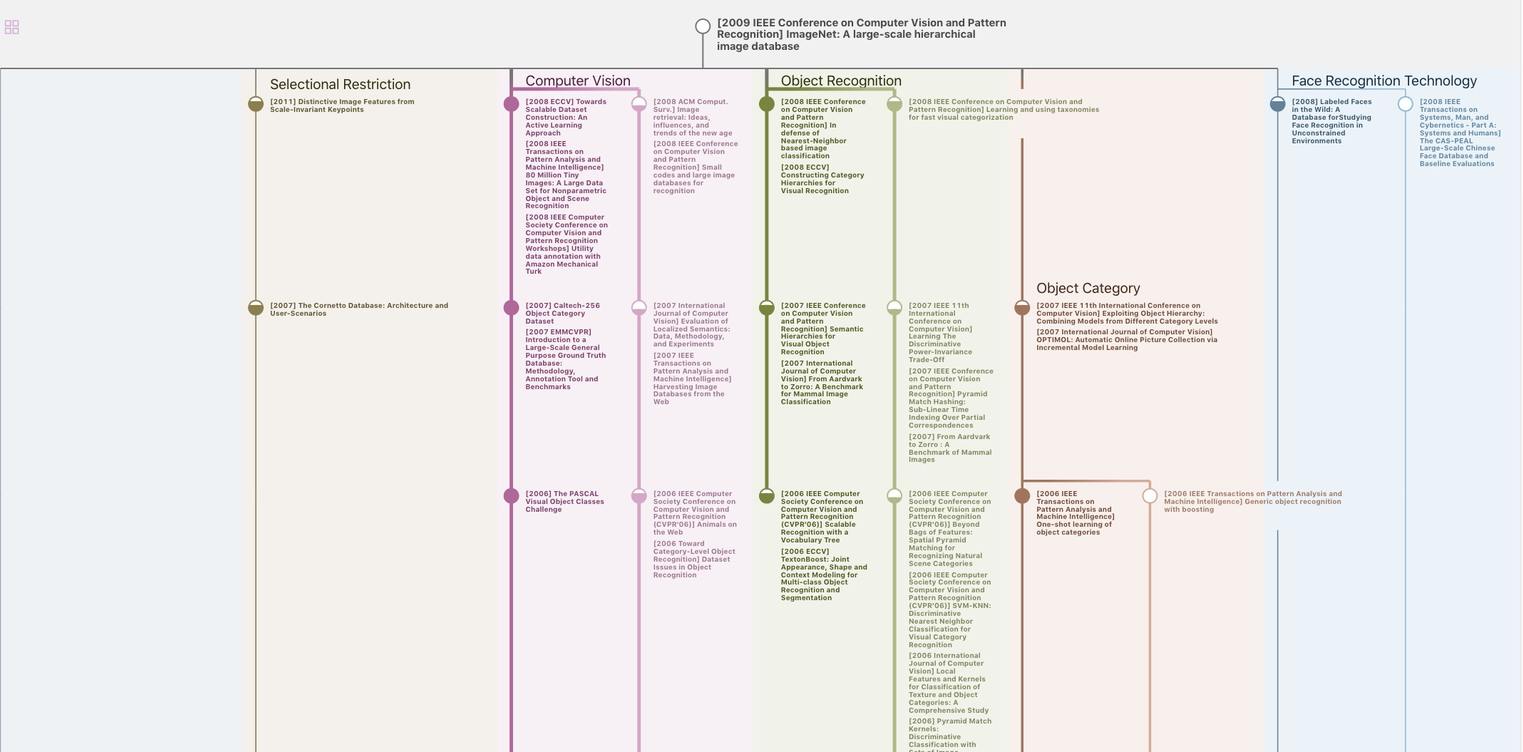
生成溯源树,研究论文发展脉络
Chat Paper
正在生成论文摘要