Optimal domain adaptive object detection with self-training and adversarial-based approach for construction site monitoring
AUTOMATION IN CONSTRUCTION(2024)
摘要
In practice, object detection models used for construction site monitoring exhibit performance degradation owing to different monitoring settings and dynamic construction environments. Collecting and labeling images from new construction sites is necessary to mitigate these challenges; however, this requires significant re-sources. This paper presents an optimal domain-adaptive object detection model that employs an unsupervised domain adaptation approach to address the performance degradation challenges. Experiments were conducted at two construction sites to validate and identify the most effective domain-adaptive object detection model for construction sites. Several domain-adaptive object detection models have been developed, and our experimental results demonstrate that a hybrid approach, combining self-training and adversarial-based approaches improves the mean average precision by 12.8 and 7.1 over that of the baseline model at each construction site. These findings underscore the potential of domain adaptation-based methods to train object detection models in the construction domain, offering improved performance and reduced labeling efforts at specific target construction sites.
更多查看译文
关键词
Construction sites monitoring,Computer vision,Unsupervised domain adaptation,Domain adaptive object detection,Self-training,Adversarial-based domain adaptation
AI 理解论文
溯源树
样例
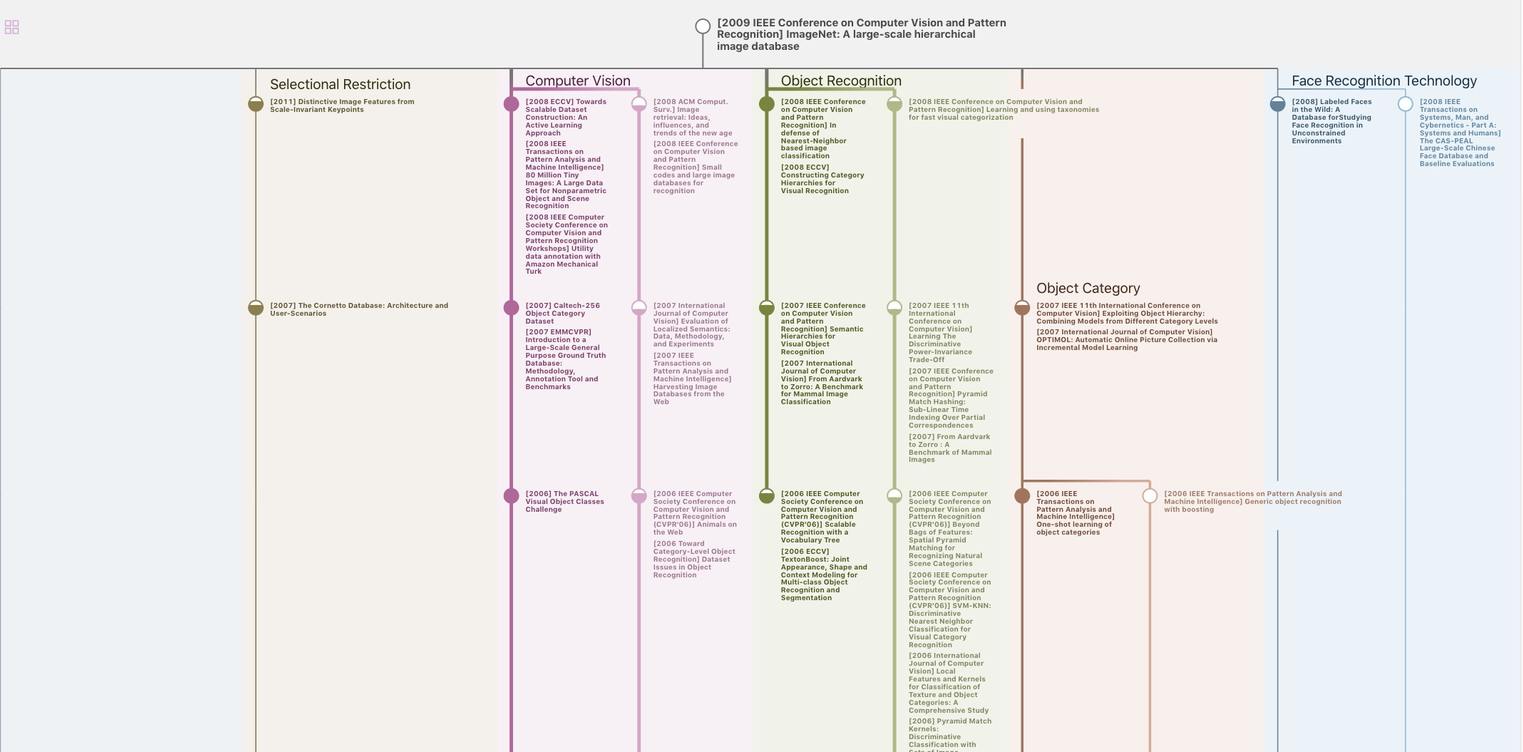
生成溯源树,研究论文发展脉络
Chat Paper
正在生成论文摘要