Mesoscale material modeling with memoryless isotropic point particles
JOURNAL OF COMPUTATIONAL SCIENCE(2024)
摘要
There has been a proliferation of particle systems developed to model complex systems. These are attractive because they are mesh-free, avoiding issues associated with solver remeshing and convergence. They have however fragmented into niches, using increasingly complex particles that introduce internal degrees of freedom and external solver coupling. We show that, contrary to prior assumptions in the literature, memoryless isotropic point particles can model material properties including anisotropy, hysteresis, and failure solely through the statistics of their distributions. The resulting models offer compact code that is straightforward to accelerate and port, can span between micro- and macro-structure, require few parameters to set up a simulation, and unlike high-dimensional machine learning models they use a low-dimensional representation that can be efficiently learned. Rather than deriving them as approximations to either molecular dynamics or partial differential equations we investigate how these models can be found directly, and illustrate this with both qualitative comparisons of phenomenology and quantitative comparisons of predictions.
更多查看译文
关键词
Particle systems,Discrete element method,Molecular dynamics,Machine learning,Anisotropy,Hysteresis,Failure,Composites
AI 理解论文
溯源树
样例
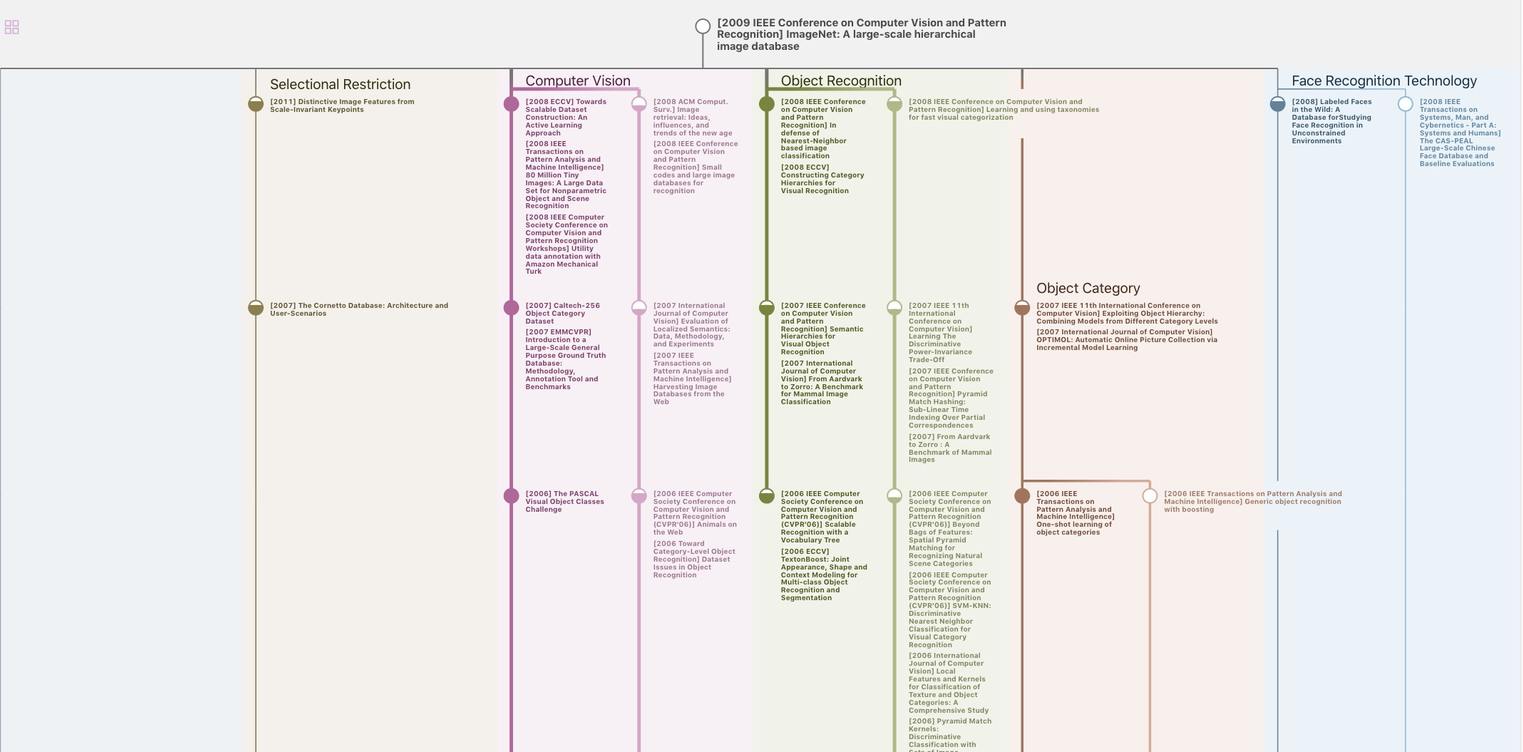
生成溯源树,研究论文发展脉络
Chat Paper
正在生成论文摘要