A Novel Multi-Scale Graph Neural Network for Metabolic Pathway Prediction
IEEE-ACM TRANSACTIONS ON COMPUTATIONAL BIOLOGY AND BIOINFORMATICS(2024)
摘要
Predicting the metabolic pathway classes of compounds in the human body is an important problem in drug research and development. For this purpose, we propose a Multi-Scale Graph Neural Network framework, named MSGNN. The framework includes a subgraph encoder, a feature encoder and a global feature processor, and a graph augmentation strategy is adopted. The subgraph encoder is responsible for extracting the local structural features of the compound, the feature encoder learns the characteristics of the atoms, and the global feature processor processes the information from the pre-training model and the two molecular fingerprints, while the graph augmentation strategy is to expand the train set through a scientific and reasonable method. The experiment result illustrates that the accuracy, precision, recall and F1 metrics of MSGNN reach 98.17%, 94.18%, 94.43% and 94.30%, respectively, which is superior to the similar models we have known. In addition, the ablation experiment demonstrates the indispensability of MSGNN modules.
更多查看译文
关键词
Feature extraction,Compounds,Graph neural networks,Drugs,Chemicals,Support vector machines,Training,Metabolic pathway prediction,graph neural network,machine learning
AI 理解论文
溯源树
样例
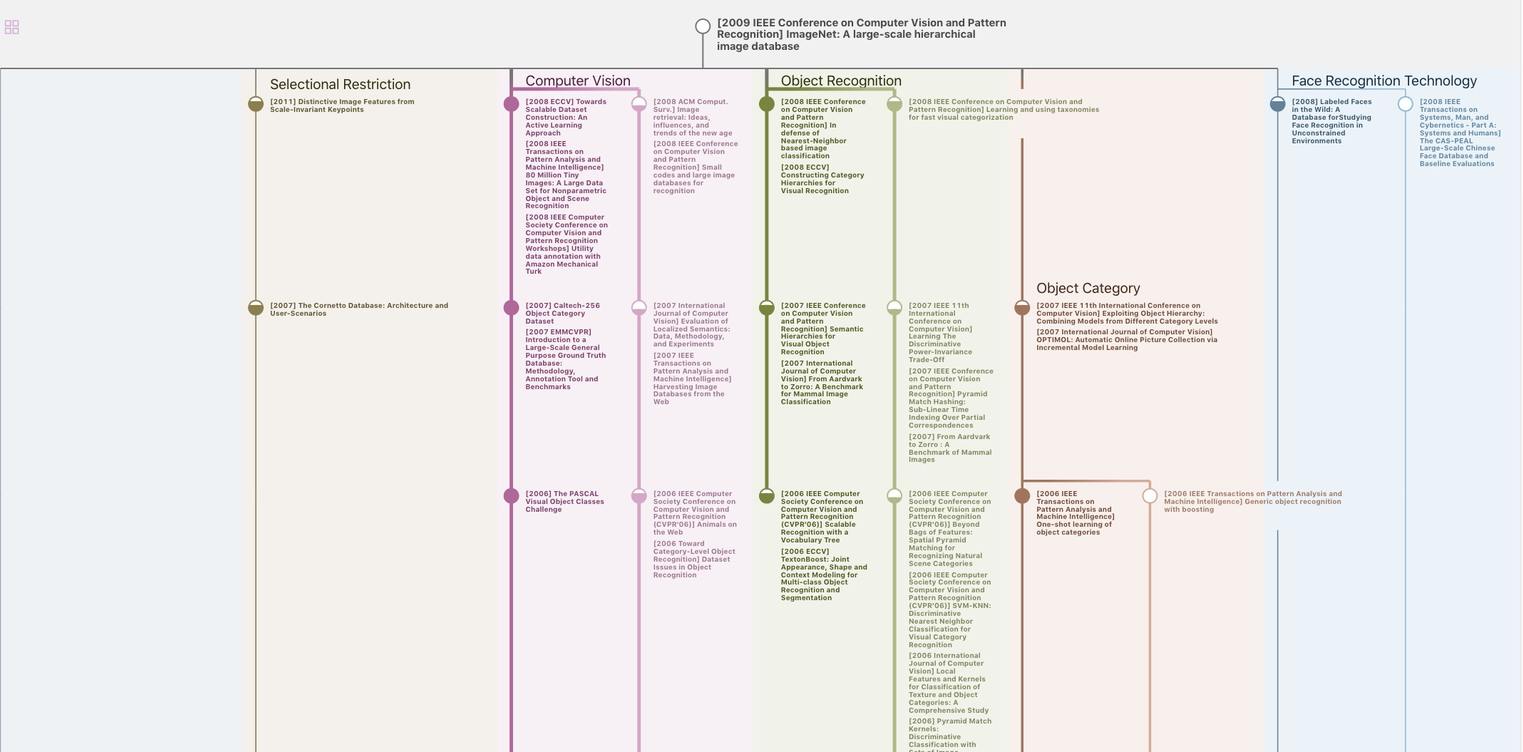
生成溯源树,研究论文发展脉络
Chat Paper
正在生成论文摘要