Multiscale Deep Attention Q Network: A New Deep Reinforcement Learning Method for Imbalanced Fault Diagnosis in Gearboxes
IEEE TRANSACTIONS ON INSTRUMENTATION AND MEASUREMENT(2024)
摘要
Ensuring the safety of mechanical driving systems relies heavily on accurate gearbox fault diagnosis. However, the presence of actual multiworking conditions and uneven sample distribution makes fault diagnosis in gearboxes more challenging. Although intelligent fault diagnosis (IFD) employing convolutional neural networks (CNNs) has shown promising results, they often require strong feedback learning and experienced adjustment of hyperparameters for different tasks. In this article, a novel approach called multiscale deep attention Q network (MDAQN) is proposed for imbalanced gearbox fault diagnosis from a deep reinforcement learning (DRL) perspective. An imbalanced classification Markov decision process (ICMDP) is introduced that considers interclass deviation, serving as an environment simulation to enhance classification policy learning under data imbalance. In addition, a new multiscale attention convolution network is designed as the agent structure of the deep Q network (DQN) algorithm, thereby improving the discriminative feature learning ability under complex running conditions. By employing weak feedback interaction from DRL, the diagnostic model is trained to enable imbalanced gearbox fault diagnosis effectively. Experimental results on three gearbox imbalanced datasets demonstrate that MDAQN exhibits superior feature extraction ability and generalization, achieving an accuracy of over 99.0% when compared to multiple existing approaches.
更多查看译文
关键词
Attention,deep reinforcement learning (DRL),gearbox,imbalanced fault diagnosis,multiscale learning
AI 理解论文
溯源树
样例
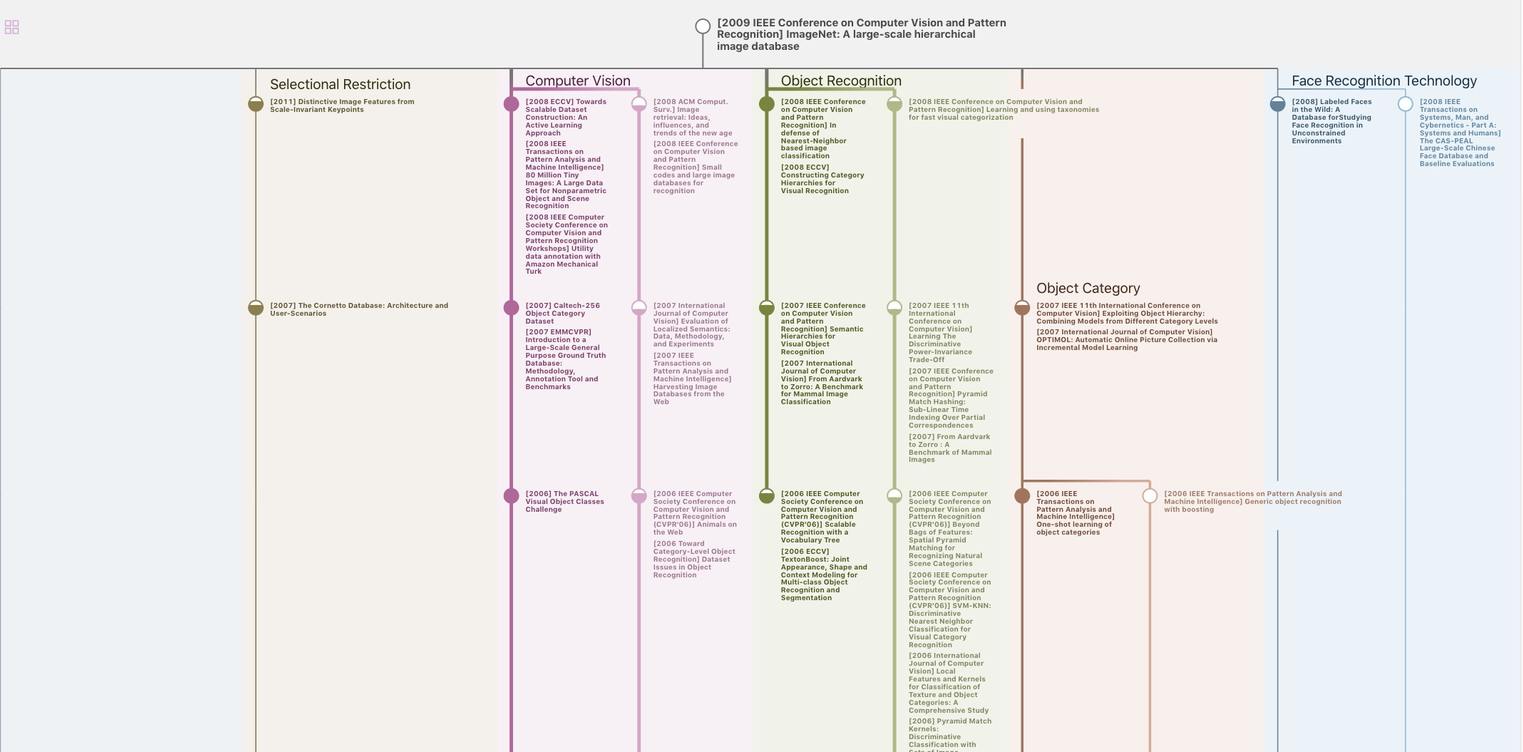
生成溯源树,研究论文发展脉络
Chat Paper
正在生成论文摘要