LLD-MFCOS: A Multiscale Anchor-Free Detector Based on Label Localization Distillation for Wheelset Tread Defect Detection
IEEE TRANSACTIONS ON INSTRUMENTATION AND MEASUREMENT(2024)
摘要
Wheelset surface defect detection is an important aspect of train safety maintenance. However, due to the constraints of low industrial computing power, multiscale defects, and complex backgrounds, conventional defect detection methods are difficult to fully exploit their detection performance. To solve the above issues, we proposed a novel multiscale anchor-free detector based on a label localization distillation strategy, called LLD-MFCOS. In the proposed method, we designed a cross-layer refined feature pyramid network (CR-FPN) for the multiscale problem of tread defects. It integrates and allocates the multilevel features in the backbone network to the lateral connection of feature pyramid network (FPN) to improve the ability of multiscale feature extraction. To weaken the influence of complex background, a newly designed hybrid nonlocal (HNL) module, which is obtained by extending the channel pipeline in the nonlocal (NL) module, is introduced into the backbone to improve the extraction efficiency of discriminative features in key regions and shape information. To effectively compress and simplify the detection models, we proposed a label localization knowledge distillation strategy. It first locates the main and secondary distillation regions according to the ground truth and background information in the labels and then performs the distillation task on the localization head and classification head of the detector, respectively. In this way, the distillation efficiency can be improved while avoiding the confusion of localization knowledge and classification knowledge. Finally, we demonstrated the effectiveness and superiority of the proposed module and distillation method through comparative experiments, ablation experiments, generalization performance experiments, and visualization analysis and found that LLD-MFCOS can improve the mAP of the student network by about 2.4%-4.8%. It means that the proposed method can effectively achieve the balance between detection speed and accuracy.
更多查看译文
关键词
Attention mechanism,deep learning,feature pyramid network (FPN),knowledge distillation,wheelset tread defect detection
AI 理解论文
溯源树
样例
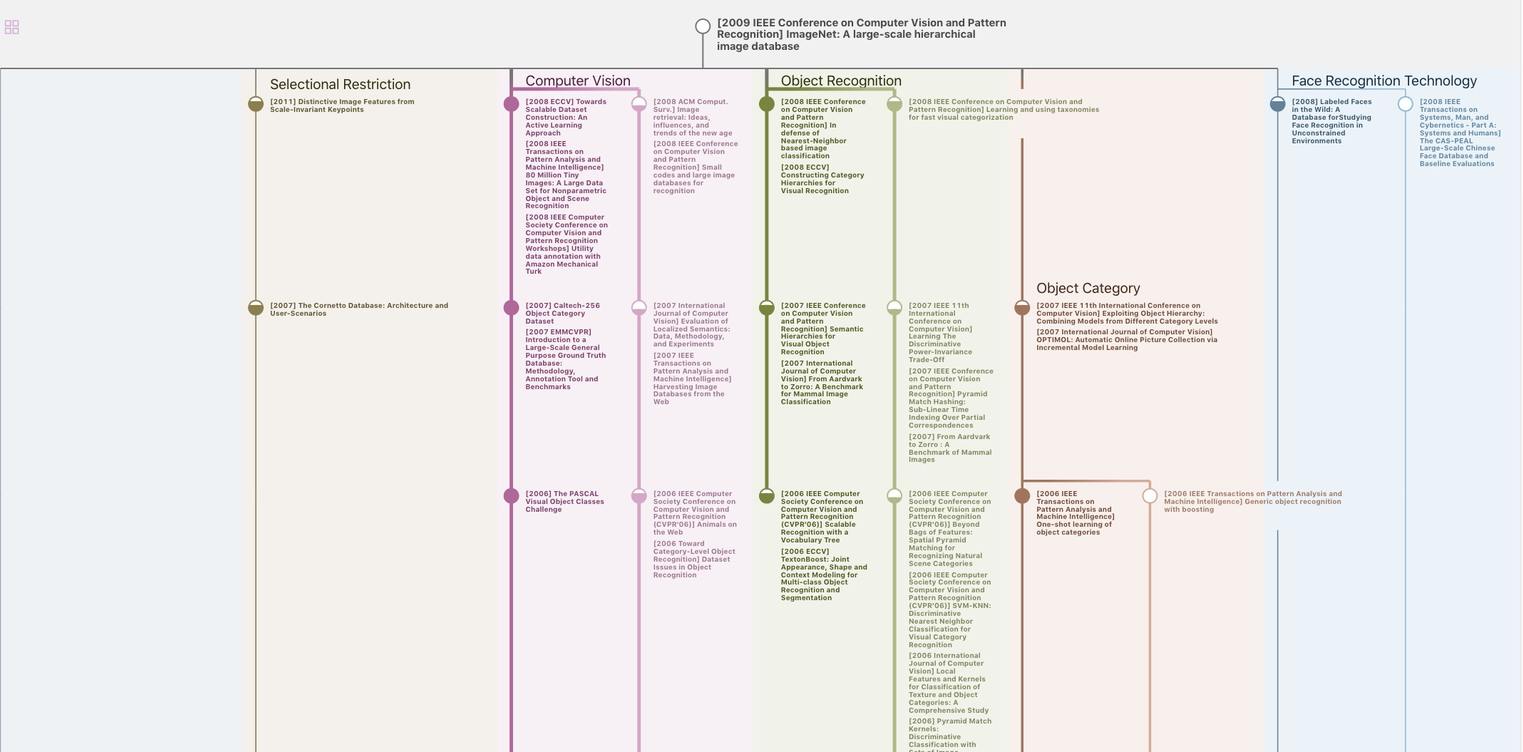
生成溯源树,研究论文发展脉络
Chat Paper
正在生成论文摘要