Welfare Effects of Ex-Ante Bias and Tie-Breaking Rules on Observational Learning with Fake Agents.
2023 21st International Symposium on Modeling and Optimization in Mobile, Ad Hoc, and Wireless Networks (WiOpt)(2023)
摘要
Networks that provide agents with access to a common database of the agents' actions enable an agent to easily learn by observing the actions of others, but are also susceptible to manipulation by “fake” agents. Prior work has studied a model for the impact of such fake agents on ordinary (rational) agents in a sequential Bayesian observational learning framework. That model assumes that ordinary agents do not have an ex-ante bias in their actions and that they follow their private information in case of an ex-post tie between actions. This paper builds on that work to study the effect of fake agents on the welfare obtained by ordinary agents under different ex-ante biases and different tie-breaking rules. We show that varying either of these can lead to cases where, unlike in the prior work, the addition of fake agents leads to a gain in welfare. This implies that in such cases, if fake agents are absent or are not adequately present, an altruistic platform could artificially introduce fake actions to effect improved learning.
更多查看译文
关键词
Information cascades,herding,Bayesian optimality,ex-ante bias
AI 理解论文
溯源树
样例
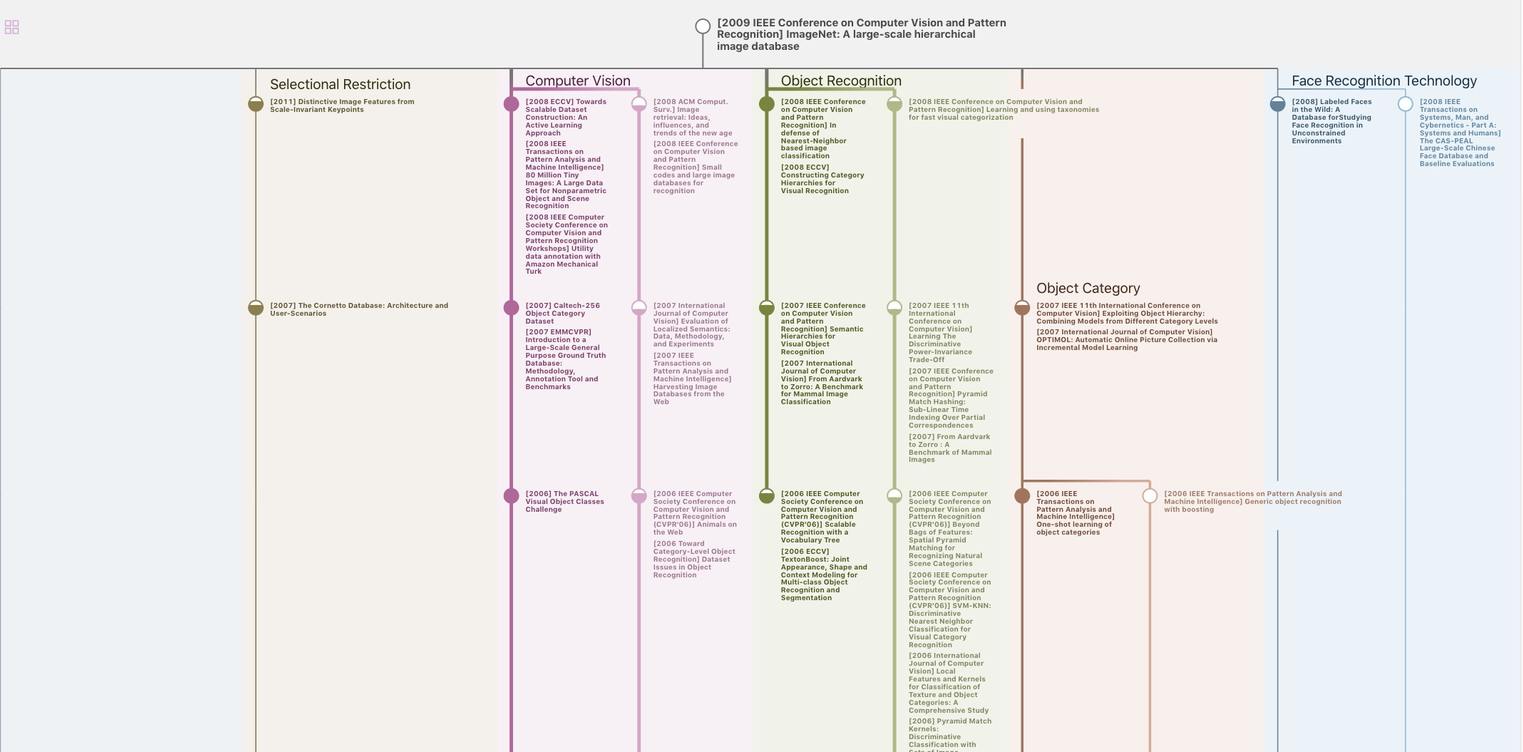
生成溯源树,研究论文发展脉络
Chat Paper
正在生成论文摘要