Age-of-Information Minimization for Energy Harvesting Sensor in Non-Stationary Environment.
2023 21st International Symposium on Modeling and Optimization in Mobile, Ad Hoc, and Wireless Networks (WiOpt)(2023)
摘要
In this paper, we consider age-of-information (AoI) minimization for an energy harvesting (EH) source that samples and sends the observations to a sink node. At each time, the source observes the channel quality, the available energy, and the age, and decides whether to sample and send the observation to the sink. We formulate the problem as a Markov decision process (MDP) and establish a threshold structure for the optimal sampling policy. Next, motivated by the popular sliding window upper confidence bound reinforcement learning (SW-UCRL2) algorithm for non-stationary reinforcement learning (RL), we leverage this threshold structure and propose an age-energy-channel based SW-UCRL2 (AEC-SW-UCRL2) algorithm to handle unknown and time-varying energy harvesting rate and channel statistics, when an upper bound to the total variation of each of these quantities over the time horizon is available. Next, for the case when these variation budgets are not available, motivated by the popular bandit-over-RL (BORL) algorithm, we propose an algorithm named age-energy-channel based bandit-over-reinforcement learning (AEC-BORL). Finally, we demonstrate the superior numerical performance of these proposed algorithms via numerical experiments.
更多查看译文
关键词
Age-of-information,Non-stationary Reinforcement learning (RL),Markov Decision Process
AI 理解论文
溯源树
样例
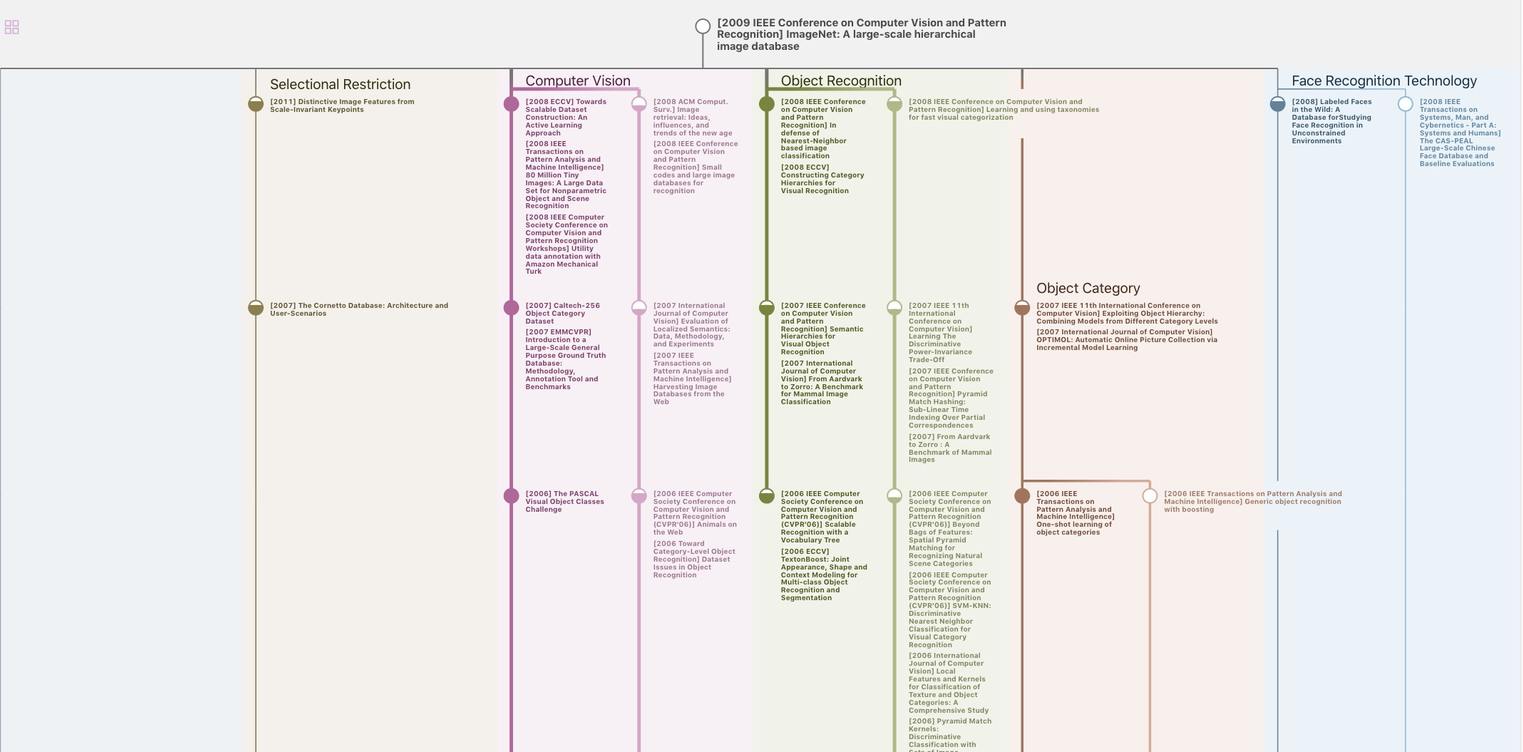
生成溯源树,研究论文发展脉络
Chat Paper
正在生成论文摘要