Uncertainty Estimation for Predictive Collision Avoidance in Human-Robot Collaboration.
2023 IEEE International Conference on Robotics and Biomimetics (ROBIO)(2023)
摘要
Predictive motion planning enables robots to consider workspace obstacles and proactively avoid them. Estimating future poses of various obstacles is complex and exhibits results with uncertainties. Especially in the case of human-robot collaboration, the forecast process has to deal with sudden motion changes and varying human intentions. This paper proposes an uncertainty estimation for human motion extrapolations in the joint space of a human skeleton. The approach utilizes Gaussian Mixture Models that allow an online determination of the accuracy of current extrapolations based on preceding extrapolation errors. Multiple human model representations enable consideration of estimated uncertainties and are presented along with evaluating the resulting robot trajectories. The results show that considering estimated uncertainties leads to a robot trajectory with increased distances between humans and robots and decreased danger index from the literature, which is desirable for collaborative scenarios.
更多查看译文
AI 理解论文
溯源树
样例
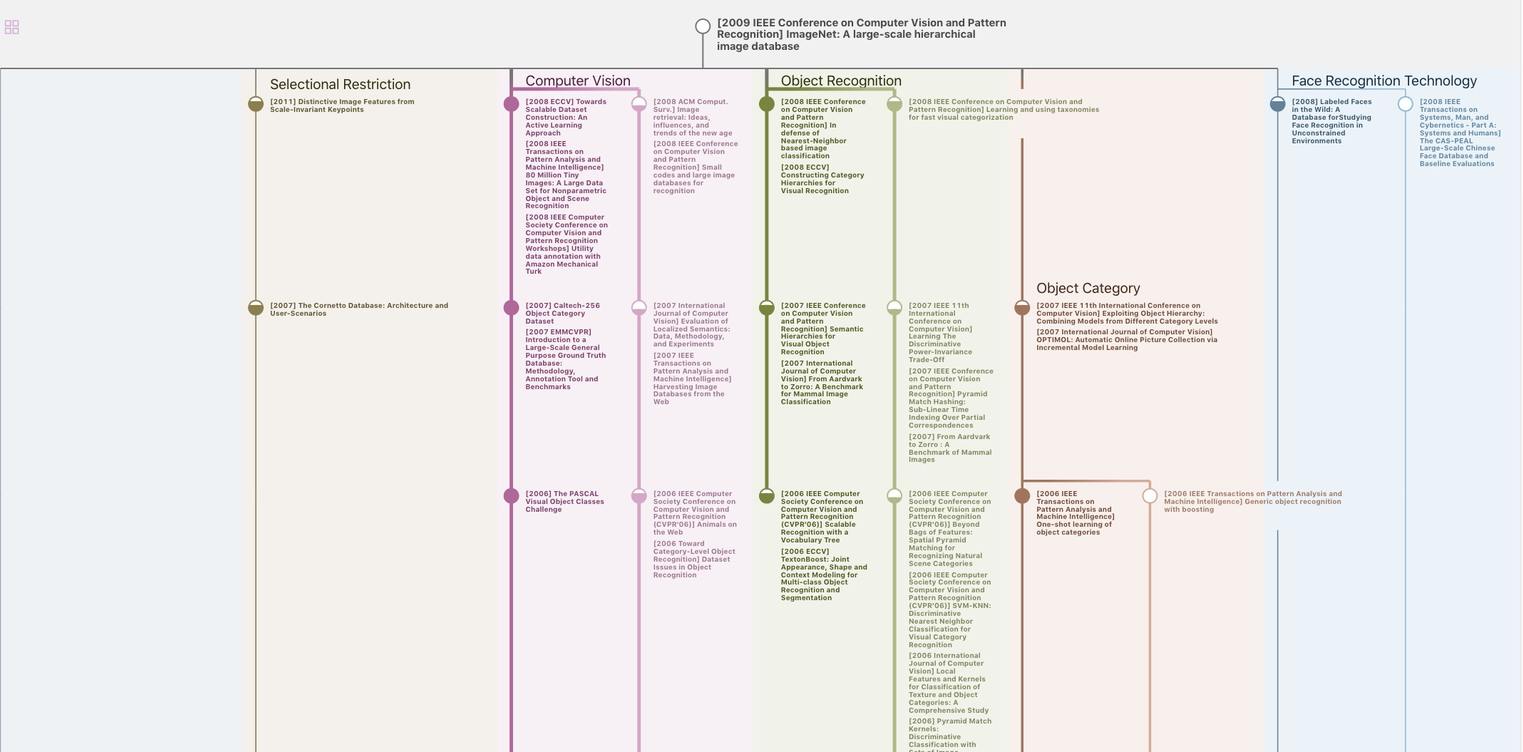
生成溯源树,研究论文发展脉络
Chat Paper
正在生成论文摘要