Combining a multi-feature neural network with multi-task learning for emergency calls severity prediction
ARRAY(2024)
摘要
In emergency call centers, operators are required to analyze and prioritize emergency situations prior to any intervention. This allows the team to deploy resources efficiently if needed, and thereby provide the optimal assistance to the victims. The automation of such an analysis remains challenging, given the unpredictable nature of the calls. Therefore, in this study, we describe our attempt in improving an emergency calls processing system's accuracy in the classification of an emergency's severity, based on transcriptions of the caller's speech. Specifically, we first extend the baseline classifier to include additional feature extractors of different modalities of data. These features include detected emotions, time-based features, and the victim's personal information. Second, we experiment with a multi-task learning approach, in which we attempt to detect the nature of the emergency on the one hand, and improve the severity classification score on the other hand. Additional improvements include the use of a larger dataset and an explainability study of the classifier's decision-making process. Our best model was able to predict 833 emergency calls' severity with a 71.27% accuracy, a 5.33% improvement over the baseline model. Moreover, we extended our tool with additional modules that can prove to be useful when handling emergency calls.
更多查看译文
关键词
Emergency calls,Natural language processing,Speech emotion recognition,Deep learning
AI 理解论文
溯源树
样例
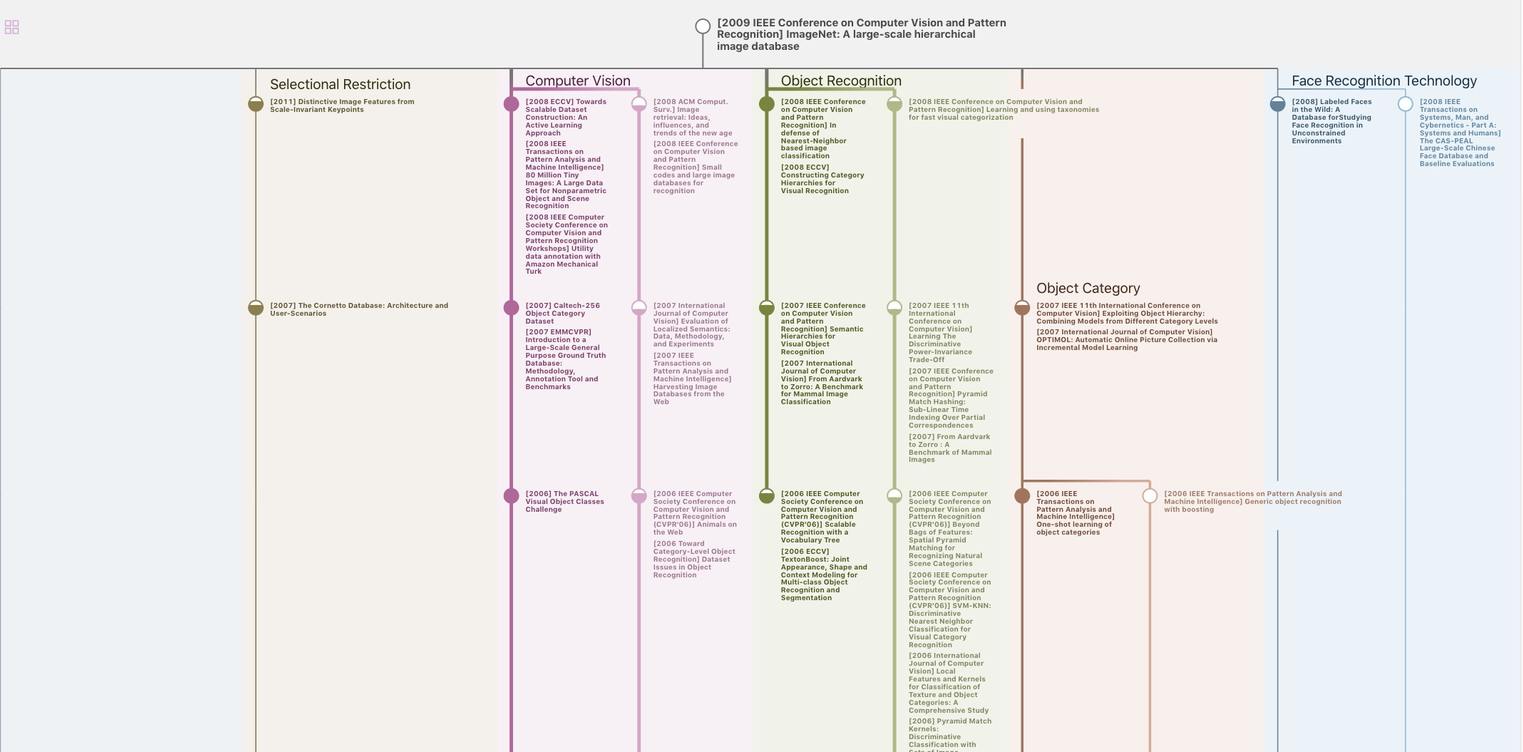
生成溯源树,研究论文发展脉络
Chat Paper
正在生成论文摘要