Wear depth estimation from single 2-D image based on shape from Shading and convolutional neural network hybrid model for in-situ wear assessment
WEAR(2024)
摘要
Vision-based wear inspection is a prevalent technique in in-situ assessment, such as borescope inspection. Evaluating wear volume of the damage has relied on Shape From Shading (SFS) algorithms to reconstruct the 3-D topography from a single 2-D image. However, this approach faces limitations in accuracy due to data insufficiency and the impact of non-Lambertian reflections on a 2-D surface image. To address this issue, a depth estimation method is proposed, centered on an SFS-Convolutional Neural Network (CNN) hybrid model. First, in terms of the insufficient information of a 2-D image, a coarse-to-fine detection network has been developed to identify the damage region. Regarding the influence of the non-Lambert reflection of the worn surface, an SFSCNN hybrid model is constructed incorporating an SFS-based guidance branch and a CNN branch based on a dual-attention mechanism. Specifically, the latter emphasizes the connection between the 2-D damage image and the depth information, disregarding non-Lambertian reflection, while the former extracts the initial depth information from the damage region for CNN learning. Consequently, the wear volume can be derived by accumulating the depth of the reconstructed 3-D worn region. To verify, several real worn surfaces have been used and examined using the proposed model and Laser Scanning Confocal Microscopy. Experimental results indicate that the proposed method can determine the depth of a worn surface from a single surface image, and the absolute relative error of the computed wear volume is less than 19 %, which satisfies the requirement of in-situ wear condition assessment.
更多查看译文
关键词
Wear volumetric analysis,Surface topography,Depth estimation,Damage detection
AI 理解论文
溯源树
样例
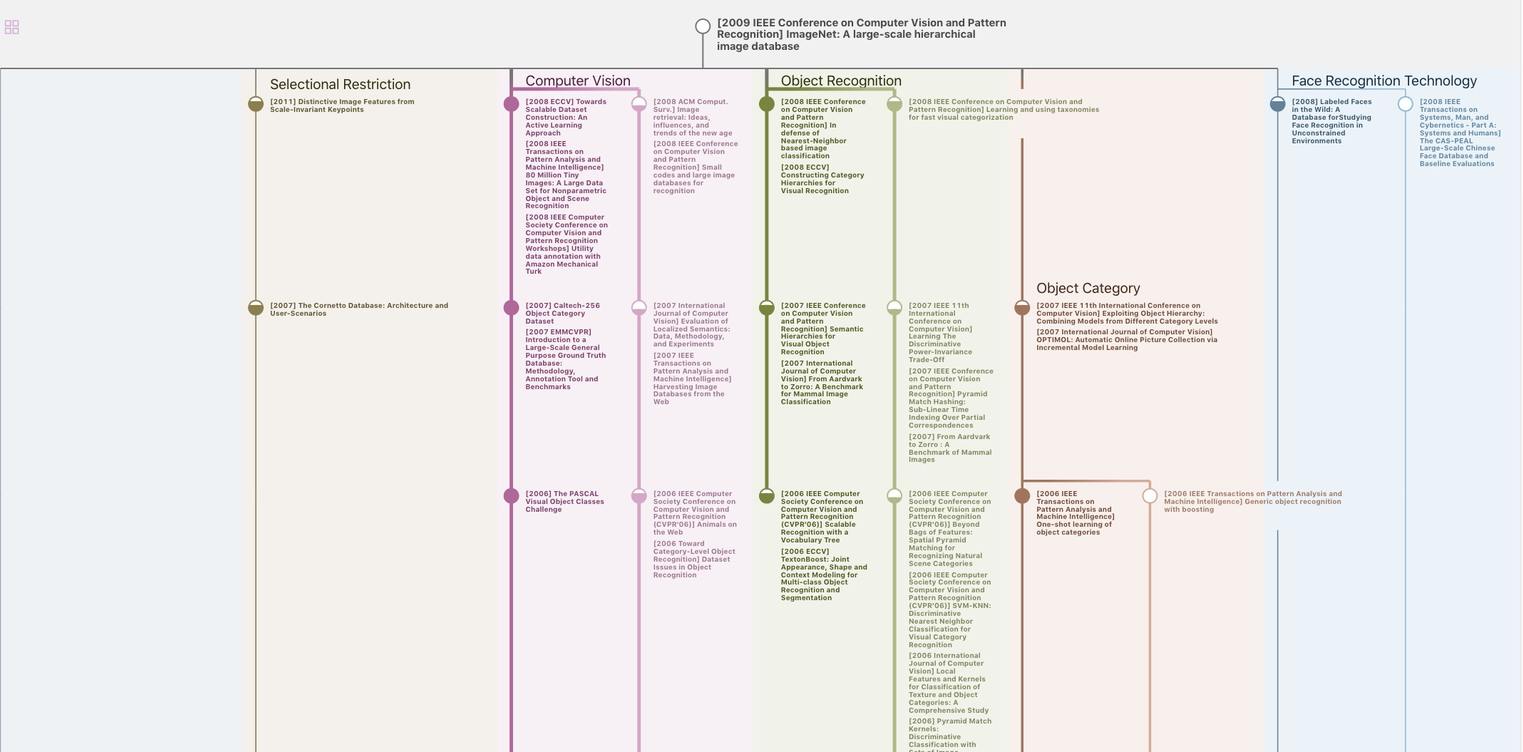
生成溯源树,研究论文发展脉络
Chat Paper
正在生成论文摘要