Pre-training with pseudo-labeling for regulatory sequence prediction
biorxiv(2024)
摘要
Predicting molecular processes using deep learning is a promising approach to provide biological insights for non-coding SNPs identified in genome-wide association studies. However, most deep learning methods rely on supervised learning which requires DNA sequences associated with functional data, and whose amount is severely limited by the finite size of the human genome. Conversely, the amount of mammalian DNA sequences is growing exponentially due to ongoing large-scale sequencing projects, but in most cases without functional data. To alleviate the limitations of supervised learning, we propose a novel semi- supervised learning based on pseudo-labeling, which allows to explot unannotated DNA sequences from numerous genomes during model pre-training. The approach is very flexible and can be used to train any neural architecture including state-of-the-art models, and shows in certain situations strong predictive performance improvements compared to standard supervised learning in most cases.
### Competing Interest Statement
The authors have declared no competing interest.
更多查看译文
AI 理解论文
溯源树
样例
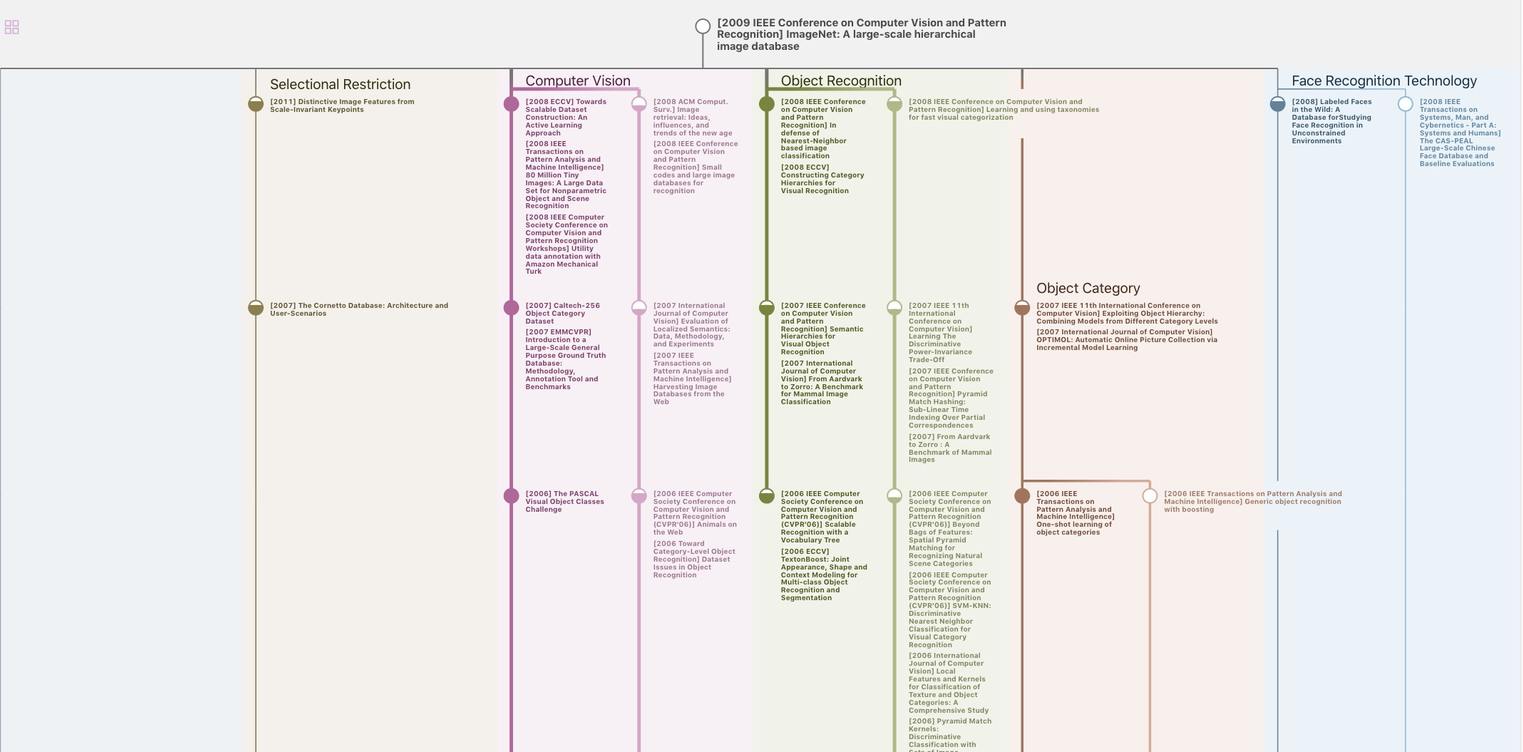
生成溯源树,研究论文发展脉络
Chat Paper
正在生成论文摘要