Machine learning-guided exploration and experimental assessment of unreported compositions in the quaternary Ti-Zr-Cu-Pd biocompatible metallic glass system
ACTA BIOMATERIALIA(2024)
摘要
Due to their outstanding elastic limit, biocompatible Ti -based bulk metallic glasses (BMGs) are candidate materials to decrease the size of medical implants and therefore reduce their invasiveness. However, the practical use of classical Ti-BMGs in medical applications is in part hindered by their high copper content: more effort is thus required to design low -copper Ti-BMGs. In this work, in line with current rise in AI -driven tools, machine learning (ML) approaches, a neural -network ML model is used to explore the glass -forming ability (GFA) of unreported low -copper compositions within the biocompatible Ti-Zr-CuPd system. Two types of models are trained and compared: one based on the alloy composition only, and a second based on various features derived from the alloying elements. Contrary to expectation, the predictive power of both models in evaluating GFA is similar. The compositional space identified by ML as promising is experimentally assessed, finding unfortunately low GFA. These results indicate that the ML approach may be premature for specific composition tuning of amorphous metallic materials. We emphasise that the development of ML tools in GFA prediction requires an improvement of the dataset, in terms of homogeneity, size and GFA descriptors, which must be supported by increased reporting of high -quality experimental GFA measurements, both positive and negative.
更多查看译文
关键词
Biomaterials,AI-Design,Bulk metallic glasses,Ti-alloys
AI 理解论文
溯源树
样例
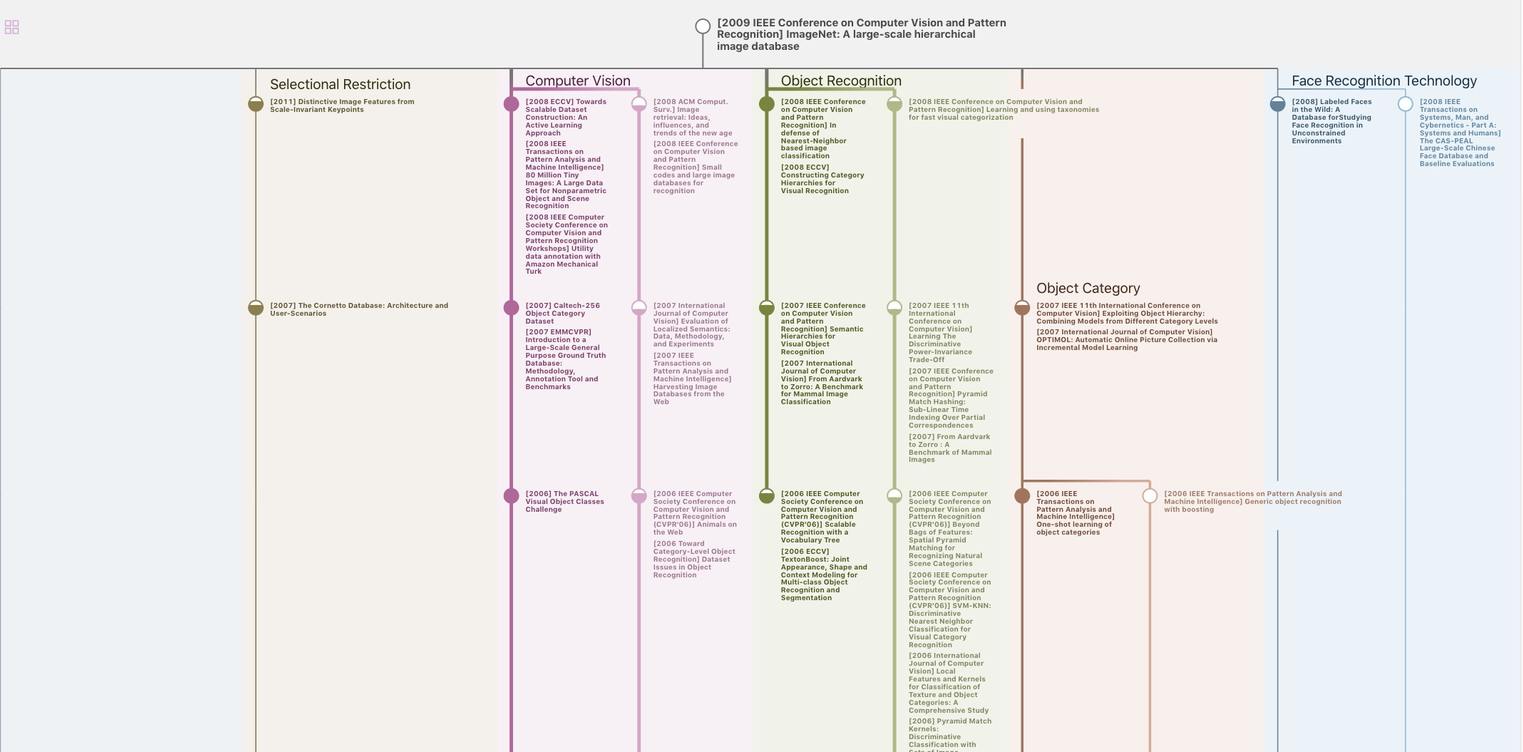
生成溯源树,研究论文发展脉络
Chat Paper
正在生成论文摘要