In-Line Detection of Clinical Mastitis by Identifying Clots in Milk Using Images and a Neural Network Approach
ANIMALS(2023)
摘要
Simple Summary The study focused on improving the detection of clinical bovine mastitis, the inflammation of the udder in cows as a response to intramammary infection, which can be identified by the presence of clots in the milk. Currently, automated milking systems do not detect this important disease very accurately. To address this, we developed a clots detection program using a neural network. This neural network was trained to recognize clots in milk samples from dairy cows by using a large number of pictures of milk filter socks, some with and some without clots. These pictures were divided into different sets for training, validating, and testing the program, respectively. The settings of the neural network were optimized using a genetic algorithm. The program's interpretations were explained using a method called integrated gradients. The program was found to be 100% accurate in identifying clots in the test pictures. This suggests that the method could be very useful for automatically checking for clinical mastitis on dairy farms, although further field validation through integration into the existing systems is needed.Abstract Automated milking systems (AMSs) already incorporate a variety of milk monitoring and sensing equipment, but the sensitivity, specificity, and positive predictive value of clinical mastitis (CM) detection remain low. A typical symptom of CM is the presence of clots in the milk during fore-stripping. The objective of this study was the development and evaluation of a deep learning model with image recognition capabilities, specifically a convolutional neural network (NN), capable of detecting such clots on pictures of the milk filter socks of the milking system, after the phase in which the first streams of milk have been discarded. In total, 696 pictures were taken with clots and 586 pictures without. These were randomly divided into 60/20/20 training, validation, and testing datasets, respectively, for the training and validation of the NN. A convolutional NN with residual connections was trained, and the hyperparameters were optimized based on the validation dataset using a genetic algorithm. The integrated gradients were calculated to explain the interpretation of the NN. The accuracy of the NN on the testing dataset was 100%. The integrated gradients showed that the NN identified the clots. Further field validation through integration into AMS is necessary, but the proposed deep learning method is very promising for the inline detection of CM on AMS farms.
更多查看译文
关键词
deep learning,clinical mastitis,automated milking systems,image recognition,clots in milk
AI 理解论文
溯源树
样例
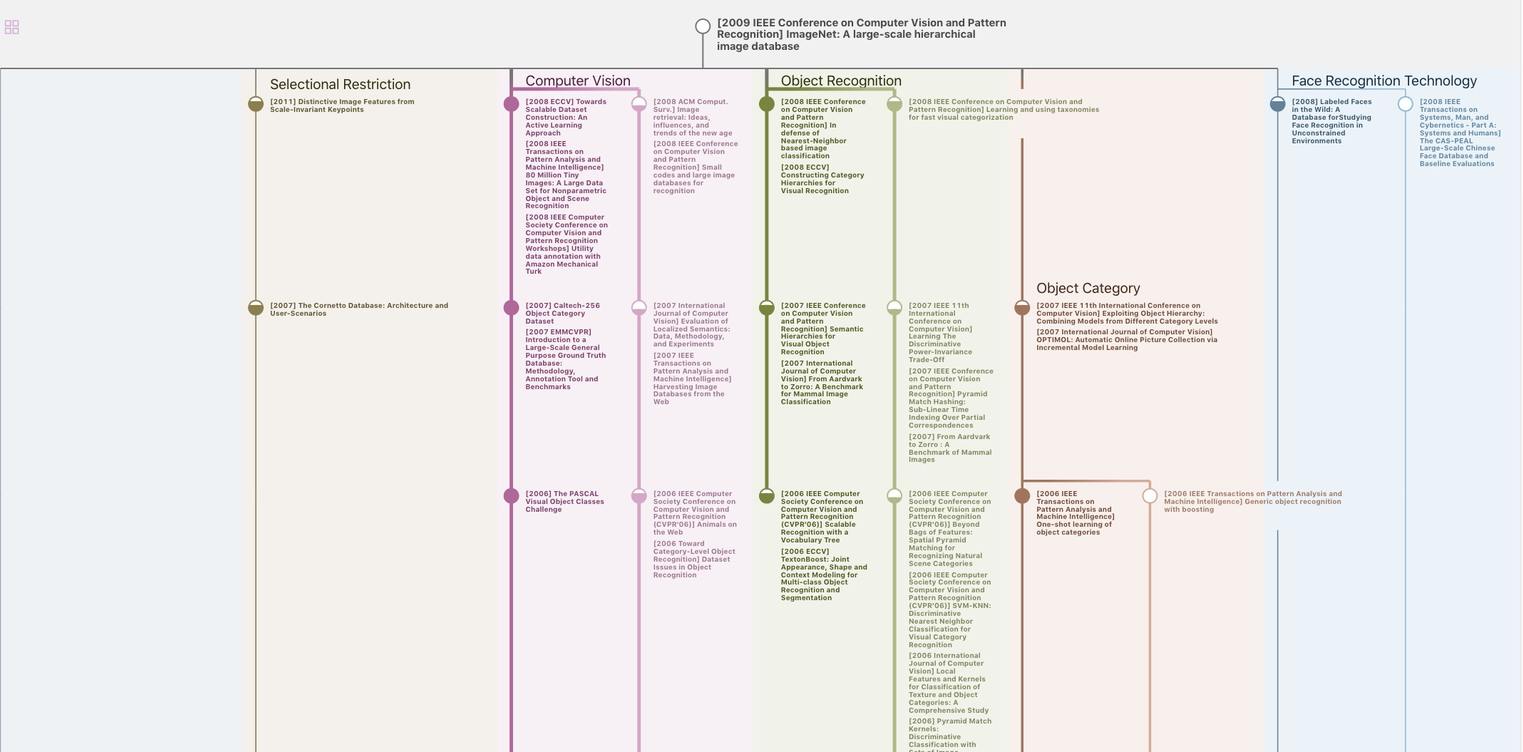
生成溯源树,研究论文发展脉络
Chat Paper
正在生成论文摘要