Robust augmented estimation for hourly PM _2.5 using heteroscedastic spatiotemporal models
Stochastic Environmental Research and Risk Assessment(2024)
摘要
We propose an adjusted robust heteroscedastic autoregressive spatiotemporal model with a data-driven process to predict the hourly PM _2.5 concentrations in Xi’an and Xianyang, China. To begin with, an adjusted variance function for the heteroscedastic model is proposed to capture the different variances of the PM _2.5 concentrations during the periods of heating and non-heating. Compared to the traditional heteroscedastic method, the proposed adjusted heteroscedastic method has lower standard errors for most of the coefficient estimators. Secondly, to improve the prediction accuracy, the temporal correlations of the PM _2.5 data are incorporated into the adjusted heteroscedastic model by augmenting some additional predictors generated from an autoregressive process. The prediction results of the testing data demonstrate that the adjusted robust heteroscedastic autoregressive model significantly improves the prediction. Finally, utilizing the spatiotemporal simple kriging method, the spatial correlations of PM _2.5 concentrations at different air monitoring stations are incorporated into the prediction by adding the weighted robust residuals to the previous prediction. The prediction performance of the final proposed forecasting method is shown to be further enhanced.
更多查看译文
关键词
Heteroscedasticity,Outliers,PM _2.5,Robust estimation,Spatiotemporal correlation
AI 理解论文
溯源树
样例
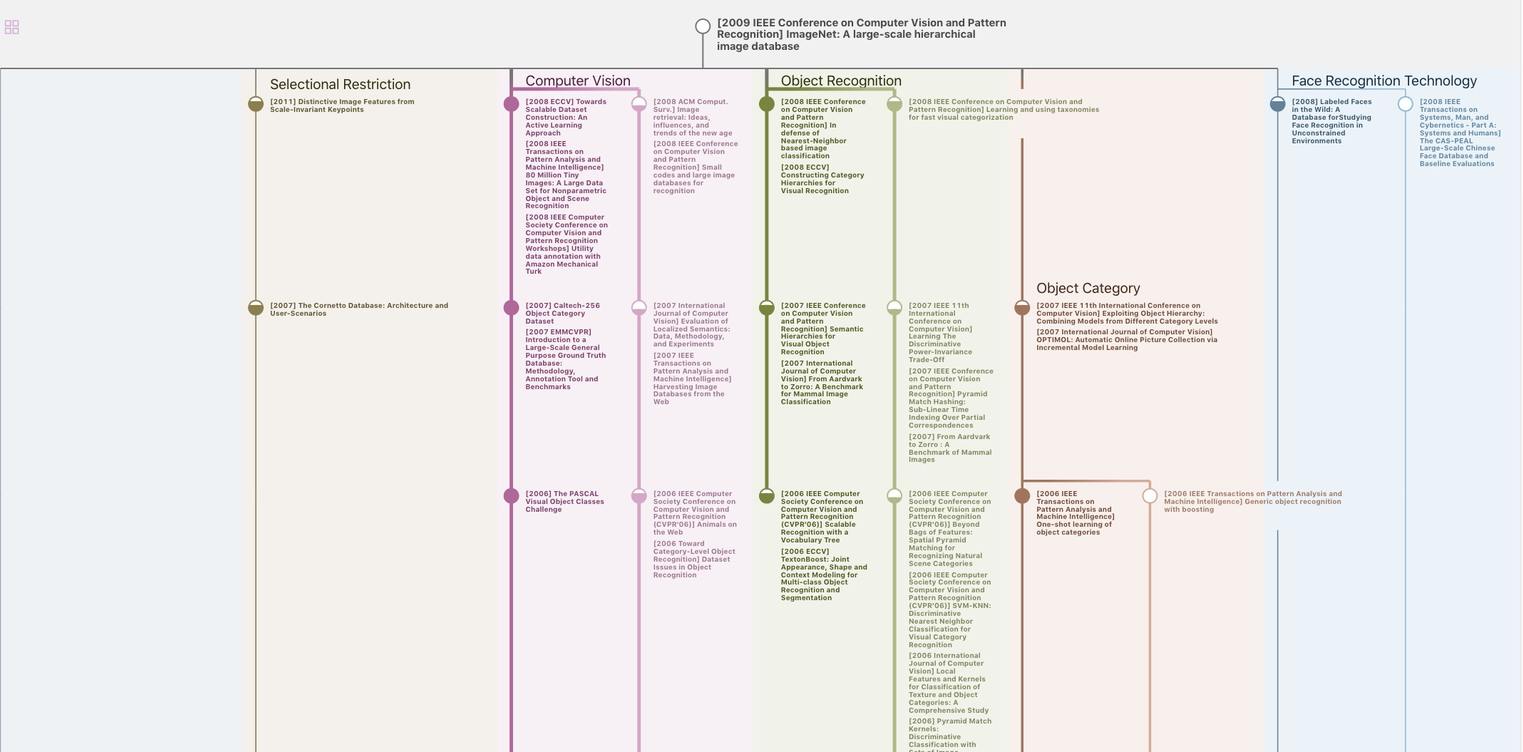
生成溯源树,研究论文发展脉络
Chat Paper
正在生成论文摘要