RGGC-UNet: Accurate Deep Learning Framework for Signet Ring Cell Semantic Segmentation in Pathological Images
BIOENGINEERING-BASEL(2024)
摘要
Semantic segmentation of Signet Ring Cells (SRC) plays a pivotal role in the diagnosis of SRC carcinoma based on pathological images. Deep learning-based methods have demonstrated significant promise in computer-aided diagnosis over the past decade. However, many existing approaches rely heavily on stacking layers, leading to repetitive computational tasks and unnecessarily large neural networks. Moreover, the lack of available ground truth data for SRCs hampers the advancement of segmentation techniques for these cells. In response, this paper introduces an efficient and accurate deep learning framework (RGGC-UNet), which is a UNet framework including our proposed residual ghost block with ghost coordinate attention, featuring an encoder-decoder structure tailored for the semantic segmentation of SRCs. We designed a novel encoder using the residual ghost block with proposed ghost coordinate attention. Benefiting from the utilization of ghost block and ghost coordinate attention in the encoder, the computational overhead of our model is effectively minimized. For practical application in pathological diagnosis, we have enriched the DigestPath 2019 dataset with fully annotated mask labels of SRCs. Experimental outcomes underscore that our proposed model significantly surpasses other leading-edge models in segmentation accuracy while ensuring computational efficiency.
更多查看译文
关键词
semantic segmentation,signet ring cell,residual ghost block,ghost coordinate attention
AI 理解论文
溯源树
样例
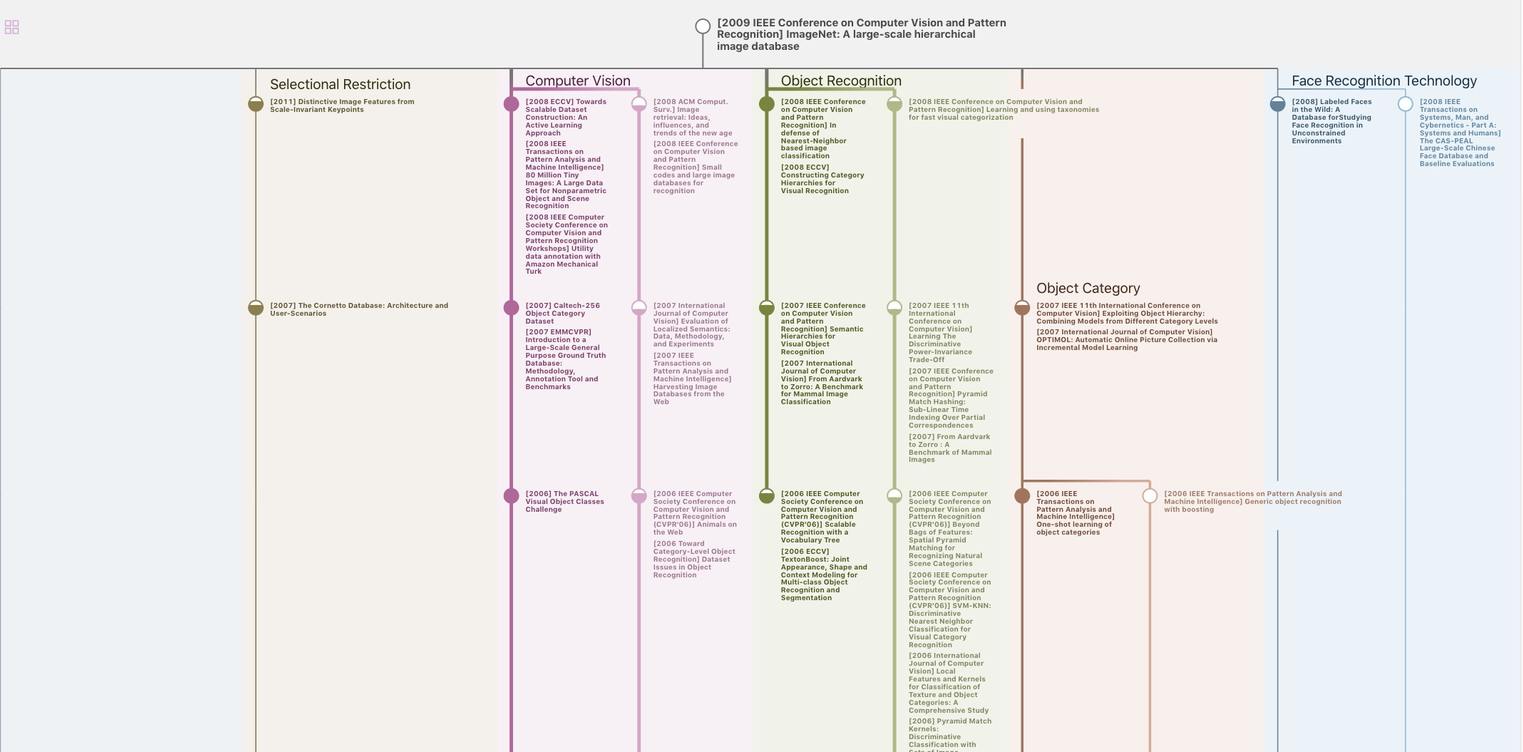
生成溯源树,研究论文发展脉络
Chat Paper
正在生成论文摘要