Reducing the Computational Cost of Transformers for Person Re-identification.
2023 IEEE International Conference on Robotics and Biomimetics (ROBIO)(2023)
摘要
Transformer-based visual technologies have witnessed remarkable progress in recent years, and person re-identification (ReID) is one of the active research areas that adopts transformers to improve the performance. However, a major challenge of applying transformers to ReID is the high computational cost, which hinders the real-time deployment of such methods. To address this issue, this paper proposes two simple yet effective techniques to reduce the computation of transformers for ReID. The first technique is to eliminate the invalid patches that do not contain any person information, thereby reducing the number of tokens fed into the transformer. Considering that computational complexity is quadratic with respect to input tokens, the second technique partitions the image into multiple windows, applies separate transformers to each window, and merges class tokens from each window, which can reduce the complexity of the self-attention mechanism. By combining these two techniques, our proposed method reduces the SOTA baseline model by 12.2% FLOPs, while slightly improving the rank-1 accuracy and only sacrificing 1.1% mAP on DukeMTMC-ReID dataset.
更多查看译文
AI 理解论文
溯源树
样例
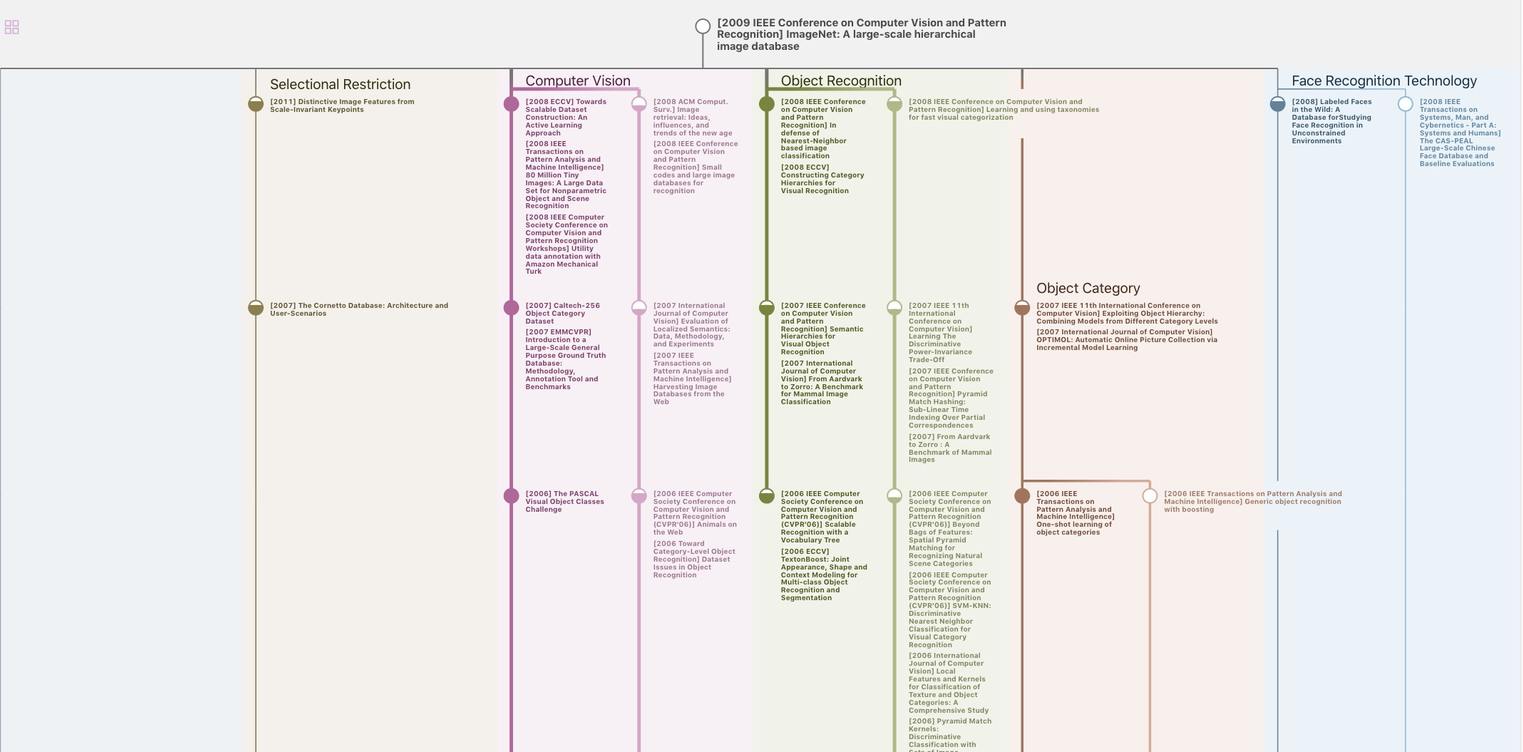
生成溯源树,研究论文发展脉络
Chat Paper
正在生成论文摘要