Feedforward Enhanced Reinforcement Learning Control for a Small Humanoid Robot KHR-3HV * .
2023 IEEE International Conference on Robotics and Biomimetics (ROBIO)(2023)
摘要
The humanoid gait generation task of biped robots has been a lasting challenge. To achieve a stable gait in such a complex kinematic system, with the improvement of computing power and algorithms updates, machine learning methods such as reinforcement learning (RL) are widely applied to this topic and made progressive results. RL provides a convenient end-to-end solution and has become the mainstream machine learning method in the field of robotics. However, there are still problems when using traditional RL methods in aspects of similarity to human natural gait, training time, robustness to perturbances, and generalization ability of other tasks. In this paper, we propose an improved framework based on the feedforward enhanced reinforcement learning (FERL) algorithm to efficiently generate a humanoid gait for a small humanoid robot KRH-3HV. In FERL, the control action of biped robots consists of two parts: the reference part and the weighted RL part. In this paper, we introduced prior knowledge that human walking gait exhibits sinusoidal characteristics and designed reference actions by inverse kinematic analysis based on this principle. The weighted RL part is independently set to motivate the biped robot to complete the target task. Providing a reference control signal sequence helps to generate an ideal gait and the action space of the weighted RL is decreased compared with traditional RL so that the training time is reduced while still maintaining certain robustness. By setting up 4 groups of control experiments in a simulation environment, we efficiently generated an effective humanoid gait to complete the target tasks of walking on flat ground and climbing stairs, and proved that the proposed method works well in gait biomimetic similarity, training efficiency, robustness, and generalization ability to other tasks by simple reward function designing.
更多查看译文
AI 理解论文
溯源树
样例
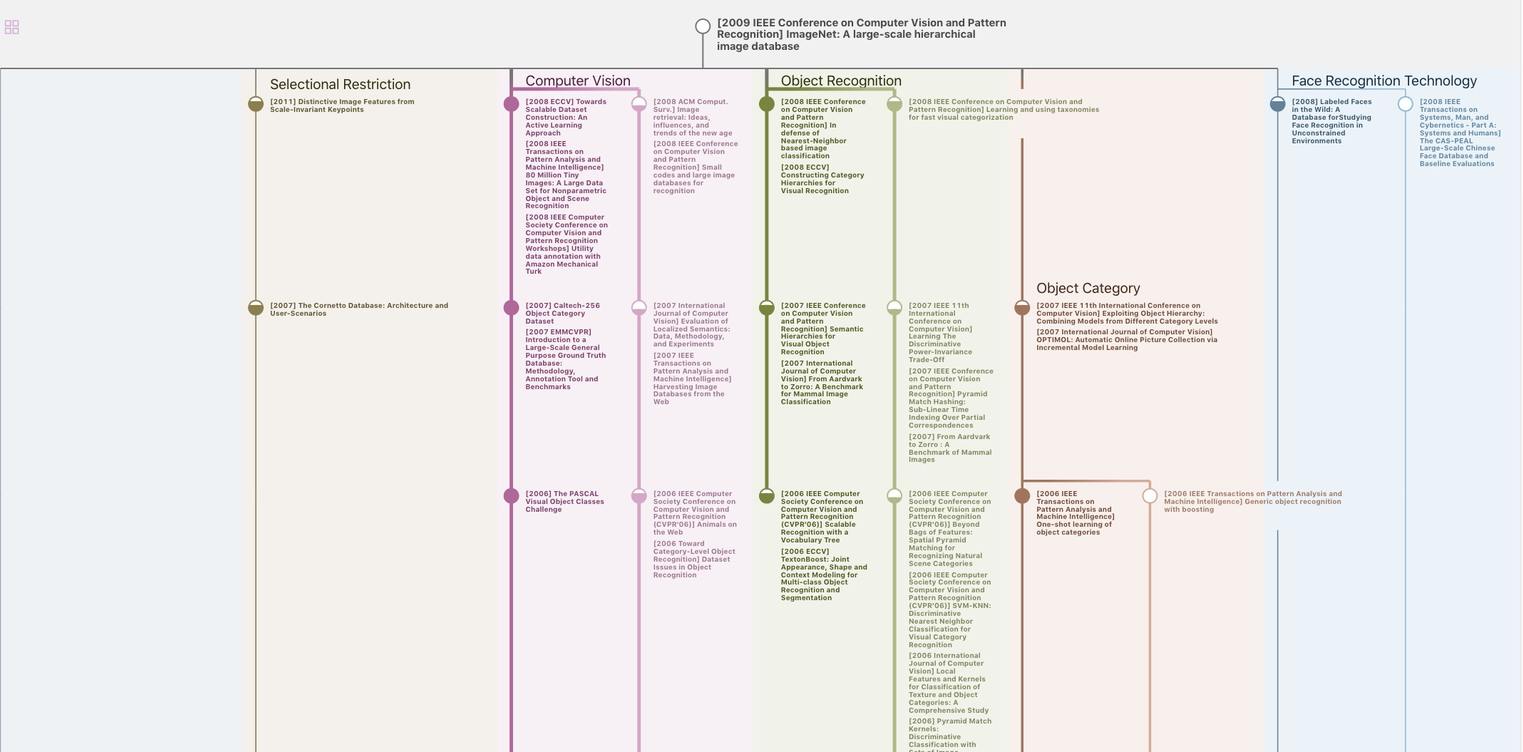
生成溯源树,研究论文发展脉络
Chat Paper
正在生成论文摘要