An Effective Representation Learning Approach: The Integrated Self-Supervised Pre-Training Models of StyleGAN2-ADA and DINO for Colon Polyp Images
IEEE ACCESS(2023)
摘要
In order to achieve optimal performance in the task of visual representation learning from image or video datasets, a significant quantity of annotated data is required. However, the process of collecting and annotating large-scale datasets is both costly and time-consuming, particularly in domains such as medicine where access to patient images is limited due to privacy concerns and the difficulty of accurately annotating images without expert assistance. One potential solution to this challenge is the utilization of both Self-Supervised Learning (SSL) methods and Generative Adversarial Networks (GANs). These fields have seen rapid advancement in recent years, with GANs possessing the unique ability to generate unlabelled data sources containing highly realistic images, while SSL methods can extract general features from large-scale data without the need for human-annotated labels. In this study, we investigate the use of the recently introduced StyleGAN2-ADA and Self-Supervised Pre-Training of DINO for the pretext task, and propose an integrated approach that combines these methods with Transfer Learning (TL) in order to improve representation learning on polyp datasets in the medical domain.
更多查看译文
关键词
Task analysis,Feature extraction,Training,Medical diagnostic imaging,Transfer learning,Representation learning,Generative adversarial networks,polyp,self-supervised learning,transfer learning
AI 理解论文
溯源树
样例
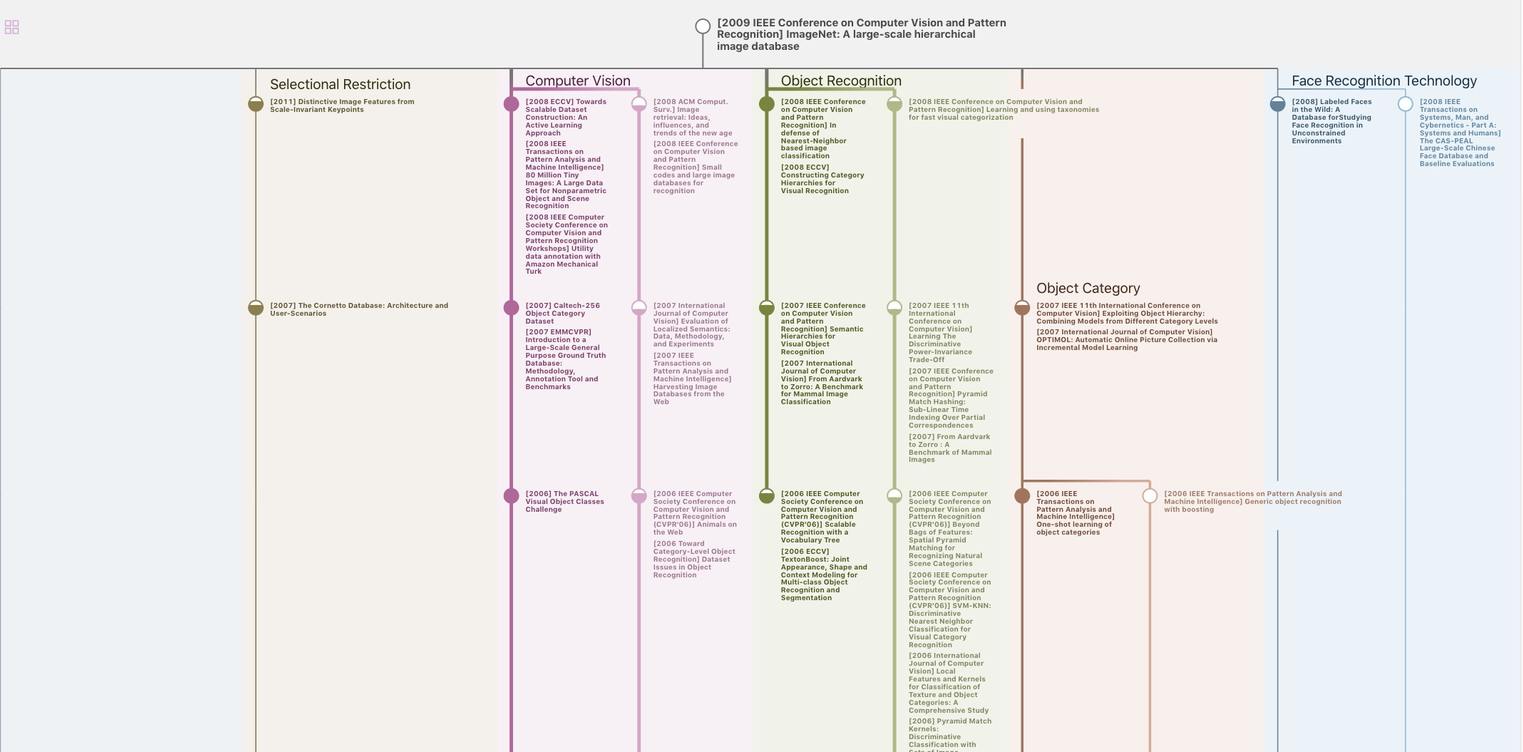
生成溯源树,研究论文发展脉络
Chat Paper
正在生成论文摘要