Small CNN for Plant Disease Classification Using Quantization-Aware Training: A Case Study on MobileNetV3
2023 International Conference on Radar, Antenna, Microwave, Electronics, and Telecommunications (ICRAMET)(2023)
摘要
One of the most significant issues that frequently arises in farming is plant diseases. Therefore, it is very important to detect and classify plant diseases accurately and quickly. Developments in artificial intelligence (AI) research have made it possible to automatically detect plant diseases, with one of these methods being convolutional neural networks (CNNs). However, the sizes of many CNN models are not suitable for the agricultural sector. For this reason, the symbolizing technique of small and accurate replicated neural networks becomes crucial when applied to embedded systems. In this study, we investigated the application of Quantization-Aware Training (QAT) techniques to the MobileNetV3 model, which aims to improve efficiency by compressing the model and reducing runtime without decreasing accuracy in the classification of plant diseases, especially in the corn corp. Models quantized with QAT have smaller sizes and lighter computing requirements. Thus, the implementation of QAT on MobileNetV3 will be an innovation that enables the optimization of models with limited resources. Our experiments prove that QAT makes MobileNetV3 as the smallest model (4.3 MB) compared to other models that without QAT, and achieve the fastest running time (637.9 s) without a reduction in accuracy (94.86).
更多查看译文
关键词
Plant diseases,MobileNet,Quantization Aware Training,Small CNN,Disease Classification
AI 理解论文
溯源树
样例
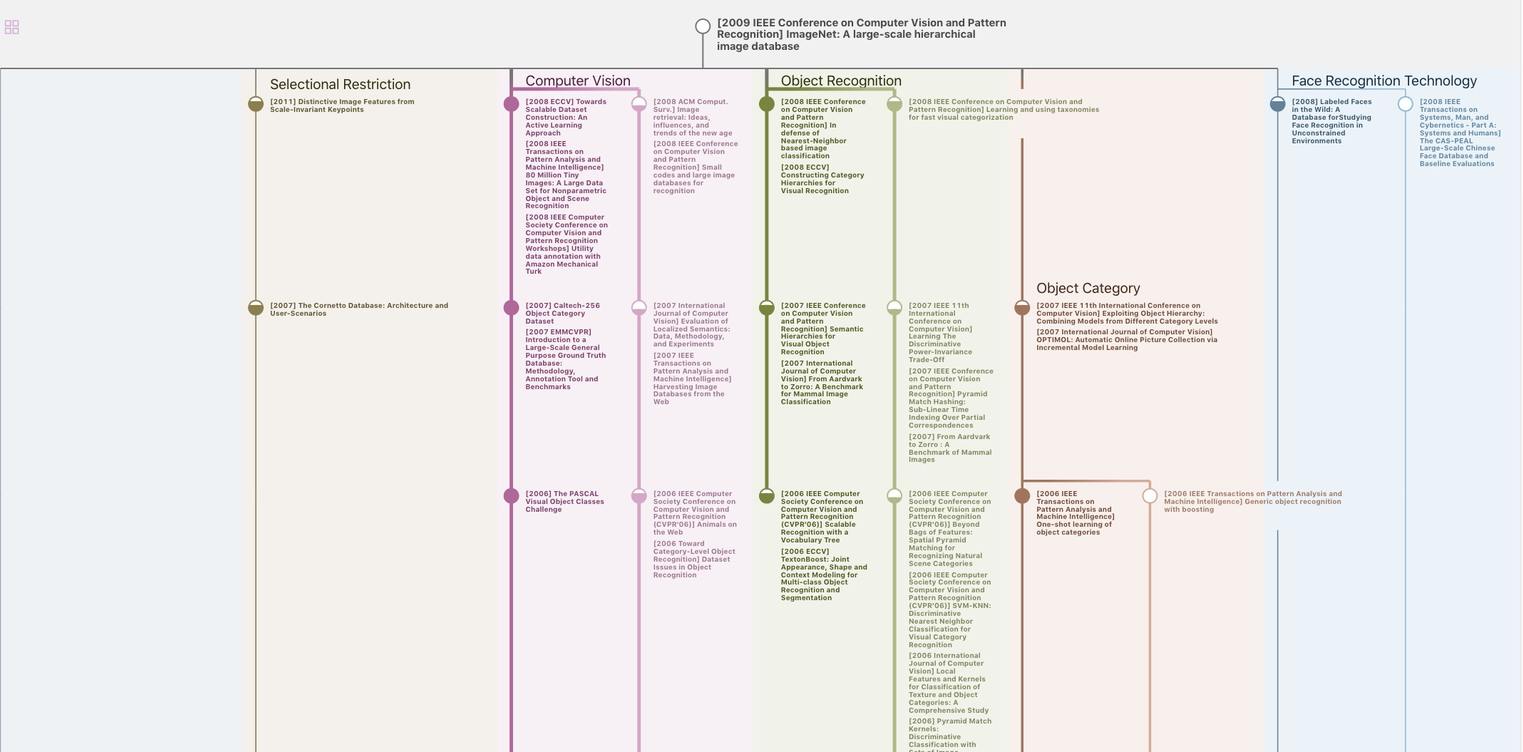
生成溯源树,研究论文发展脉络
Chat Paper
正在生成论文摘要