Multiple Spatial-Spectral Features Aggregated Neural Network for Hyperspectral Change Detection
IEEE GEOSCIENCE AND REMOTE SENSING LETTERS(2024)
摘要
Recently, convolution neural networks (CNNs) have flourished in hyperspectral image (HSI) change detection (CD). However, these approaches typically rely on single-scale and single-level features to obtain change information, limiting the further boost detection accuracy. To solve the above problem, we propose a novel multiscale and multilevel spatial-spectral features aggregated neural network (M(2)S(2)Net) for HSI CD. The proposed method leverages the multiple spatial-spectral (SS) features of bi-temporal images to mine temporal change information. First, the HSIs are cropped into patches with different spatial and spectral sizes to provide various scales SS information and alleviate the problem of spectral redundancy. Then, the patches are utilized to capture the multiscale SS features in the residual connection block (ResBlock). These bi-temporal features are inputted into the Transformer encoder-based feature fusion module to learn the discriminative change representations. Via the global receive field of the self-attention mechanism, various fine-grained change information is learned from the features at different scales. Finally, the change features of each level are aggregated to produce the change map. The experiments on two public HSI datasets verify the effectiveness of the proposed method. Specifically, the overall accuracy (OA) of the M(2)S(2)Net surpasses the second-best method by 0.56% and 0.10% on the Farmland and River datasets, respectively.
更多查看译文
关键词
Attention mechanism,change detection (CD),hyperspectral image (HSI),multiscale features
AI 理解论文
溯源树
样例
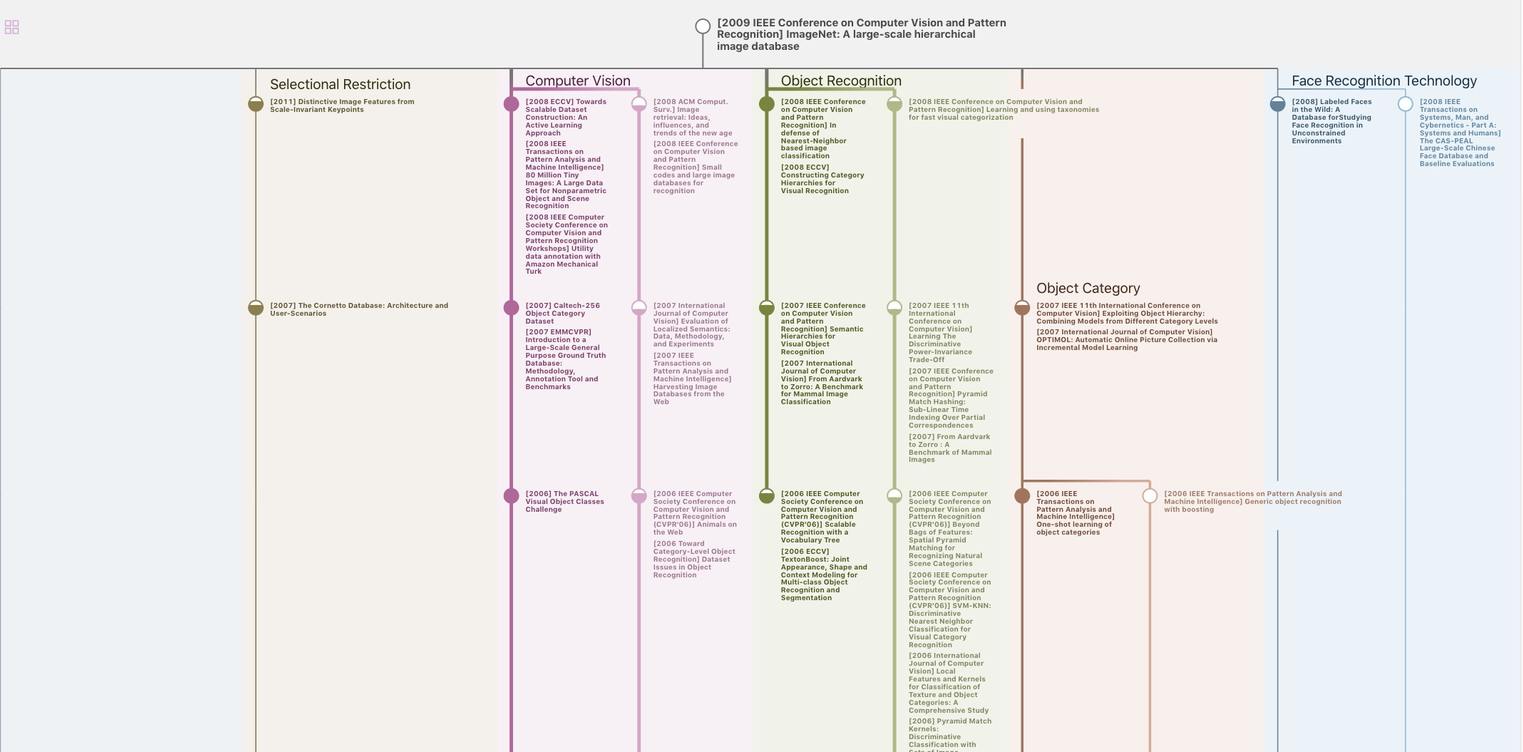
生成溯源树,研究论文发展脉络
Chat Paper
正在生成论文摘要