Detection of Fusarium Damaged Kernels in Wheat Using Deep Semi-Supervised Learning on a Novel WheatSeedBelt Dataset
2023 IEEE/CVF INTERNATIONAL CONFERENCE ON COMPUTER VISION WORKSHOPS, ICCVW(2023)
摘要
Fusarium head blight, caused by Fusarium spp., is a destructive disease of wheat worldwide. Fusarium damaged kernels (FDKs) significantly reduce grain yield and quality. Thus, FDK detection is a priority for wheat breeders seeking to develop high-grain quality and FDK-resistant wheat cultivars. However, traditional FDK measurement methods are time-consuming, labor-intensive, and of variable accuracy. Image-based phenotyping methods have the potential to efficiently detect FDK, but are challenging to develop due to the lack of large-scale damage-annotated wheat kernel datasets. Addressing this issue, we introduced Wheat-SeedBelt, a high-resolution large-scale dataset including 40, 420 close-up top- and side-view single-kernel images of 268 wheat varieties with kernel damage annotations. Utilizing this dataset, we developed an image-processing pipeline to efficiently process images and extract the representative features for machine and deep-learning purposes. We also conducted three experiments on the dataset using pretraining and semi-supervised fine-tuning phases to classify wheat kernels into healthy, unhealthy but non-FDK, and FDK affected. Our best models achieved an F1-score of 84.29% for the Healthy-Unhealthy (including FDKs) task, 56.35% for the binary FDK-nonFDK, and 68.30% for the 3-class task (Healthy, Unhealthy, and FDK). We also conducted an inter-rater reliability study, which indicated that human experts do not outperform our model in FDK prediction, providing evidence that visual classification of FDK from RGB images is a challenging task.
更多查看译文
AI 理解论文
溯源树
样例
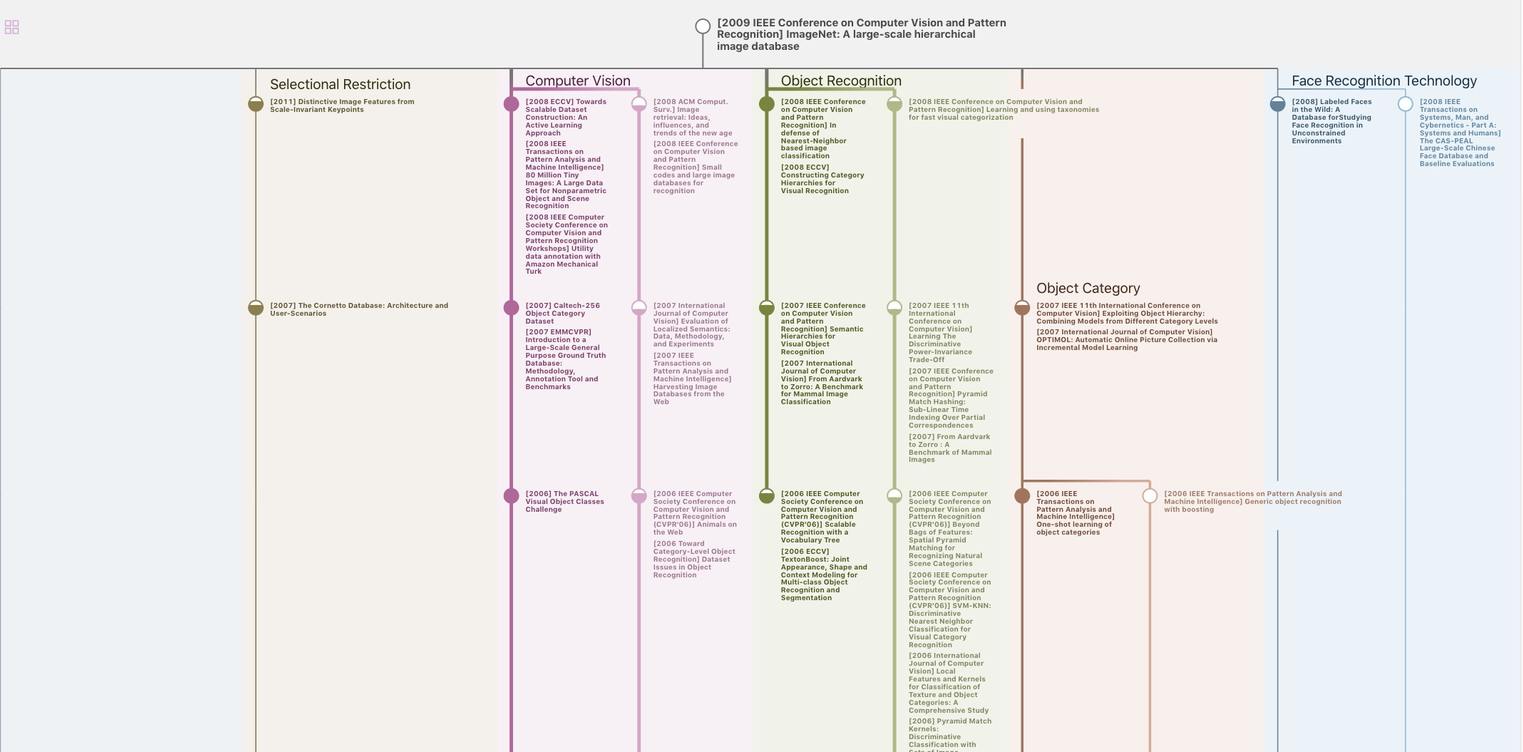
生成溯源树,研究论文发展脉络
Chat Paper
正在生成论文摘要