DeepMPR: Enhancing Opportunistic Routing in Wireless Networks via Multi-Agent Deep Reinforcement Learning
MILCOM 2023 - 2023 IEEE MILITARY COMMUNICATIONS CONFERENCE(2023)
摘要
Opportunistic routing exploits the broadcast nature of the wireless medium. It can bring higher reliability and robustness in highly dynamic and/or severe environments such as mobile or vehicular ad-hoc networks (MANETs/VANETs) but at the cost of higher redundancy. To reduce the cost of broadcast, multicast routing schemes use the connected dominating set (CDS) or multi-point relaying (MPR) set to decrease the network overhead and hence, their selection algorithms are critical. Common MPR selection algorithms are heuristic, rely on coordination between nodes, need high computational power for large networks, and are difficult to optimally tune for highly dynamic wireless conditions. In this paper, we use multi-agent deep reinforcement learning to design a novel MPR multicast routing technique, DeepMPR, which significantly outperforms the OLSR MPR selection algorithm while eliminating MPR announcement messages. Simulation results demonstrate the significantly increased performance gain compared to other popular MPR techniques over a wide range of conditions.
更多查看译文
AI 理解论文
溯源树
样例
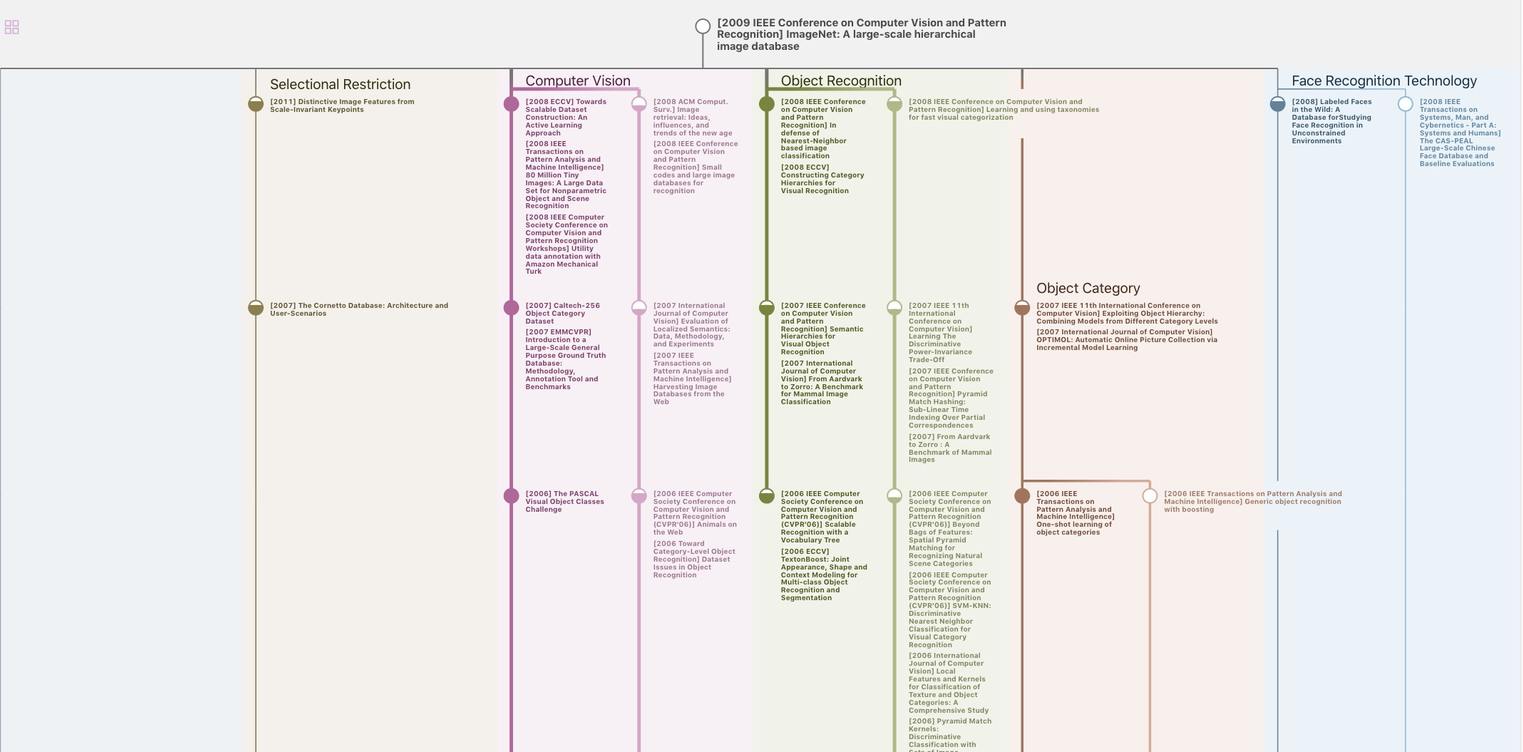
生成溯源树,研究论文发展脉络
Chat Paper
正在生成论文摘要