ZO-AdaMU Optimizer: Adapting Perturbation by the Momentum and Uncertainty in Zeroth-Order Optimization
AAAI 2024(2024)
摘要
Lowering the memory requirement in full-parameter training on large models has become a hot research area. MeZO fine-tunes the large language models (LLMs) by just forward passes in a zeroth-order SGD optimizer (ZO-SGD), demonstrating excellent performance with the same GPU memory usage as inference. However, the simulated perturbation stochastic approximation for gradient estimate in MeZO leads to severe oscillations and incurs a substantial time overhead. Moreover, without momentum regularization, MeZO shows severe over-fitting problems. Lastly, the perturbation-irrelevant momentum on ZO-SGD does not improve the convergence rate. This study proposes ZO-AdaMU to resolve the above problems by adapting the simulated perturbation with momentum in its stochastic approximation. Unlike existing adaptive momentum methods, we relocate momentum on simulated perturbation in stochastic gradient approximation. Our convergence analysis and experiments prove this is a better way to improve convergence stability and rate in ZO-SGD. Extensive experiments demonstrate that ZO-AdaMU yields better generalization for LLMs fine-tuning across various NLP tasks than MeZO and its momentum variants.
更多查看译文
关键词
NLP: Learning & Optimization for NLP,ML: Optimization,NLP: (Large) Language Models,NLP: Applications
AI 理解论文
溯源树
样例
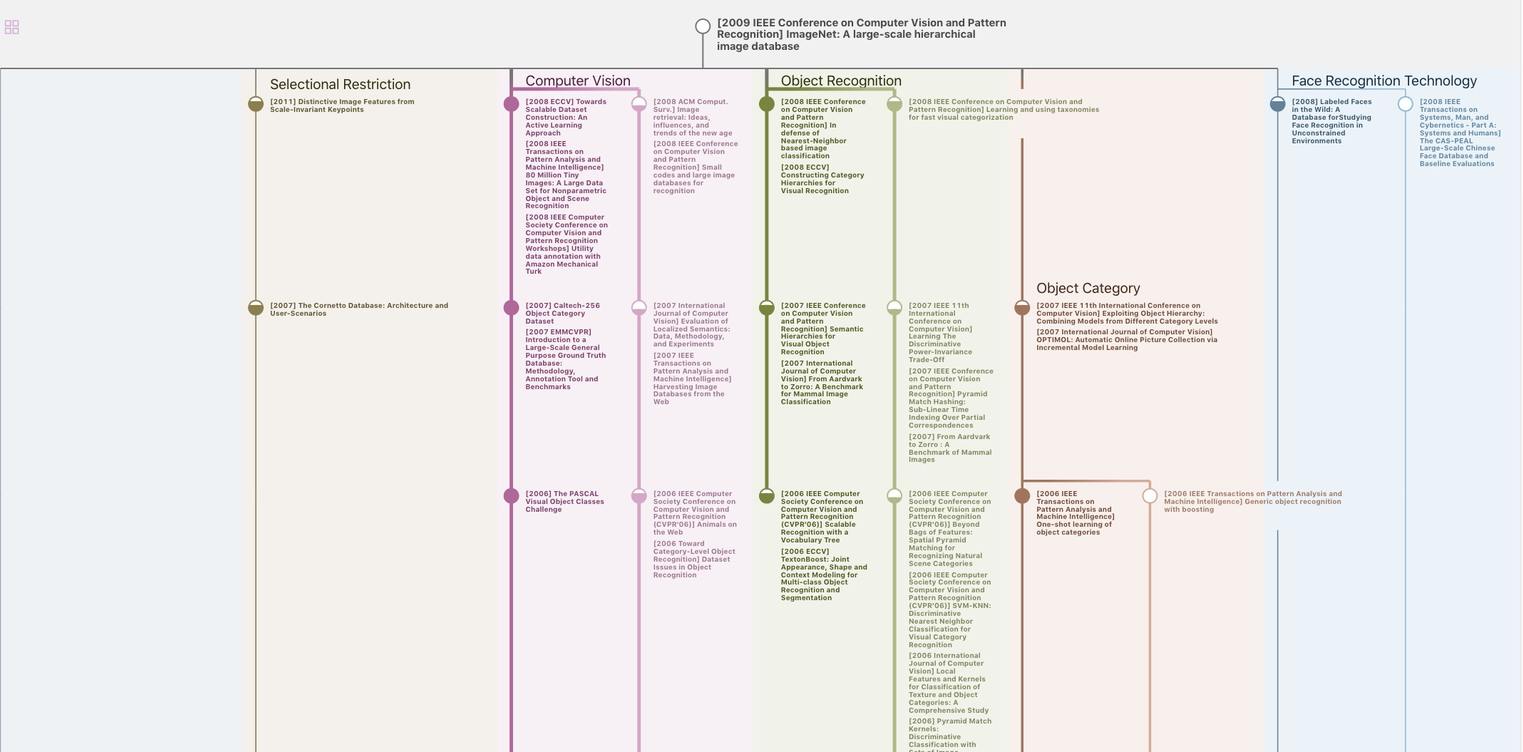
生成溯源树,研究论文发展脉络
Chat Paper
正在生成论文摘要