Explainable machine learning model based on clinical factors for predicting the disappearance of indeterminate pulmonary nodules
COMPUTERS IN BIOLOGY AND MEDICINE(2024)
摘要
Background: During lung cancer screening, indeterminate pulmonary nodules (IPNs) are a frequent finding. We aim to predict whether IPNs are resolving or non-resolving to reduce follow-up examinations, using machine learning (ML) models. We incorporated dedicated techniques to enhance prediction explainability.Methods: In total, 724 IPNs (size 50-500 mm3, 575 participants) from the Dutch-Belgian Randomized Lung Cancer Screening Trial were used. We implemented six ML models and 14 factors to predict nodule disappearance. Random search was applied to determine the optimal hyperparameters on the training set (579 nodules). ML models were trained using 5-fold cross-validation and tested on the test set (145 nodules). Model predictions were evaluated by utilizing the recall, precision, F1 score, and the area under the receiver operating characteristic curve (AUC). The best-performing model was used for three feature importance techniques: mean decrease in impurity (MDI), permutation feature importance (PFI), and SHAPley Additive exPlanations (SHAP). Results: The random forest model outperformed the other ML models with an AUC of 0.865. This model achieved a recall of 0.646, a precision of 0.816, and an F1 score of 0.721. The evaluation of feature importance achieved consistent ranking across all three methods for the most crucial factors. The MDI, PFI, and SHAP methods highlighted volume, maximum diameter, and minimum diameter as the top three factors. However, the remaining factors revealed discrepant ranking across methods.Conclusion: ML models effectively predict IPN disappearance using participant demographics and nodule characteristics. Explainable techniques can assist clinicians in developing understandable preliminary assessments.
更多查看译文
关键词
Indeterminate pulmonary nodule,Clinical factor,Explainable machine learning,Feature importance,Visualization
AI 理解论文
溯源树
样例
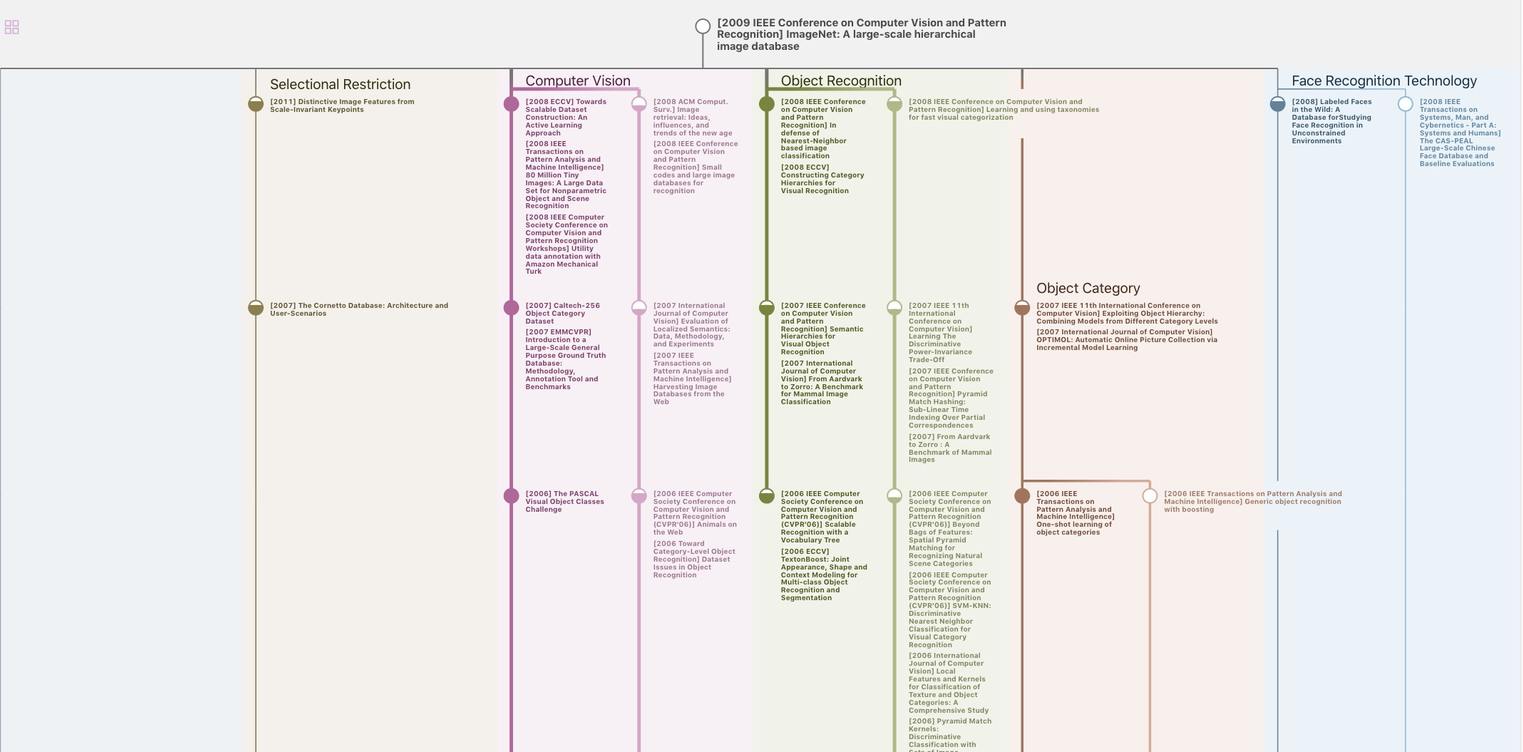
生成溯源树,研究论文发展脉络
Chat Paper
正在生成论文摘要