Deep Occupational Representations Based on Career Mobility.
DTPI(2023)
摘要
To gain a comprehensive understanding of the complexity of occupations and their interconnections, the integration of information technology with occupational studies is essential. This necessitates the mapping of conceptualized occupations into a digital space. In the study, we model the complex connections between occupations by a career mobility network, and learn vector representations of occupations using network embedding. These representations are finally used to construct a digital occupational space. Specifically, we propose a network embedding model to represent nodes in the career mobility network. The model emphasizes occupation connections by defining path commonalities to preserve the main patterns of career mobility. Then, we combine random walks and deep learning to learn these patterns. The model can capture both network structure and occupational characteristics at the same time. We demonstrate the effectiveness of the proposed network embedding model in node classification and link prediction using real-world data, as well as provide evidence for the validity of the occupational space. Taken together, our work presents a feasible way to use advanced computing technology to learn task-independent occupational representations.
更多查看译文
关键词
career mobility,complex network,occupational representations,network embedding
AI 理解论文
溯源树
样例
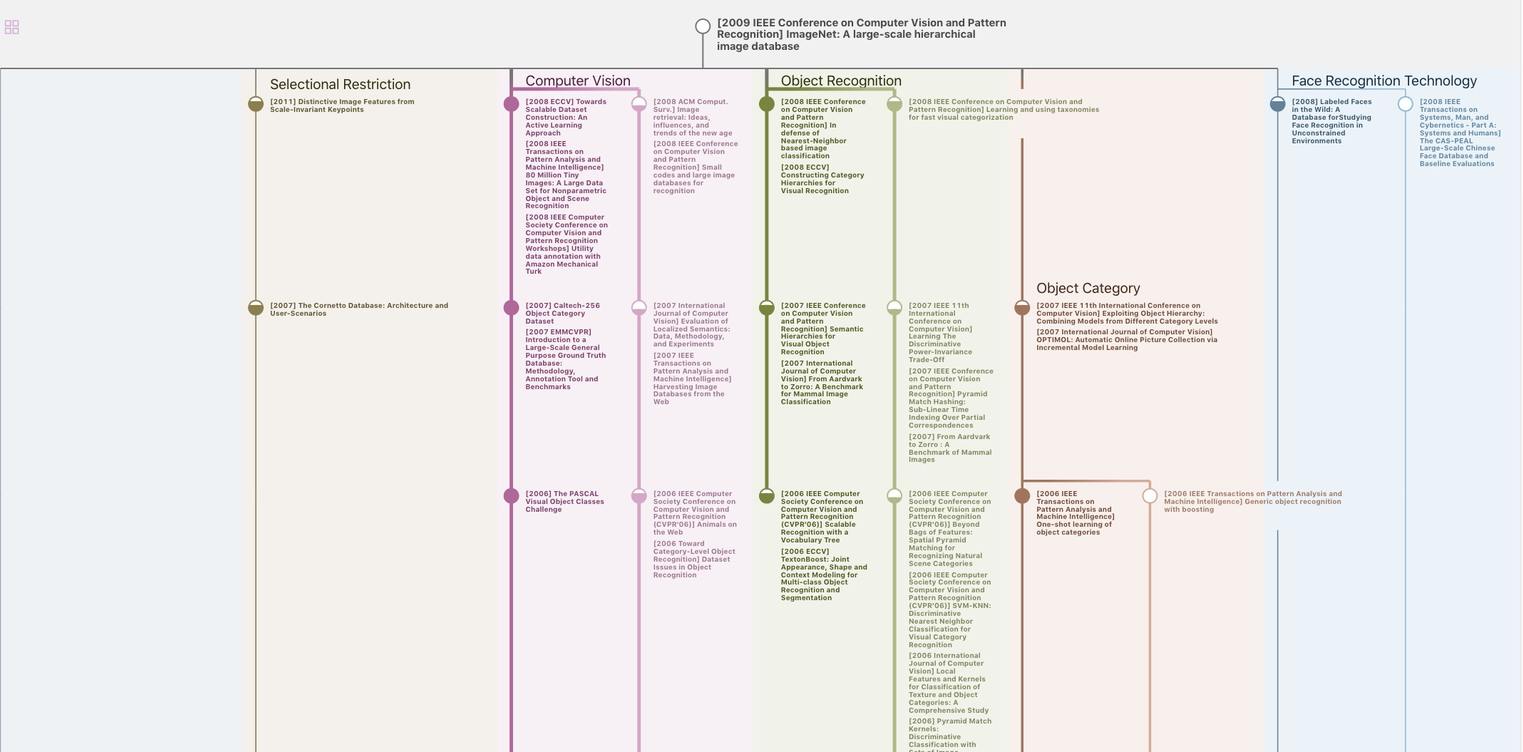
生成溯源树,研究论文发展脉络
Chat Paper
正在生成论文摘要