The Effect of Missing Data when Predicting Readmission in Heart Failure Patients.
2023 Computing in Cardiology (CinC)(2023)
摘要
Background: The discharge of patients from hospital care is regulated by guidelines. Still, readmission of heart failure (HF) patients is a common issue, and several calculators have been published to predict it. Aims: We elaborate on how the prediction performance decreases when features become missing. We also elaborate on which features should a user include every time to reach acceptable prediction performance. Method: We prepared a balanced dataset from HF patients in the MIMIC-III database
$(N=2,204)$
with 16 features. Using training data (80%) in a four-fold cross-validation manner, we evaluated all feature combinations
$(N=Z^{16}-1)$
and found the optimal feature set for the logistic regression model. We also evaluated feature presence in top-performing models (N=655) and identified mandatory features. Finally, we trained the resultant model using all training data and evaluated the effect of missing features (
$N=2^{8}$
combinations) using separate test data (20%). Results: We identified three mandatory features (age, blood urea nitrogen, and systolic blood pressure) and eight optional. This led to a resultant model with eleven features. The hazard ratio (HR) using test data showed a value of 2.08 (95%CI 1.66-2.61) when all eleven features were present. It also showed an HR of 1.73 (95%CI1.39-2.17) when only three mandatory features were present, and others were missing (i.e., replaced by zeros).
更多查看译文
AI 理解论文
溯源树
样例
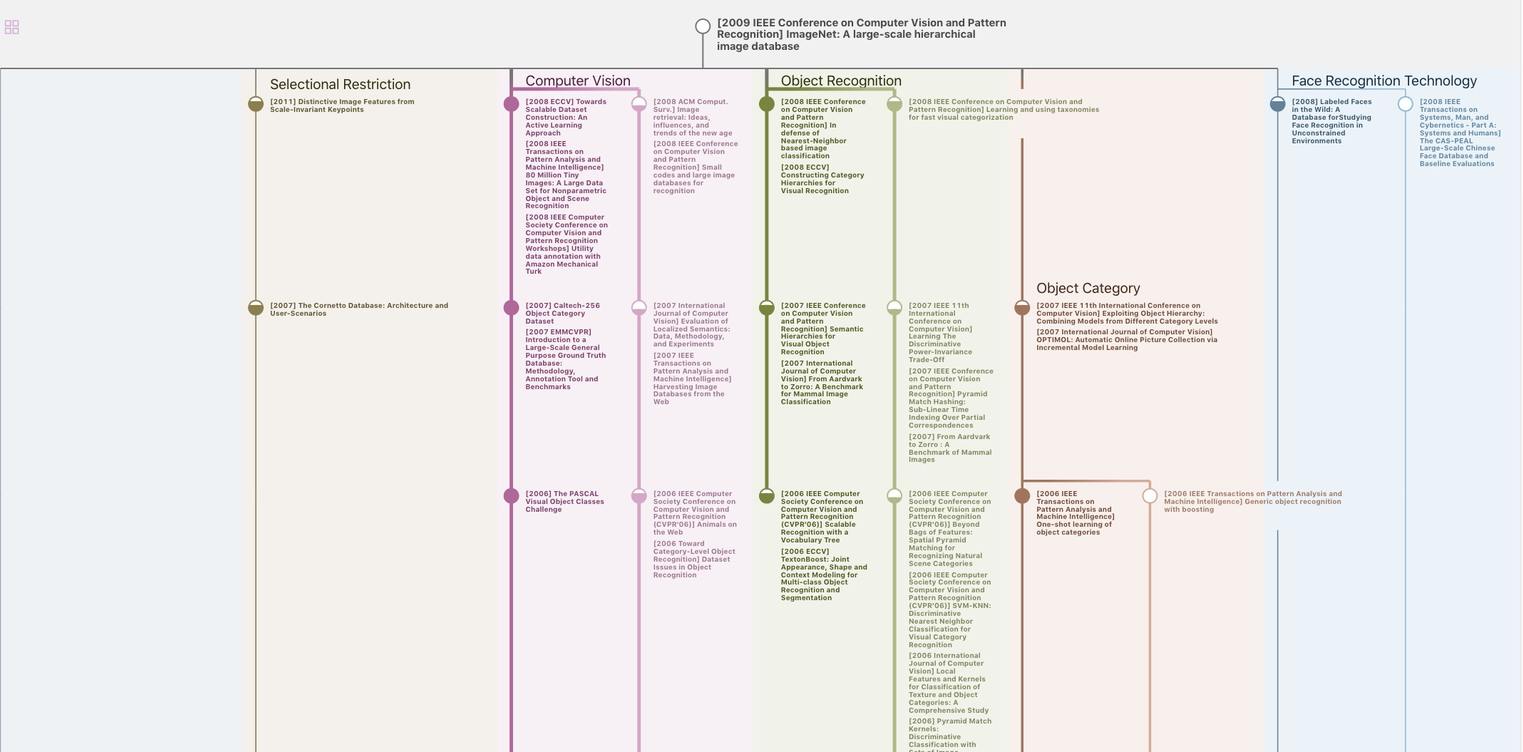
生成溯源树,研究论文发展脉络
Chat Paper
正在生成论文摘要