Estimation of Quiet Sleep in Preterm and Full-Term Newborns Using Machine Learning Algorithms Based on Cardio-Respiratory and Motion Signals.
2023 Computing in Cardiology (CinC)(2023)
摘要
Monitoring sleep states of newborns, especially those born prematurely, before 37 weeks of gestation, is essential for tracking their development. This study presents an automated method for estimating the state of Quiet Sleep (QS). Given that QS is characterized by regular cardiorespiratory rhythm and non-movement, this approach combines machine learning algorithms trained on cardiorespiratory features with body motion segmentation. It was evaluated on manually annotated recordings from 10 preterm and 5 full-term newborns. Each newborn was recorded for eight hours during their first week of life, and preterm newborns were recorded again at 37 weeks Post-Menstrual Age (PMA). The results achieved an average balanced accuracy of 78% and a Cohen's kappa of 0.51 across all recordings. For neonates with a PMA greater than 33 weeks, these values increased to 82% and 0.58, respectively. This approach proves effective and holds promise for continuously monitoring QS in newborns with a PMA greater than 33 weeks using non-invasive signals.
更多查看译文
AI 理解论文
溯源树
样例
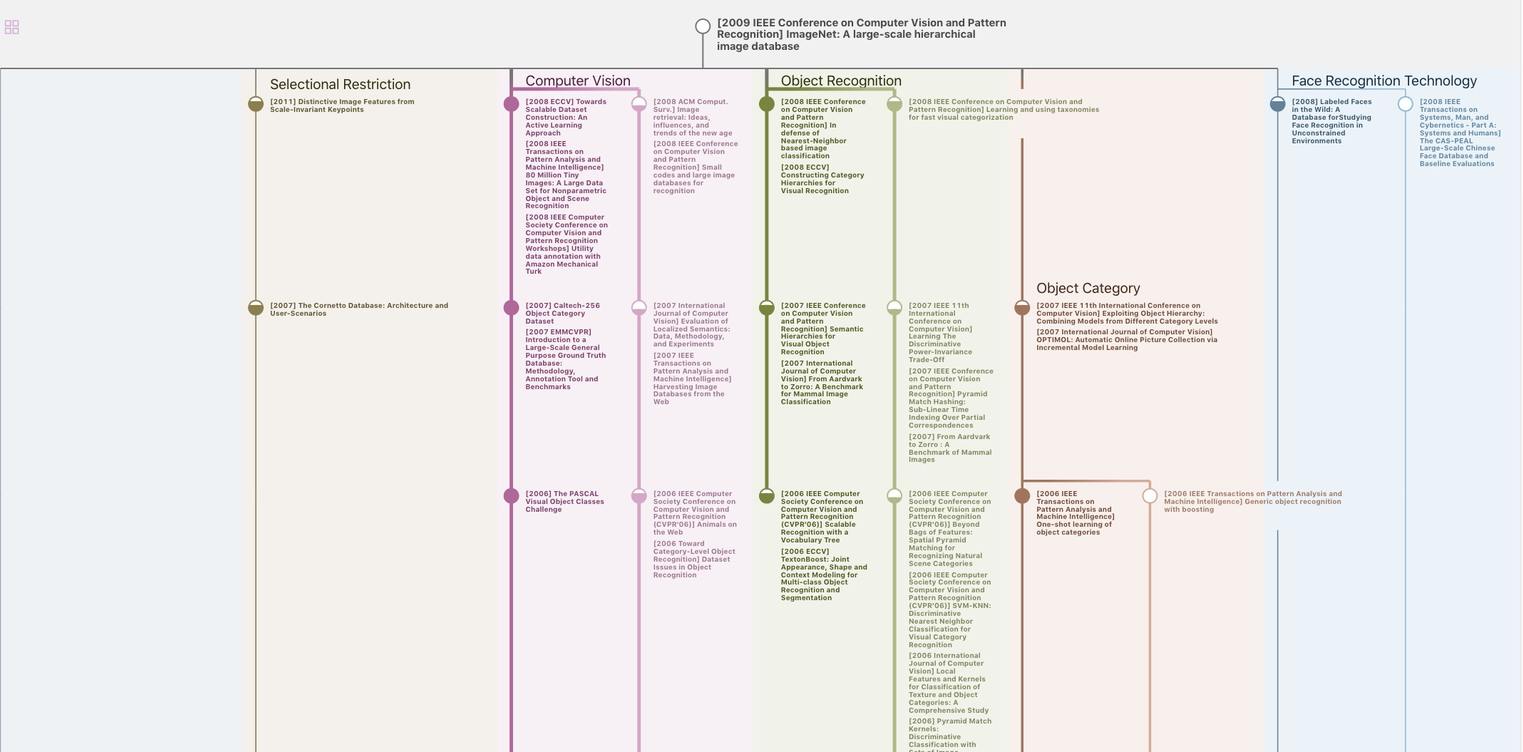
生成溯源树,研究论文发展脉络
Chat Paper
正在生成论文摘要