An Explainable AI Predictor to Improve Clinical Prognosis for Acute Respiratory Distress Syndrome.
2023 Computing in Cardiology (CinC)(2023)
摘要
Acute Respiratory Distress Syndrome (ARDS) is a severe respiratory disorder characterized by the failure of the lungs and often associated with elevated death rates. Providing an accurate clinical prognosis for ARDS patients is complex due to the myriad clinical variables involved. In this study, we introduce an Explainable AI Predictor designed to improve the accuracy of prognostic predictions for ARDS. -This work outlines a solution to the challenge of short-term ARDS diagnosis, utilizing an algorithm that leverages a multi-feature fusion approach based on XGBoost learning and Optuna. The proposed algorithm enables ARDS status prediction within a critical 48-hour window, which is vitally important for life-saving interventions in clinical settings. Furthermore, ‐‐ the proposed algorithm incorporates features that enhance interpretability, assisting medical professionals in diagnosis and treatment planning. Experimental results substantiate the efficacy of our proposed method, with the algorithm achieving an overall micro-AUC of 0.832 when applied to the test set. This performance metric underscores the accuracy and predictive strength of our approach. The encouraging results emphasize the algorithm's potential to facilitate healthcare professionals in making timely and precise decisions in managing ARDS.
更多查看译文
AI 理解论文
溯源树
样例
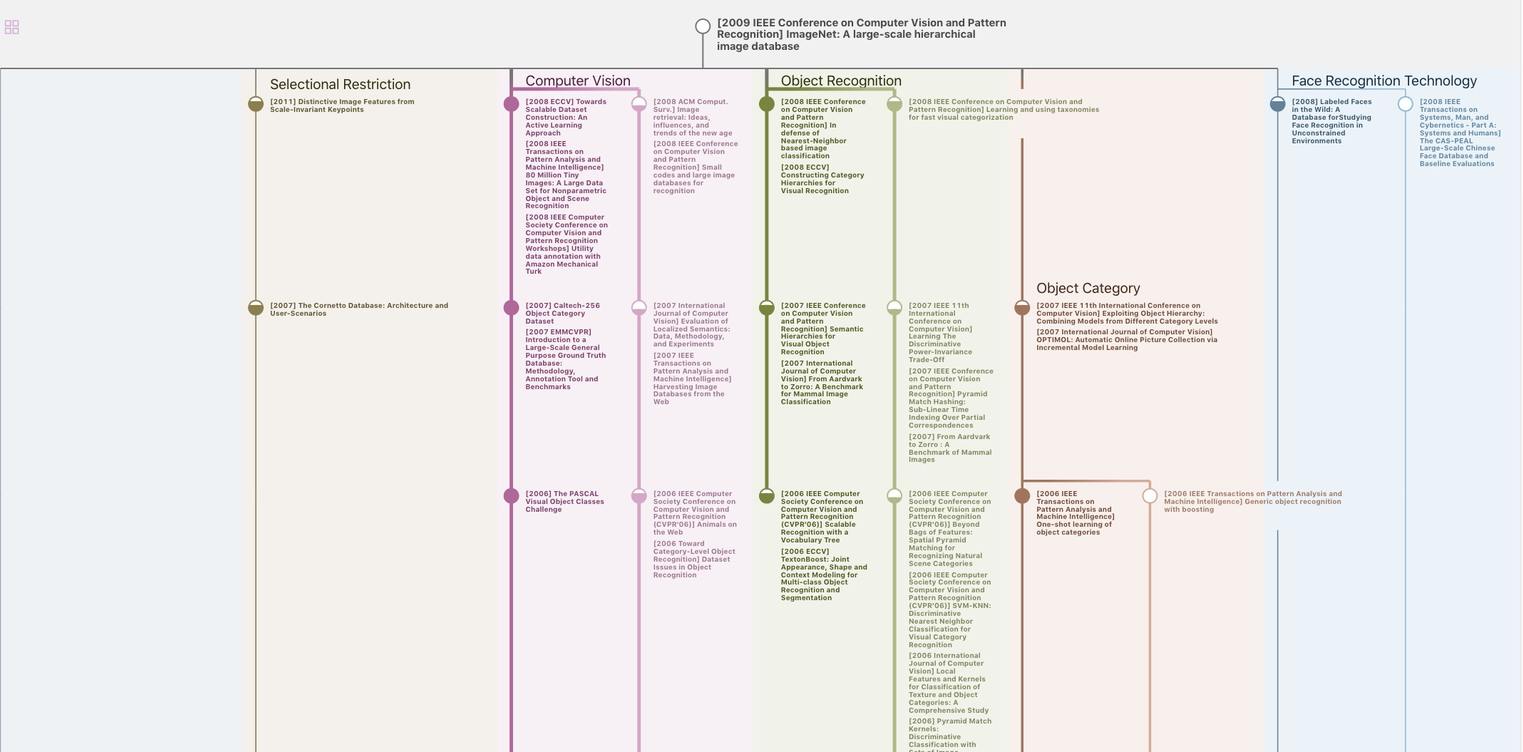
生成溯源树,研究论文发展脉络
Chat Paper
正在生成论文摘要