Patients Clustering on BPJS Health Insurance Data Using Partition Clustering Algorithm
2023 International Conference of Computer Science and Information Technology (ICOSNIKOM)(2023)
摘要
Patient Clustering is a grouping of patients who receive health insurance benefits that later can be used to identify, analyze, and predict the segmentation characteristics of patients with the health services they receive. The challenges for clustering in health insurance data are difficulties in understanding patient patterns and complex data. One of the complex health insurance data is BPJS (the National Health Insurance program in Indonesia) data. This study proposed a solution for clustering complexity of the BPJS data, which has mixed data types, by applying some blends in the data preparation phase involving data transformation strategies (standardization, one hot encoding, and binning) and data reduction techniques. The combination is also applied to optimize clustering performance. We experimented with Principal Component Analysis (PCA), Multiple Correspondence Analysis (MCA), and Autoencoder to simplify and reduce features without eliminating critical information so the result still produces meaningful information. Finally, the KMeans clustering algorithm is used to group BPJS patients. The result shows that the reduced dimension significantly affects the cluster structure; with the best approach, the MCA method combined with binning transformation produces clusters with a silhouette coefficient value of 0.66 (increased by more than 50% compared with the scenario without the dimension reduction technique).
更多查看译文
关键词
Patients Clustering,Health Insurance Data Clustering,BPJS Data Clustering (key words)
AI 理解论文
溯源树
样例
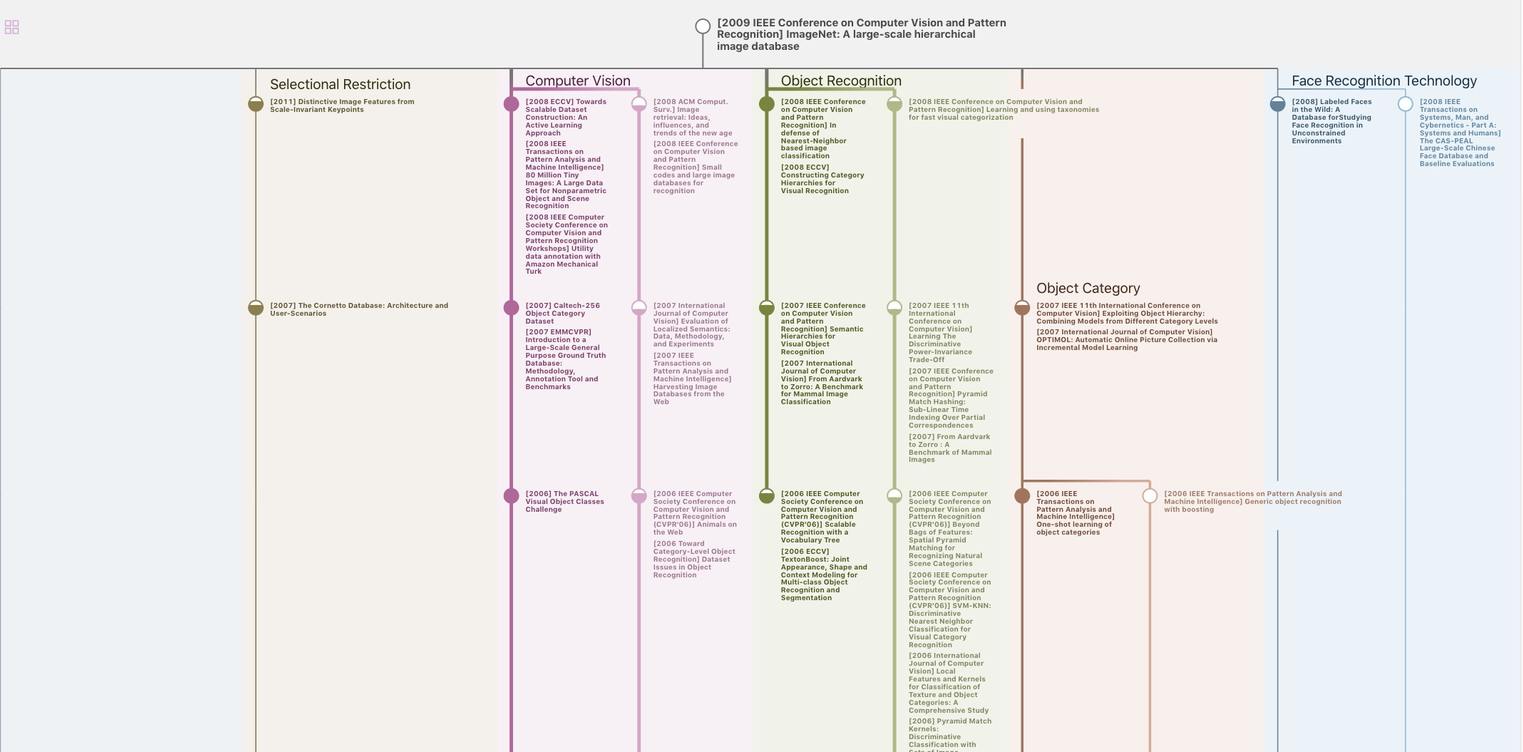
生成溯源树,研究论文发展脉络
Chat Paper
正在生成论文摘要