Decoding Performance Testing Results: Empowering Trust with Explainable Artificial Intelligence (XAI)
NAECON 2023 - IEEE National Aerospace and Electronics Conference(2023)
摘要
The paper advocates utilizing Explainable Artificial Intelligence (XAI) to enhance the trustworthiness of both black-box and interpretable models in the context of performance testing. The proposed methodology involves employing the Shapley Additive exPlanation (SHAP) algorithm as a surrogate model to aid performance analysts in comprehending the decision-making process of black-box machine learning models. By incorporating SHAP around black-box models, analysts can gain insights into the factors influencing the models' pass-or-fail predictions and understand the relative importance of performance data. To validate the effectiveness of the approach, extensive load testing experiments were conducted on a real-world testbed, incorporating industry-standard benchmarks and manual injection of performance bugs. The results demonstrate that the proposed approach significantly improves the trustworthiness of machine learning models by offering explanatory capabilities for their decision-making. Furthermore, the approach can be applied across various domains and requires minimal effort to operate, thus showcasing its generalizability and practicality.
更多查看译文
关键词
XAI,Performance Testing,Load Test,Random Forest,Artificial Neural Networks
AI 理解论文
溯源树
样例
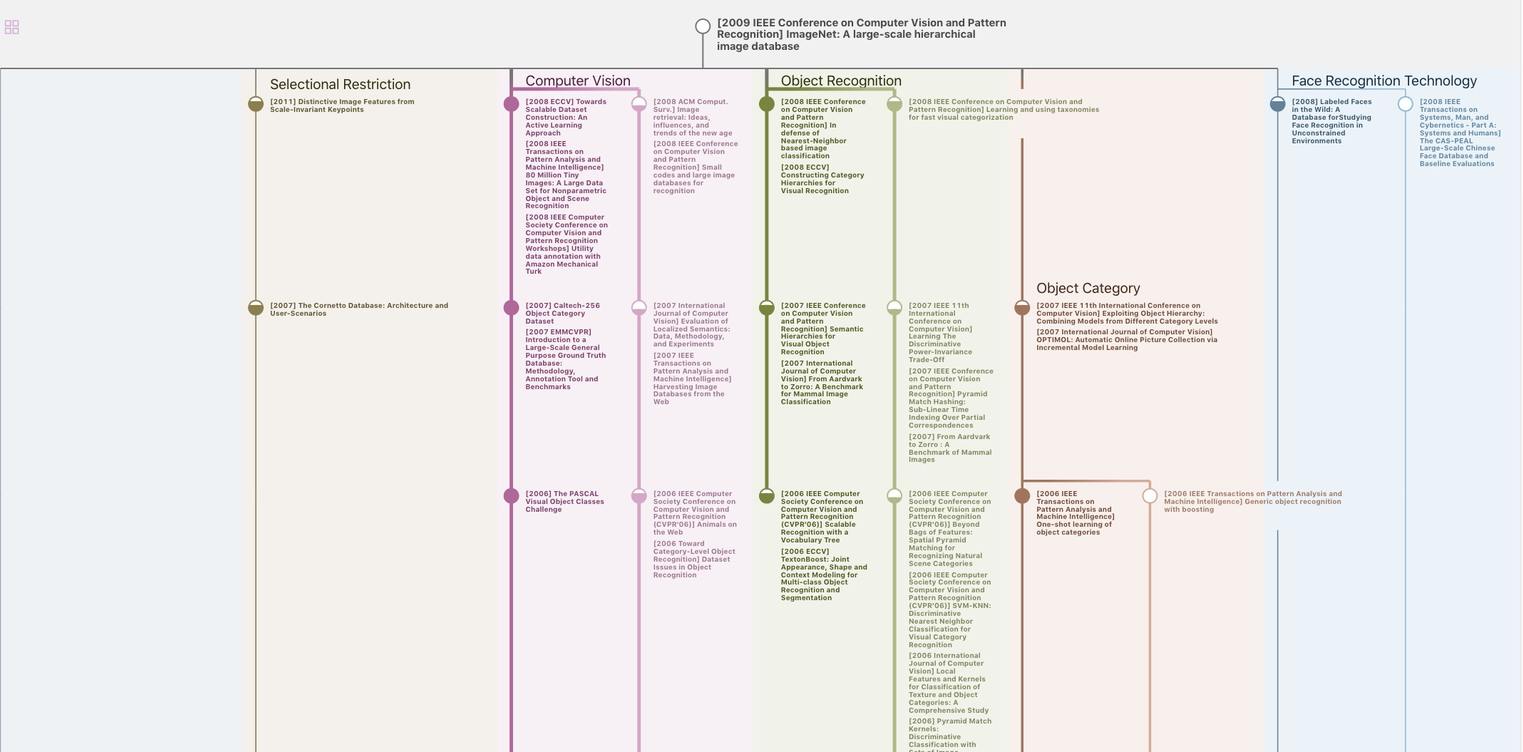
生成溯源树,研究论文发展脉络
Chat Paper
正在生成论文摘要