Machine Learning experiments for design purposes in air-traffic conflict-detection tool
Transportation Research Procedia(2023)
摘要
Machine learning (ML) is the ability of computers to learn mathematical relations to make predictions based on historical data. In recent years, ML has evolved rapidly and has become one of the most promising predictors. This work is aimed at providing insights into different experiments needed to optimize the design and performance of Conflict Detection (CD) tools based on ML algorithms. CD is the process of identifying pairs of aircraft that are likely to violate separation minima. Until now, CD predictors have been based on mathematical technology, i.e., the standard CD procedure is to predict aircraft trajectories and then analyze them to identify separation infringements. The approach presented here advances CD procedure by directly predicting separation infringement without the need to predict trajectory. This data-driven approach takes into account the ADS-B data broadcast by aircraft and the relative variables calculated between aircraft pairs. The algorithm's output is a prediction of the distance at the nearest axis. The results show insights into various possible solutions for building CD modules: depending on the aircraft pair's convergence or divergence, the aircraft pair considers reducing proximity distances, and it is evaluated if one predictor based on 3D separation provides better results than the combination of horizontal and vertical independent predictors. The goal is to analyze different operational solutions and identify the best solution to optimize CD tool performance.
更多查看译文
关键词
Air Traffic Control,Conflict Detection,Machine Learning
AI 理解论文
溯源树
样例
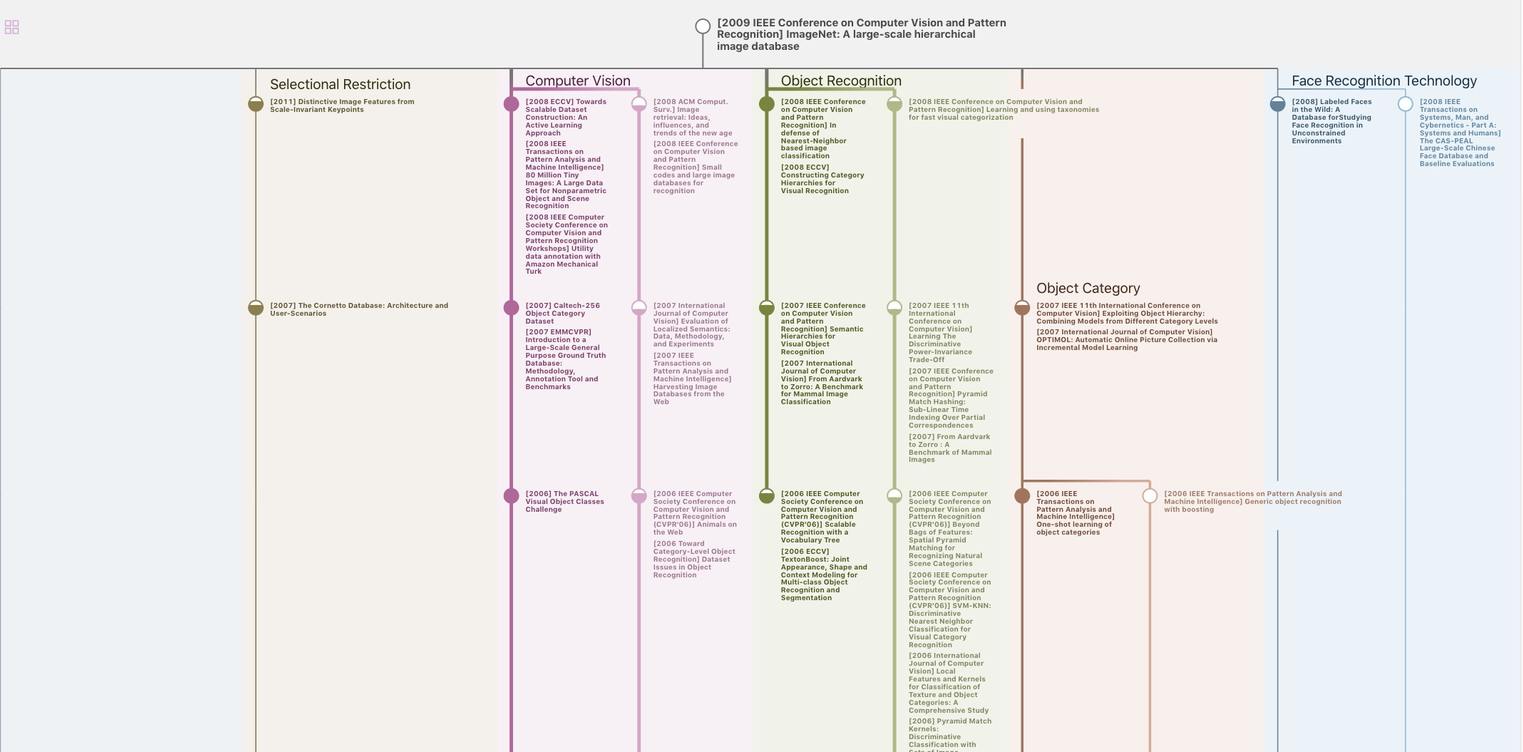
生成溯源树,研究论文发展脉络
Chat Paper
正在生成论文摘要