Machine learning framework for the real-time reconstruction of regional 4D ocean temperature fields from historical reanalysis data and real-time satellite and buoy surface measurements
PHYSICA D-NONLINEAR PHENOMENA(2024)
摘要
The ability to model 4D properties of near-coast ocean regions in real time is valuable for both general environmental monitoring and for specialized industry applications. We present a computationally parsimonious framework to build improved spatiotemporal models that combine dynamics inferred from historical high-fidelity model outputs and real-time sensor measurements. We are motivated by a temperature data set of the Massachusetts Bay from sensors that can only obtain measurements at the ocean's surface. We first apply standard principal component analysis (PCA) to a historical reanalysis data set of the time-evolving 3D (x, y, z) temperature field. Next, we train a temporal convolutional neural network (TCN) to predict the time coefficients of the vertical PCA modes, and their variance, as a function of surface temperature. Simultaneously, we estimate the time-evolving 2D (x, y) surface temperature field from the satellite and buoy measurements with multi-fidelity Gaussian process regression (GPR). Finally, the surface temperature is used as input to the neural network to probabilistically predict the PCA coefficients and reconstruct the full 4D temperature field. The results are compared to in-situ measurements at all depths, and the median absolute error is found to be 0.97 degrees C. Overall, the proposed framework is able to produce more accurate models in less time, and the uncertainty quantification can be leveraged for future decision-making.
更多查看译文
关键词
Ocean temperature,Temporal convolutional network,Uncertainty quantification
AI 理解论文
溯源树
样例
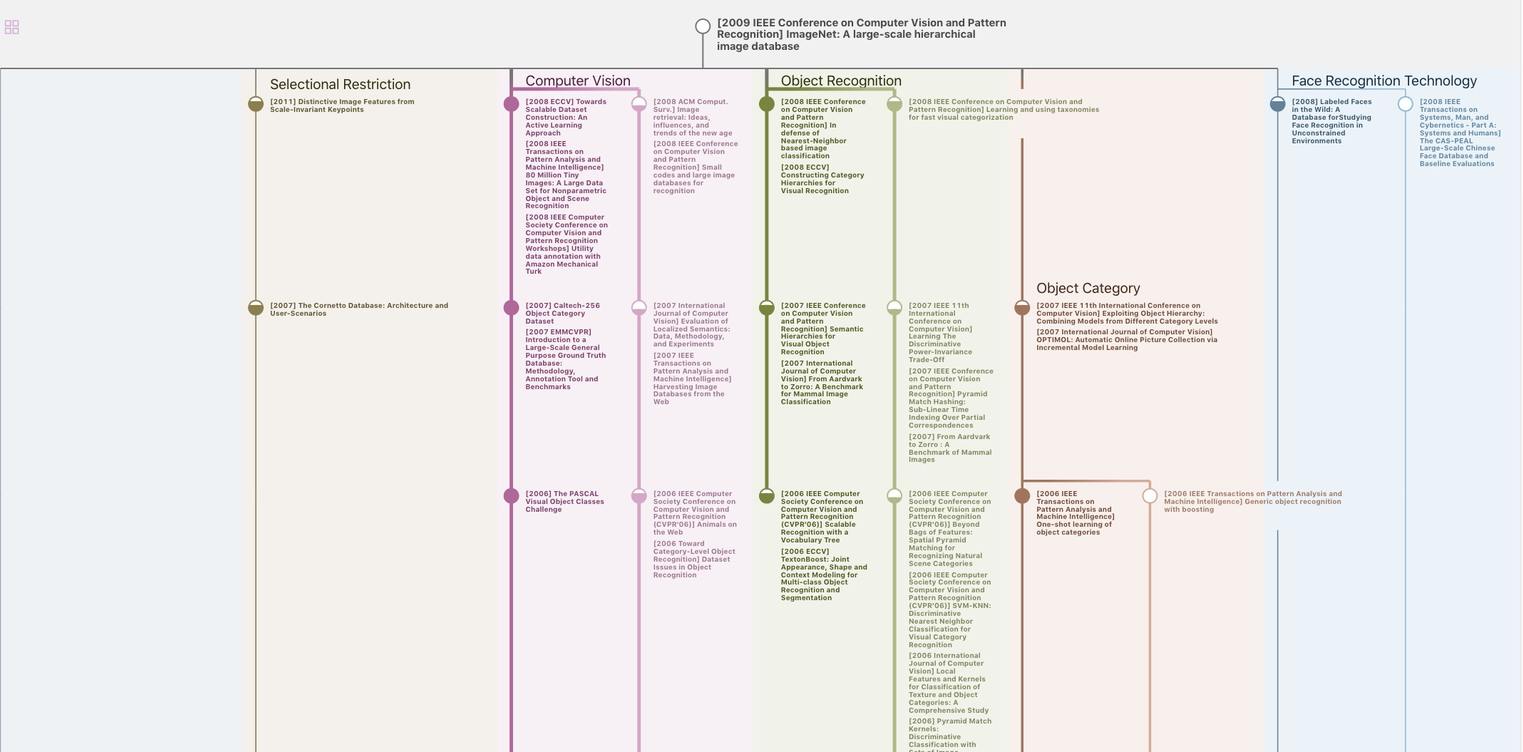
生成溯源树,研究论文发展脉络
Chat Paper
正在生成论文摘要