Spatial-temporal traffic data imputation based on dynamic multi-level generative adversarial networks for urban governance
APPLIED SOFT COMPUTING(2024)
摘要
Complete and accurate traffic data lays an essential foundation for urban governance and intelligent transportation system. Data missing is an inevitable issue in road network analysis with the expansion of urban roads and increasing application of data monitors. Data imputation is regarded as a cost-effective approach for solving data missing problem. However, the spatial-temporal features of massive traffic data have not been comprehensively considered in the previous related studies, and non-uniform distribution of missing data often causes poor results. Therefore, we propose a dynamic multi-level generative adversarial networks (MLGAN) model for the imputation of large-scale spatial-temporal traffic data. The model first captures the spatial-temporal features of traffic data through composite features extractor based on the bidirectional gated recurrent unit (BGRU) and the graph convolutional network (GCN) structure. Then, different sampler structures with varied missing rate are adopted to analyze the feature data and present the reconstruction results. Enhanced by the dynamic stratification strategy, the model is less sensitive to the variation of missing rates. Finally, data imputation experiments are conducted to validate the proposed approach using the data collected from the PeMS dataset. The results show that the proposed model has superior data imputation performance on large-scale dataset compared with the traditional models.
更多查看译文
关键词
Urban governance,Traffic data imputation,Dynamic multi -level generator,Generative adversarial networks,Deep learning
AI 理解论文
溯源树
样例
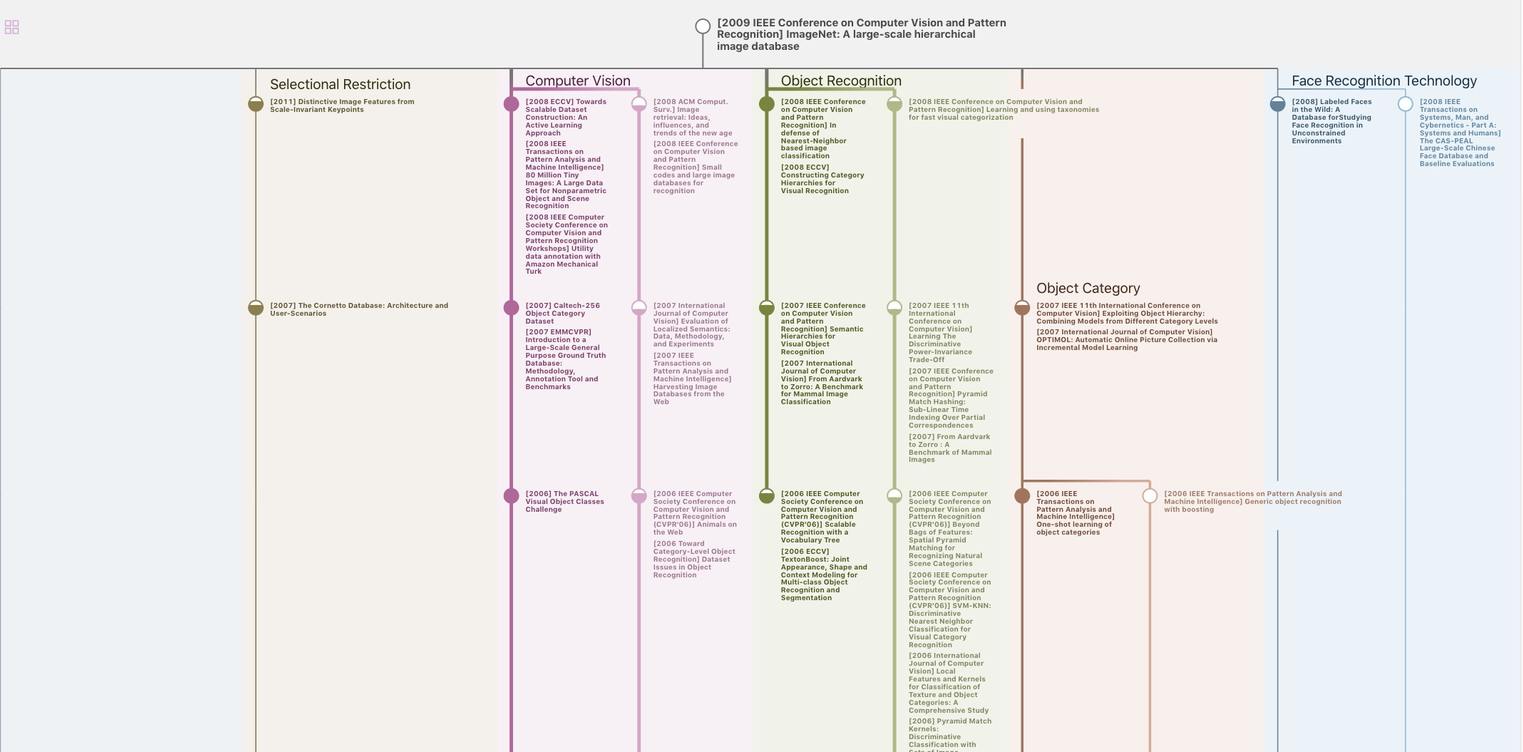
生成溯源树,研究论文发展脉络
Chat Paper
正在生成论文摘要