Calibrating low-cost sensors using MERRA-2 reconstructed PM2.5 mass concentration as a proxy
ATMOSPHERIC POLLUTION RESEARCH(2024)
摘要
Monitoring and mitigating air pollution is the most urgent need of society. In an ideal scenario, air quality must be mapped to the details of the geospatial grid to formulate area-specific roadmaps to mitigate air pollution. However, the heterogeneity of the existing air quality monitoring network in India must be addressed to improve the granularity of the data. Low-cost sensors (LCS) are a potential solution to overcome these challenges. However, their data quality remains an issue. Here, we present an alternative Machine Learning (ML)-based calibration approach for LCS using Modern-Era Retrospective Analysis for Research and Applications, version 2 (or MERRA-2) reconstructed surface-level PM2.5 data as a proxy to the reference mass concentrations at four monitoring sites in NCT-Delhi. LCS were calibrated with the co-located reference and MERRA-2 mass concentrations across sites, and their performance was evaluated. The reference and MERRA-2 reconstructed PM2.5 mass concentrations indicated a better agreement compared to the co-located reference and raw LCS measurements. The R2 values between the MERRA-2 calibrated LCS and reference mass measurements were 0.76 (R2LCS_uncalibrated = 0.68), 0.84 (R2LCS_uncalibrated = 0.76), 0.75 (R2LCS_uncalibrated = 0.65), and 0.78 (R2LCS_uncalibrated = 0.69) at sites S1, S2, S3, and S4, respectively. RMSE values reduced significantly post-calibration across sites. LCS calibrated with the reference monitors produced comparable results to MERRA-2 calibrated LCS across sites, which suggests that the calibration of LCS with MERRA-2 reconstructed PM2.5 is feasible. This new method of LCS calibration will promote citizen-science initiatives and allow regulators to gather high spatiotemporal air quality information.
更多查看译文
关键词
Low-cost sensor,MERRA-2,Calibration,Machine learning,PM2.5
AI 理解论文
溯源树
样例
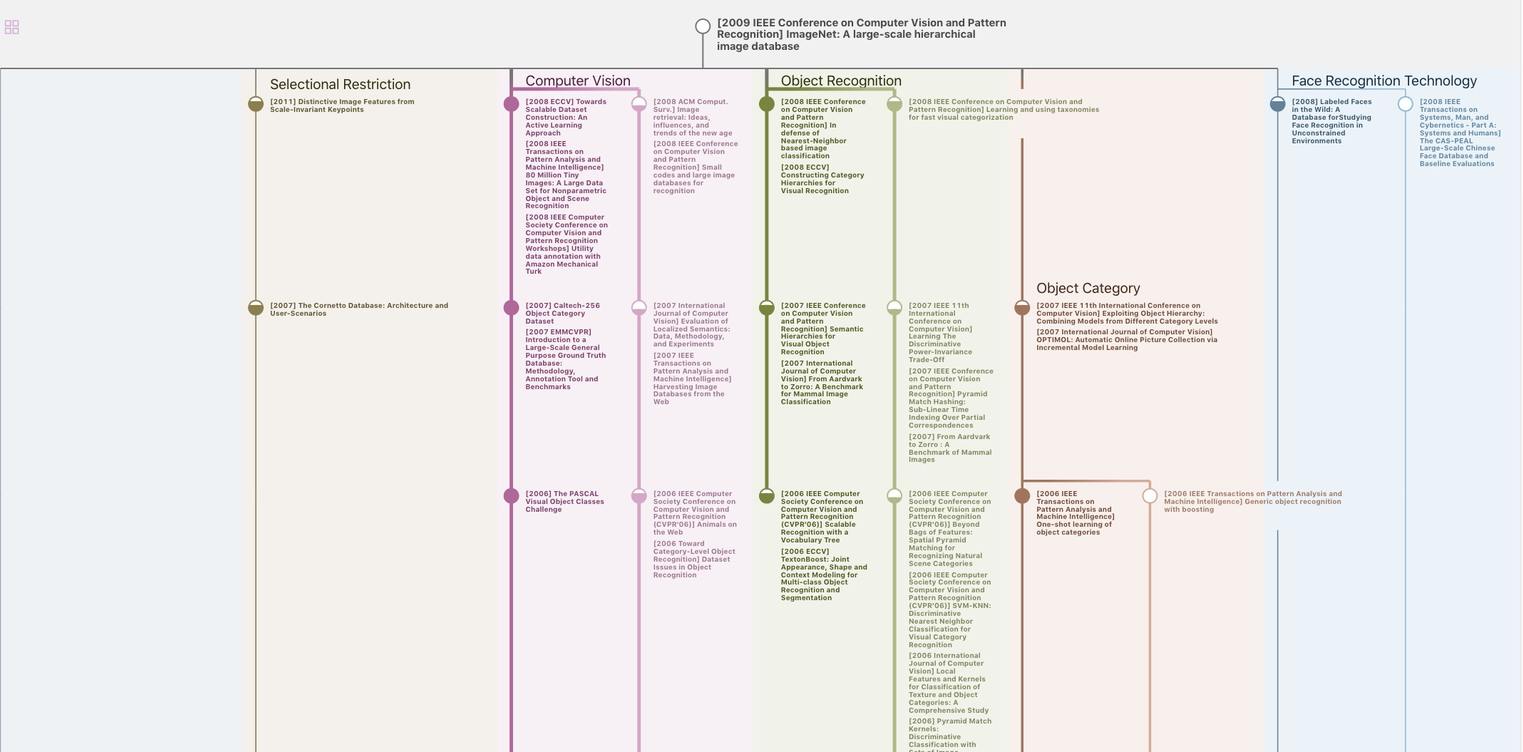
生成溯源树,研究论文发展脉络
Chat Paper
正在生成论文摘要