A multi-type semantic interaction and enhancement method for tax question understanding
ENGINEERING APPLICATIONS OF ARTIFICIAL INTELLIGENCE(2024)
摘要
Problem classification serves as a fundamental process in a tax intelligence consulting system, enabling the categorization of user-posed questions according to their semantic attributes. This categorization is pivotal in ensuring accurate question comprehension. Nevertheless, the inclusion of intricate professional terminology and the frequent alterations in linguistic structures associated with tax-related matters may culminate in suboptimal classification outcomes and hinder the precise comprehension of user demands. To address these issues, we propose a multitype semantic interaction and enhancement method (MtSIEM) to classify tax related issues that integrates entity and nonentity semantics to represent the semantic features of tax-related domain issues. Specifically, a pretraining language model and multigram mechanism are adopted to enhance the feature extraction ability. A soft attention module is also simplified to allocate interaction information weights, thereby adaptively determining the importance of the feature elements. These three components are used to perform precise learning on the tax question data. Subsequently, a dynamic routing architecture is employed to capture the relationships between the different problem features, resulting in predictive vectors. A series of comparative experiments on tax question data demonstrated that the proposed model achieved a classification accuracy of approximately 94.46%, an improvement of 2.99% compared with the baseline. Therefore, the proposed model can be utilized to predict the semantic category of tax-related issues, assisting intelligent tax advisory systems in matching questions with the most relevant knowledge and professional domains, thereby enabling faster retrieval of pertinent information and enhancing the timeliness of responses.
更多查看译文
关键词
Question classification,Pretraining language model,Deep semantic,Transformer
AI 理解论文
溯源树
样例
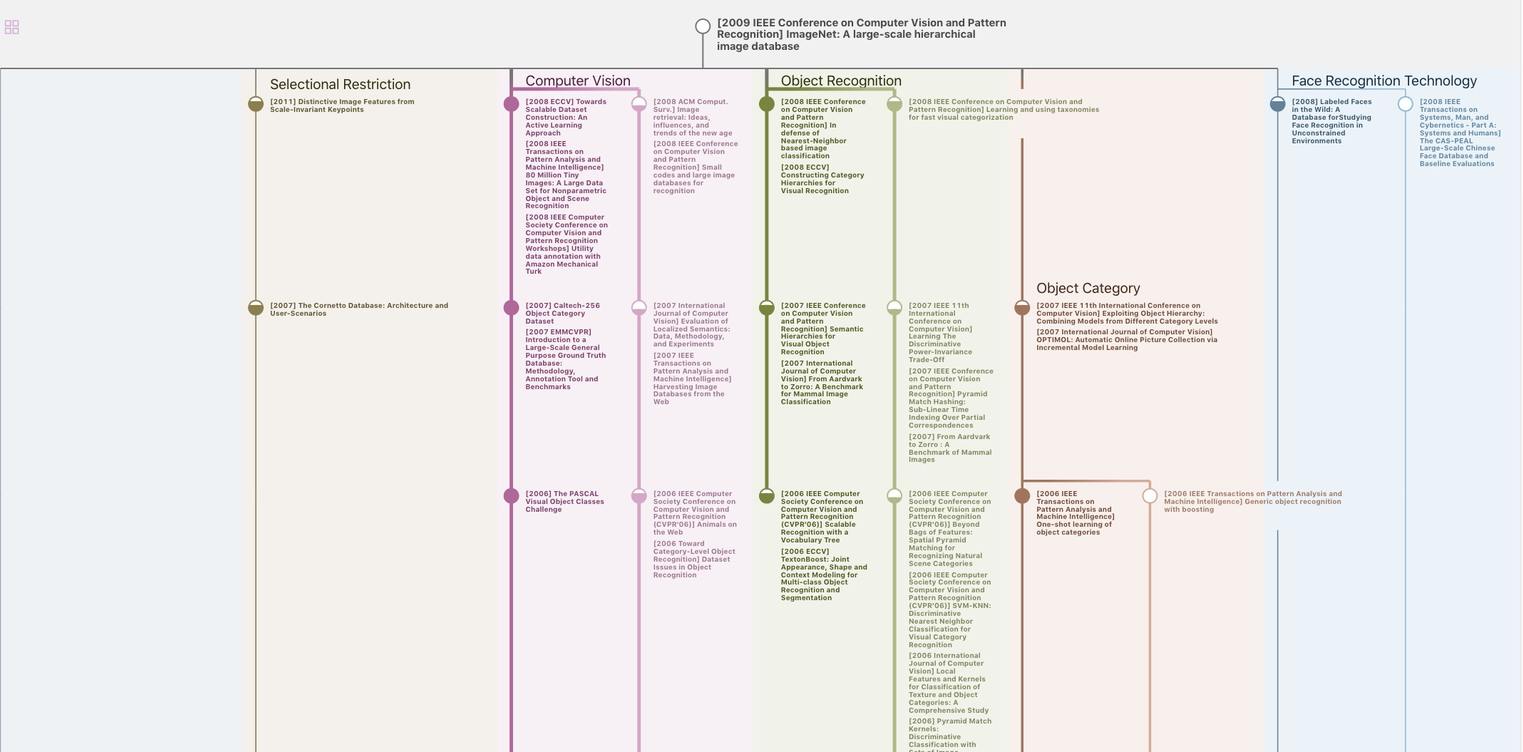
生成溯源树,研究论文发展脉络
Chat Paper
正在生成论文摘要